Writing Noise into Noise, Revisited
Revisiting the Noise Prompt in SD 1.5
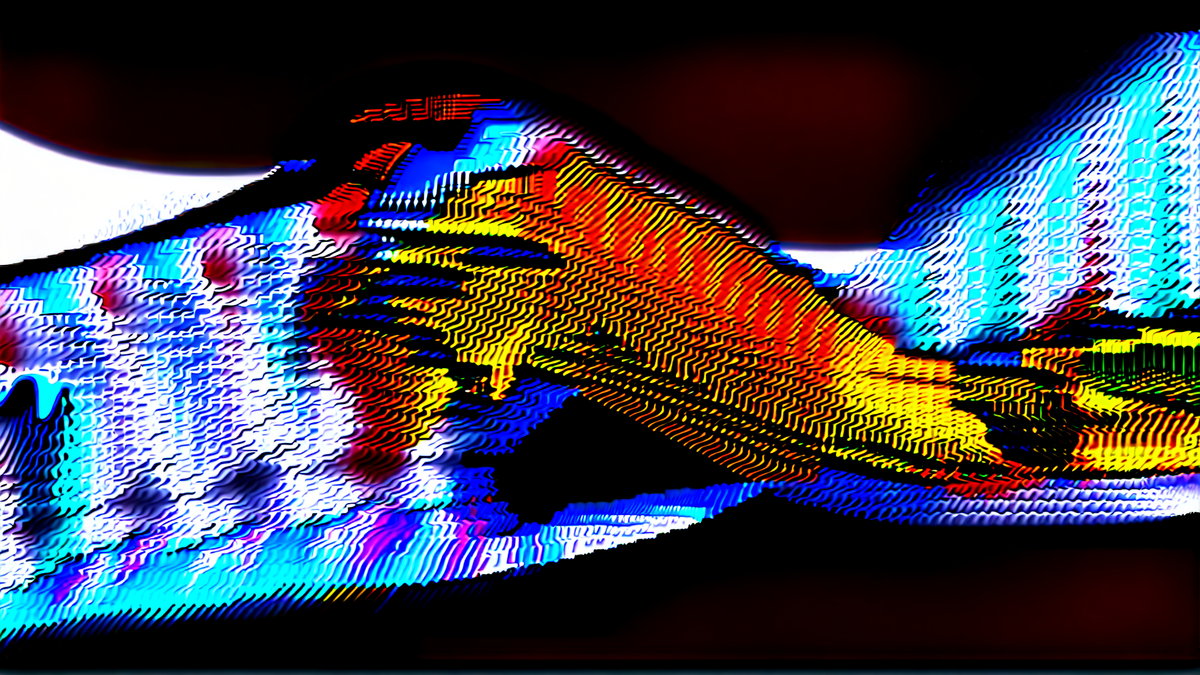
In the 1980’s, Margaret Boden wrote a book — Computer Models of Mind — dealing largely with computer vision and cognitive science. Of interest was how computational processes might translate the problems of computers seeing the world in ways that made them more or less compatible with a world of human vision. Much of it was in-the-weeds of both cognitive science and computer work (both up to the 1980s state of the art) but I was struck by this line in particular:
“Imagination is said to involve active thought, rather than being a repetition of a passive sensory experience.”
In this sense, computer vision has not changed much, nor has whatever we might call “computer imagination.” The AI generated images we see are more like a grammar, with structures inferred by training data and words filled in through paraphrase. Humans can make sense of these images because they are built upon references to 2D representations.
If we break that grammar apart — as the Dadaists and Beats did with cut-ups and other word games — we create a kind of language without references, which allows us to imagine how these aspects of language make new meanings within different structures.
Writing With Noise Instead of Data
I’m taking a class on Stable Diffusion this month lead by Derrick Shultz, and highly recommended, if you’re keen to know more about this stuff. It’s been an opportunity to revisit my first encounter with noise-based images and to think through how it works and what’s going on. It’s also lead me to think a bit differently about what AI images afford us, which is an important way of rethinking what it is that they cost us.
I want to emphasize that I acknowledge the problems of AI generated images in terms of how they are trained and the abuses they make more accessible. But I am also firmly invested in the idea that the AI image is a medium with particular properties, and that relying on them for the automation of past mediums is not only bad for creative labor, it is also just a boring use of a new technology. Many AI boosters compare the AI image to the advent of the camera.
I’ve said it before: the camera was a technological tool until artists discovered how to develop a language unique to it. The camera was invented, but photography had to be discovered, over time.
There are more straightforward ways of doing that, but the noise image was one way of thinking about what that language might be, and how the results resembles more familiar forms of feedback. The noise image is quite basic, and likely bound for obsolescence soon: already, new sampler techniques within the models make far more boring results. The results are far less interesting in more advanced models, and video models do different things.
Before I go much further, I want to talk about the process a bit.
A generated image is made pixel by pixel, but each step — or “draft” — is sharper and more refined than the previous. When you prompt the model for noise, it finds that noise instantly, because the source image of all generated images is an image of random noise, or a smeared blur. So the model locks on to that, asks an image recognition system (such as CLIP) if it “sees” what we prompted, and the recognition system likely confirms that it is — because it is noise. So then it passes on to the next step, a stage of refining edges in the noise, making clusters of noise around bits and pieces of that image to make it look more like noise.
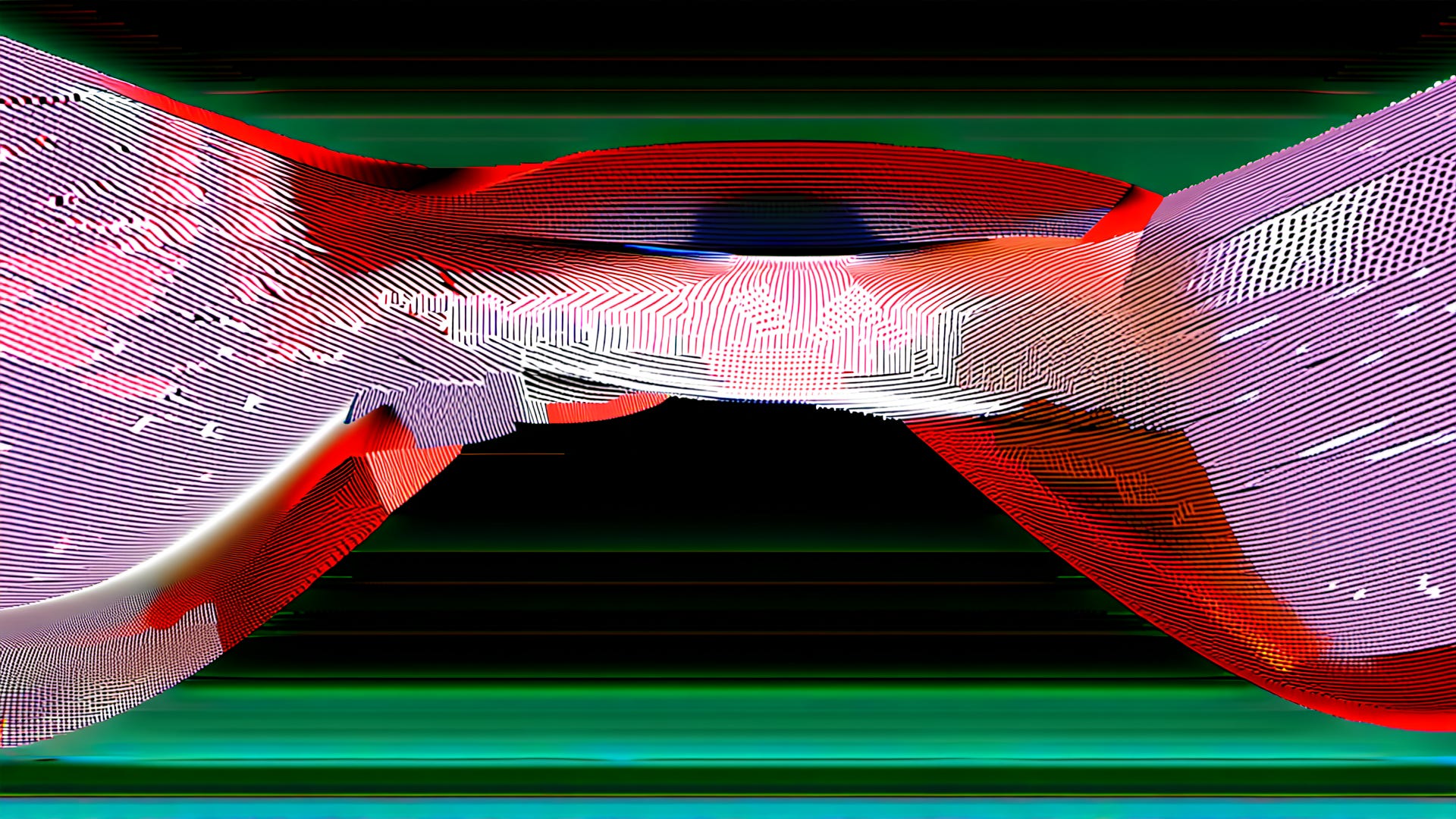
You can see where this going. Essentially, the resulting image is a “refinement” of an image which cannot be refined, being passed to a machine vision system that will accept whatever is offered to it. There’s no room engage the weights and biases of the model that was trained on any dataset. The noise is not constrained by training data, but allowed to represent itself. That’s why these images don’t resemble anything linked to noise in the training data for Stable Diffusion. There’s no engagement of that dataset’s imagery. So what we are seeing is a kind of revelation of the system’s writing process.
For a long time I showed this work and said, “I don’t think these are beautiful,” but I was lying. I actually do find them, at times, to be kind of beautiful. They’re an artefact of a rejection of the system — a result “of active thought, rather than being a repetition of a passive sensory experience.”
This is one way to make an AI generated image that qualifies as misuse of that system. It’s one visualization of that technique, and one way of thinking about how humans can insert noise into a system that is oriented to removing noise. I want to see myself as one more person in the line of artists trying to think about technologies that attempt to remove noise in order to amplify signals of their own choosing. Using noise is a creative misuse of this system, redirecting the attention of the system toward amplifying what it was designed to erase.
What is often erased by these systems is human agency. We lack agency over our data used in the dataset, agency over the control we have in calibrating the outcomes, agency in terms of making the systems do something we want them to do — rather than accepting images it makes and the way it makes them. To go outside the system and think about our relationship to that system is a different kind of agency.
To return to Boden: “Imagination is said to involve active thought, rather than being a repetition of a passive sensory experience.” Boden meant that what we envision in our heads, in the act of imagination (assuming you can visualize!) is the result of conscious attention. Whereas recall, or recollection, is repetition of what has already been seen. In computational terms, the diffusion model as it stands today is both the product and presenter of a passive sensory experience. To make it mean something else, we have to engage the system in a more active way: we have to think critically about the leverage points we have within this system, and how to use them to interfere with what is expected. The action that results is a way of personalizing the process.
I think it’s a nice way to make art with AI, but it’s just one way.
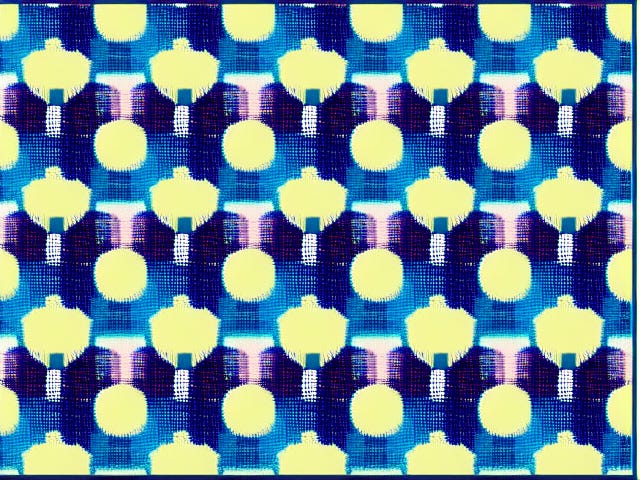
Artificial Intelligence vs Active Imagination
This is a very technical intervention in the system, and it’s one that I think appeals to those who don’t want to see AI generated images at all. But I think there are ways of working with AI generated images that are more straightforward than this, and I hope we see a deeper conceptual and ideological engagement with AI images as the medium develops.
Another method I have been exploring is the AI image as a critical response to the dataset. Richard Carter calls this critical image synthesis. Artists who work with AI images in this sense are thinking about the shapes these systems make and what they reflect, or visualize, about the structures that lie beneath their surfaces. To do that kind of work requires deep thinking about how datasets organize information, alongside how that information was obtained. It means thinking about what is adjacent within categories in the model and why. It means examining the organization of these images through the lens of historical contexts, surfacing and exposing that through AI systems.
My most recent attempts at this were embedded into the Ghost Stays in the Picture series, where I was able to discover the historical context of stereogram images and see how the AI literally fuses colonizing logics into the prompt — if you haven’t read part three of that series, you can read it here.
This work acknowledges that the aesthetics of a generative AI system’s outputs are shaped by political power over their categories. Using an AI-generated image (or more likely, a series of AI generated images) we can reveal the politics of those structures and examine how those politics circulate.
Ellen Harlizius-Klück has written that “Data does not organize itself,” and Alex Galloway has examined this quite compellingly as well. I’m definitely a product of Galloway, who I was bugging online since I was 16, and folks like Nick Couldry, who was publishing on data colonialism while I was in the media studies department of LSE (and was once forced into live-tweeting an early reading of a book chapter, having no concept of what we were about to get into).
The point is that digital aesthetics will always embed a kind of politics, an ideology of order that shapes them. Pretending it doesn’t just seems remarkably weird. And in rejecting the “order” imposed onto that data, even images as illegible as the one below are representing some form of political engagement with that system.
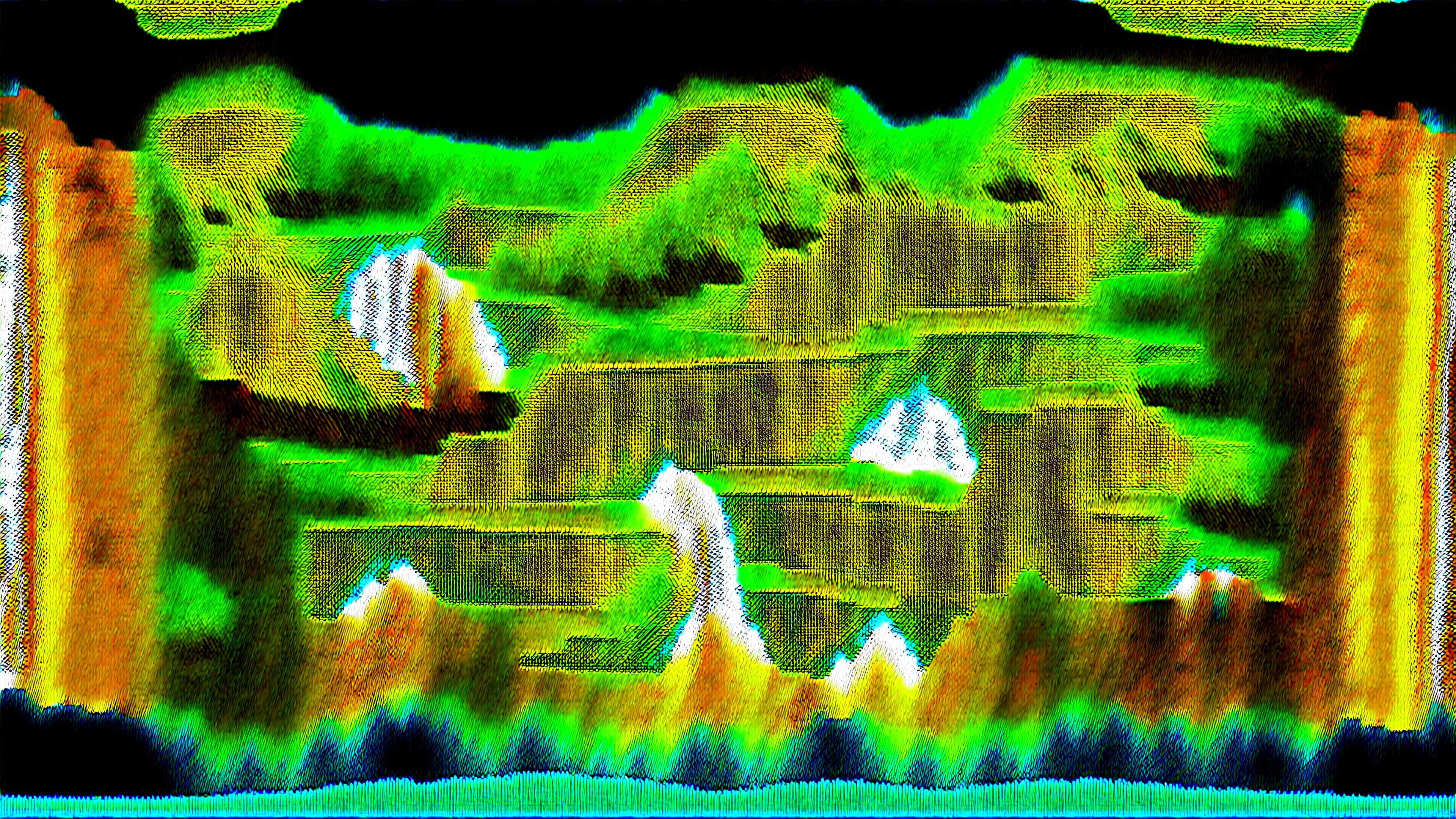
I don’t think I’m contributing to this theory that ideology shapes tech and tech shapes images, which is why I don’t really agree when I’m called a theorist. That politics is embedded into technology — and that we should pay attention to it — is factual. People can make the choice to ignore that fact, or acknowledge that fact, and we can all disagree on what to do about it. But I could never pretend there is no political dimension to aesthetics.
But People Hate Political Art, You Say
So I think it is interesting, and important, for artists to acknowledge this relationship in the work they make with these tools. Sometimes that isn’t intentional, but channeled into an intuitive visual expression in the use of these tools. If artists accept and understand any tool’s tendencies toward certain categorizations or structures, than artists can inject noise that fuzzies them up, shifts ideologies from unquestioned to questioned. Again: not the first to say this.
Certainly there is potential in AI images as art for thinking about them as assemblages of information, which are shaped politically, and working with and against those assemblages in order to challenge them. There are artists working with prompts and AI models that address this — if not explicitly, than by working sideways. Artists such as Kira Xonorika, Synthiola, Jake Elwes and fellow ARRG!ist Steph Maj Swanson are doing a lot of that work, whether they declare it or not, as are many scholars — my favorites being Nora Khan, Abeba Birhane, Roland Meyer, Matteo Pasquinelli, and Safiya Noble, though they don’t all deal with AI generated images per se. That is a short list of many!
I would not place my “noise images” into the same category, but I think the definition of “AI artist” in the current popular conception leans too much into the kind of creative industry definition of artistry, or really conservative definitions of art (“how long did that take to make?”) that make it impossible for artists to make work with a technology because people don’t like the technology’s politics. I think that is short sighted. There are some artists working with the technology who acknowledge the politics, and reject them, or challenge them, or push against them, varying from didactice to subtle.
It’s not a new idea. The risk of ordering the world for machines is in fact an old idea, and artists working against that order is a long and important tradition. I just want to understand how the power embedded into technologies applies to this new technical image. More often than not, my work is a way of finding footing within that.