A Meta Analysis
Free-to-use models merit more scrutiny, not less.
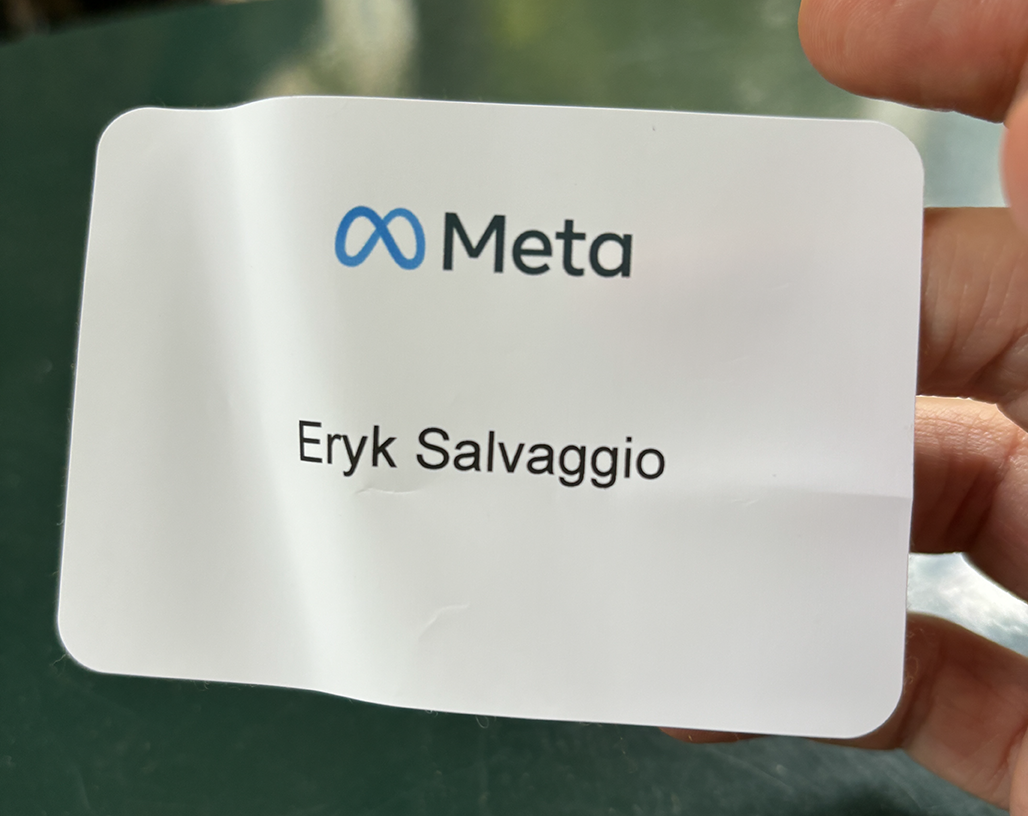
Last week I was invited to a small gathering of folks from the Middle East, Turkey, and Africa that Meta had convened to discuss the ethics of open-source AI with Yann LeCun, Facebook’s vice president and chief AI scientist, in conjunction with the UN General Assembly. I should say that Meta didn’t pay me to be there — I was in NYC for another event anyway, in my role with the Siegel Family Endowment for the Tech Together Conference focused on Public Interest Technology.
Facebook’s LLaMA is an open(ish)- source model for text generation. Some important caveats apply. For example, we don’t know what’s in the training data — that’s not accessible, and Meta uses those magic words, “publicly available data,” to let us know that it’s probably stuff you don’t want them to have. There are limits on its free use, though that only kicks in if you have 700 million or more users. For many, LLaMA is to language models what Stable Diffusion was to image models: a starting point for building new interfaces and tools.
Meta positions LLaMA as the foundation of an AI infrastructure stack. You can build on top of it, fine-tune it to new outcomes, localize them — for example, make them more culturally appropriate, or create new language versions altogether. By providing the model for free, people don’t go to Meta’s competitors. This free, distributed model has enormous advantages for anyone building an AI ecosystem.
I was in a room with several representatives from startups, nonprofits, academia, and policy from across Africa, the Middle East, and Turkey. I did not say much. They all saw LLaMA as a starting point for cultivating an AI ecosystem in their regions.
I need to position myself a bit here. While I’m grateful to have been in that room, I can only lay out the conversation as I heard it and offer my thoughts informed by scholars I respect from the region, which I will cite below (many of which come from DAIR). I am not even sure it is my conversation to make sense of, nor, I should emphasize, does it represent even a shred of the population that those 15 or so folks in the room represented.
The consensus was that the infrastructure for AI consists of three things: models, computer power to run those models, and people, though primarily this meant engineers to deploy and maintain the models. People might also apply to people subjected to these models, but we didn’t discuss that much. They also agreed that this infrastructure should lead to the development of tools from the regions where such tools would be deployed. Tools built with LLMs ought to understand local contexts and languages — which I respect — but there was little clarity, or even discussion, around what those tools might be, aside from being LLMs themselves.
Much of the discussion was about securing a future for developing these models in the places they would be used — rather than relying on Europe, China, or the US to supply models that needed adaptations. Open(ish) models certainly can make that possible. The concerns that emerged in that room were linked to GPU access. The models are there, but what then? Without access to GPUs and scholarships for engineers, the local infrastructure doesn’t advance.
LLMDs
Everyone agreed that more resources were necessary, even if nobody could say why. This isn’t rare—I often meet people or leave meetings without knowing what purpose an LLM serves that justifies the resource investment or the compromise of data protection. We’re told the purpose is out there, in the latent space of technological possibility, and that we will find it through scaling. But with resources growing more scarce, the question is more fraught.
In one striking example, an attendee mentioned the low rate of trained medical professionals in their country and how tools like Aloe (an LLM for healthcare, built on top of LLaMA 3) required more significant investments in local engineers to fine-tune the model for the regional context. Notably, Aloe is still controversial in its primary language. It’s challenging to see this as a better solution than funding medical schools or scholarships to train doctors and nurses.
There is a long-running trade-off around LLMs as being “better than nothing.” I remember, circa 2016, people were already discussing chatbot therapists for refugees. The idea was that many who struggled with mental health issues, particularly refugees with PTSD, could not access the resources of a licensed therapist. Instead, they could use a chatbot. This would provide admittedly substandard care to people deprived of standard care, and of course, this is an improvement over no help. There was a political expediency at play. Getting resources for refugees required a mobilization of political will. Building a chatbot required four engineers and a comparatively paltry investment, and what they built could be monetized (in other contexts) for a return on that investment. If it was bad, it could be improved by deploying early and often.
This always struck me as an uneasy compromise. Using vulnerable people as a test market for a mental health support system with unknown risks. That it was better than nothing, however, made me feel a sense of tremendous urgency in solving that problem, which led me to understand the compromises being made by the technologists, even if I disagreed. However, I still didn’t know why we had to build and scale a technology rather than scale the training of mental health professionals or other resources for vulnerable people. It was often pitched as an addition, but nobody ever talked about the core issue, which was that we needed more people who knew how to help people.
I worry that this way of thinking transforms the world's fundamental challenges into the logic of a product development cycle. At the same time, this was ostensibly a good use of technology, one driven by meaningful purpose.
If you believe in the myth of AI as a perpetual information machine, a problem we can solve to solve all future problems, then a different set of priorities emerges. But these priorities make increasingly less sense to those of us who don’t believe in that hypothetical idea engine.
On the other hand, if you think medical LLMs are risky, you may not want to see resources used to develop them. But you don’t have to believe LLMs are good doctors. You just have to think they are better than nothing. The problem is when “better than nothing” is mistaken for a reason to preserve the state of “nothing.”
Time after time, I have seen AI held up as a solution to issues that AI cannot solve, from climate change to traffic accidents to mental health crises. There are ways to tackle these problems other than waiting for AI to do it for us. But this was idealistic talk. People told me, in so many words, that we could all work to convince a reluctant world to train a fleet of care workers amidst vast evidence of our futility, or we could fund four guys to build a tech product. This was San Francisco. The answer was clear.
A quick note
Are you a regular reader of this newsletter? If so, please consider subscribing or upgrading to a paid subscription. This is an unfunded operation; every dollar helps convince me to keep it up. Thanks!
The Catch-22
The Catch-22 at the center of these conversations is defined by the Latent Space Myth. There is a “race” defined by words like “adoption” and the need to “advance” AI. This myth assumes that this advancement moves us closer to a goal—but what is that goal? In big tech mythos, the goal is the infinity machine, the perpetual engine. Whoever discovers the perpetual knowledge machine first will hold enormous power over those who are “left behind.”
You only need a sliver of doubt about these systems and the purposes they may someday serve to feel that the global majority should be included in finding that use. Exclude them until their use is found, and you end up exporting technologies built by China or California that are adapted for “the rest of the world” rather than developed by people who live where they are used. This repeats a pattern of colonization through infrastructure we have seen throughout history. Rich nations or their beneficiaries deploy infrastructure under extractivist terms and conditions, then build dependencies on that infrastructure with escalating costs whenever any wealth emerges.
I’m biased. Here are what my biases are, regarding what I perceive to be the real tensions at play:
- A desire to curtail (often necessary) dependencies on US (or European, or Chinese, or Elon Musk’s) corporate infrastructure from unfairly dominating local infrastructure.
- A desire to expand equitable access to the development of emerging technologies, reducing the risk of harm and widening the perspectives on social impact and deployment needed to build better, more equitable systems.
This particular emerging technology may be a bubble on the verge of a plateau or collapse rather than a breakthrough, making this tension more fraught. We are building dependencies on gen AI infrastructure everywhere, on a risky proposal. If it pays off, as Altman says — great! Infinite wealth! But if it falls short, the investment burden into this infrastructure is, as always, staggeringly disproportionate. That means a lot when the investment from within our leading economies is already reaching unsustainable levels.
If models are part of the AI infrastructure, and Meta’s infrastructure isn’t doing what it claims to be doing for other languages, then we’ve seen this all before.
My other bias is skepticism that LLMs will have such profound use cases. I believe that their main benefit will be content moderation, followed by mediating phone trees and replacing (poorly) human teachers with iPads. In that case, generative AI represents a tragic misallocation of energy, time, and resources at its current level. I am being reserved.
But what if you believe AI might still hold some small purpose — or even a tiny seed of a revolutionary purpose? What if you believe the myths of Silicon Valley?
In that case, the tragedy is that nations that are not in Europe or North America lose out on being the ones to find and shape whatever purpose is “out there” for LLMs to achieve.
We can all agree that exclusion from the development of technology leads to many well-documented problems. I recently re-read Avijit Ghosh’s findings that Stable Diffusion generates darker skin when prompted for “poorer” people in Indian contexts. From Asmelash Teka Hadgu, Paul Azunre, and Timnit Gebru at DAIR, we have research finding that Meta’s models, when tailored to Indigenous African languages, often scrape data from the Web that isn’t appropriate to the task of modeling that language and then underperform against their reported benchmarks.
Exclusion is a concern for abuse when Western companies are dictating or ignoring everyone else. Meanwhile, some forms of concern over abuse are also a tool of exclusion. For example, asking people what they will do with the tool, whether they’re “responsible enough” to manage it, flagging certain regions as being riskier, etc. I agree with Meta and many in that meeting that limiting access to compute and models based on “security fears” is racist pablum.
Many of the worst aspects of generative AI indeed arise whenever it is deployed onto people rather than developed with people — or even better, by the people who will use it. But I don’t know if Meta’s open model really fits those criteria.
Yes, Meta’s LLaMA may help accelerate the development of GAI applications in these regions, and for local developers and users, it may make sense. But what if those tools are anchored in myths and hype about capabilities, teetering on a collapse where any economic effects will be significantly more challenging for smaller economies investing in that infrastructure?
My past experience — and research from Nyalleng Moorosi, Raesetje Sefala, and Sasha Luccioni — points to other risks. GAI may also be used as an excuse to avoid investment and support for local development of local models, encouraging “adaptation” of Meta’s models that don’t work the way Meta claims they do. Pair this with the driving case being its use for health and medicine, and you will see how such scaffolding threatens to have catastrophic results for failure. We see this in all kinds of models: a vast sum of Western-biased training data modified by sparse local data. The larger dataset “haunts” the outcome of those models. It is always derived from the broader language of the LLM, and the embedded logic and cultural context remain strong.
It was suggested that “More language data (in any language) means more languages,” but I’m unconvinced. More language data also means more bias and weight towards the model's dominant language. Adapting a local model to Meta’s LLM through fine-tuning isn’t the best technical solution. It shifts local development of a more accurate local model to a dependency that has to be fine-tuned and calibrated by local users, with adverse effects simply accepted as they are rushed to deployment. A startup may need to depend on this and may find ways to make it work for them, but caveat emptor.
LLaMA and the strings attached to it merit the most vigorous critique, because it will permeate a broader range of uses. If models are part of the AI infrastructure, and Meta’s infrastructure isn’t doing what it claims to be doing for other languages, then we’ve seen this all before. It’s a result of building for but not with.
Many in the room would argue that indeed, Meta is building-with by offering its model for free. I see a disconnect there. The model wasn’t built with anybody.
Likewise, data protection rules benefit everyone. Suppose Meta uses LLaMA’s open-ishness to promote practices that harm the rights of creators, artists, and historians in these regions. Or, worse, they introduce flawed medical advice and deploy them among — or instead of — overworked care workers. In that case, it is scaffolding its AI infrastructure within broader social harms. The need to reduce those harms is even more significant because it is open — not above critique simply because open is “the right thing to do.”
I've mistyped if I’ve written anything that looks like I have answers. Instead, I just have a lot of concerns.
You got this on a Thursday because I am trying to write shorter, more frequent posts. This was part two of what was sent on Sunday, which you can read here.
Things I am Doing This Week
In Person: Unsound Festival, Kraków! (Oct. 2 & 3)
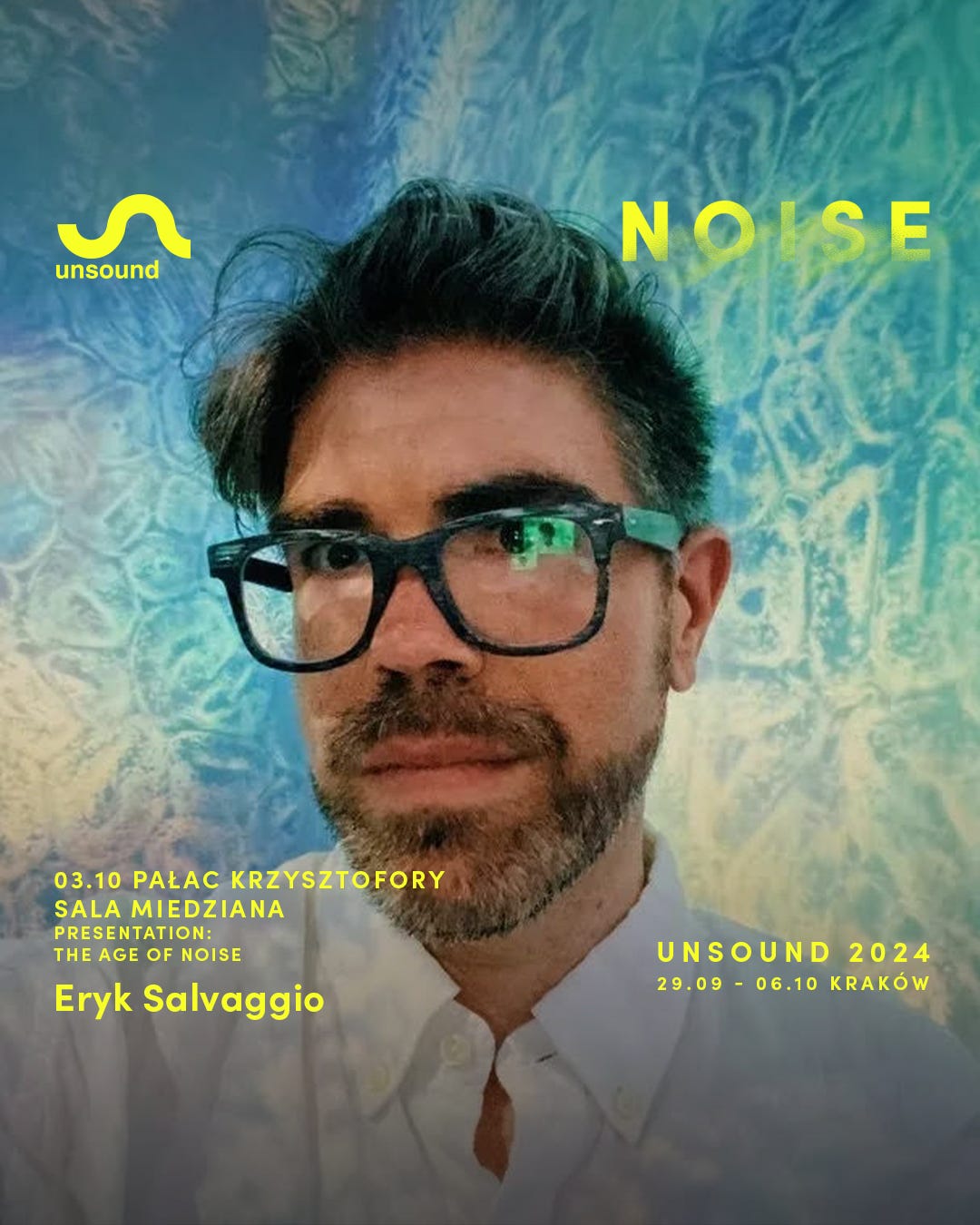
This week I’ll be in Kraków, Poland for the Unsound Festival! I was part of an experimental “panel discussion” on October 2 alongside 0xSalon’s Wassim Alsindi and Alessandro Longo, percussionist/composer Valentina Magaletti and Leyland Kirby aka The Caretaker.
Today (October 3 at 3pm!) I’ll present a live video-lecture-performance talk, “The Age of Noise.” Both events are at the Museum of Kraków. Join us! More info at unsound.pl.
Exhibition: Poetics of Prompting, Eindhoven!
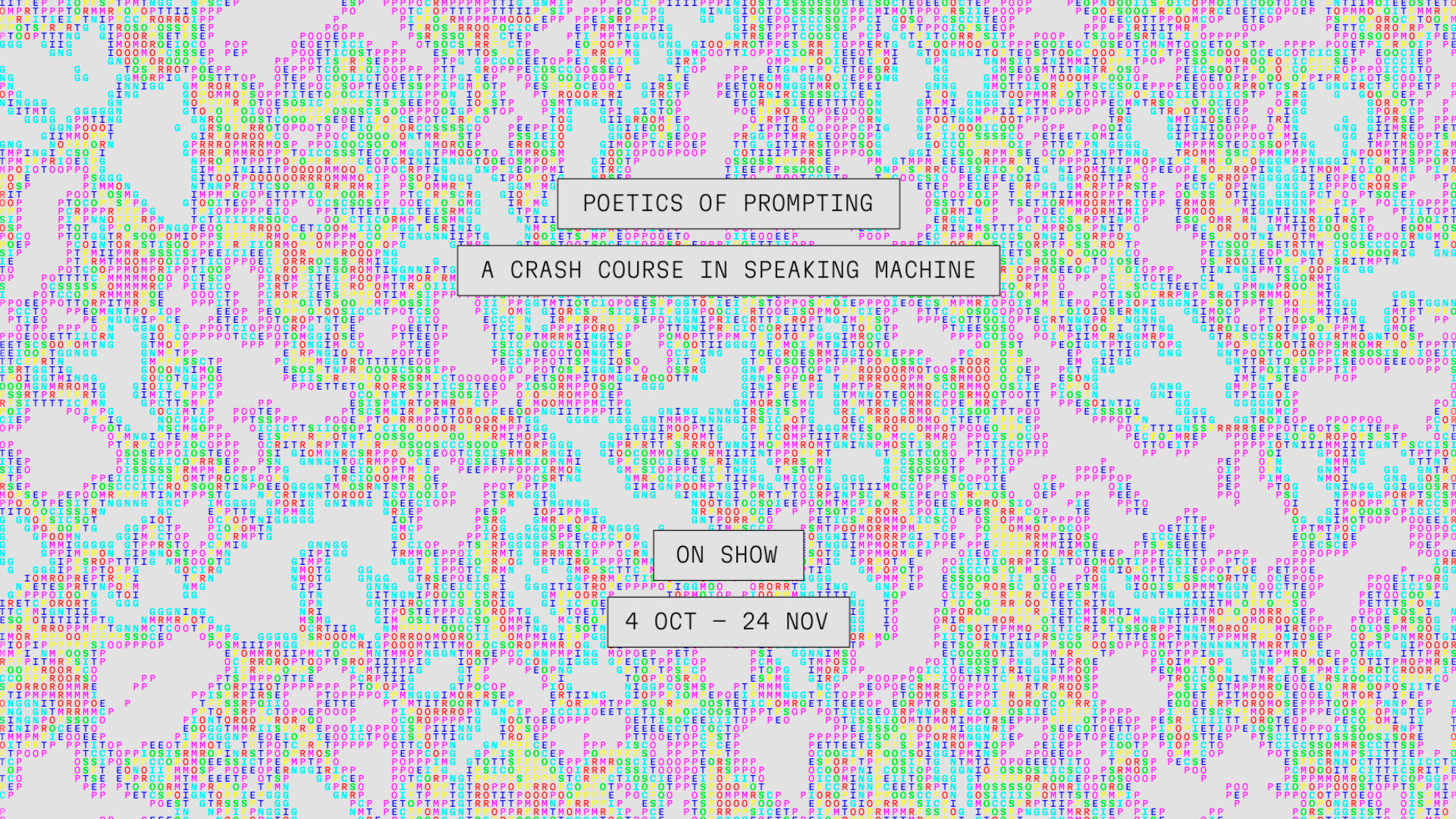
Poetics of Prompting brings together 21 artists and designers curated by The Hmm collective to explore the languages of the prompt from different perspectives and experiment in multiple ways with AI. In their work, machines’ abilities and human creativity merge. Through new and existing works, interactive projects, and a public program filled with talks, workshops and film screenings, this exhibition explores the critical, playful and experimental relationships between humans and their AI tools.
The exhibition features work by Morehshin Allahyari, Shumon Basar & Y7, Ren Loren Britton, Sarah Ciston, Mariana Fernández Mora, Radical Data, Yacht, Kira Xonorika, Kyle McDonald & Lauren McCarthy, Metahaven, Simone C Niquille, Sebastian Pardo & Riel Roch-Decter, Katarina Petrovic, Eryk Salvaggio, Sebastian Schmieg, Sasha Stiles, Paul Trillo, Richard Vijgen, Alan Warburton, and The Hmm & AIxDesign.