Clip Art Doesn’t Come to Life
The AI Industry’s Creation Myth
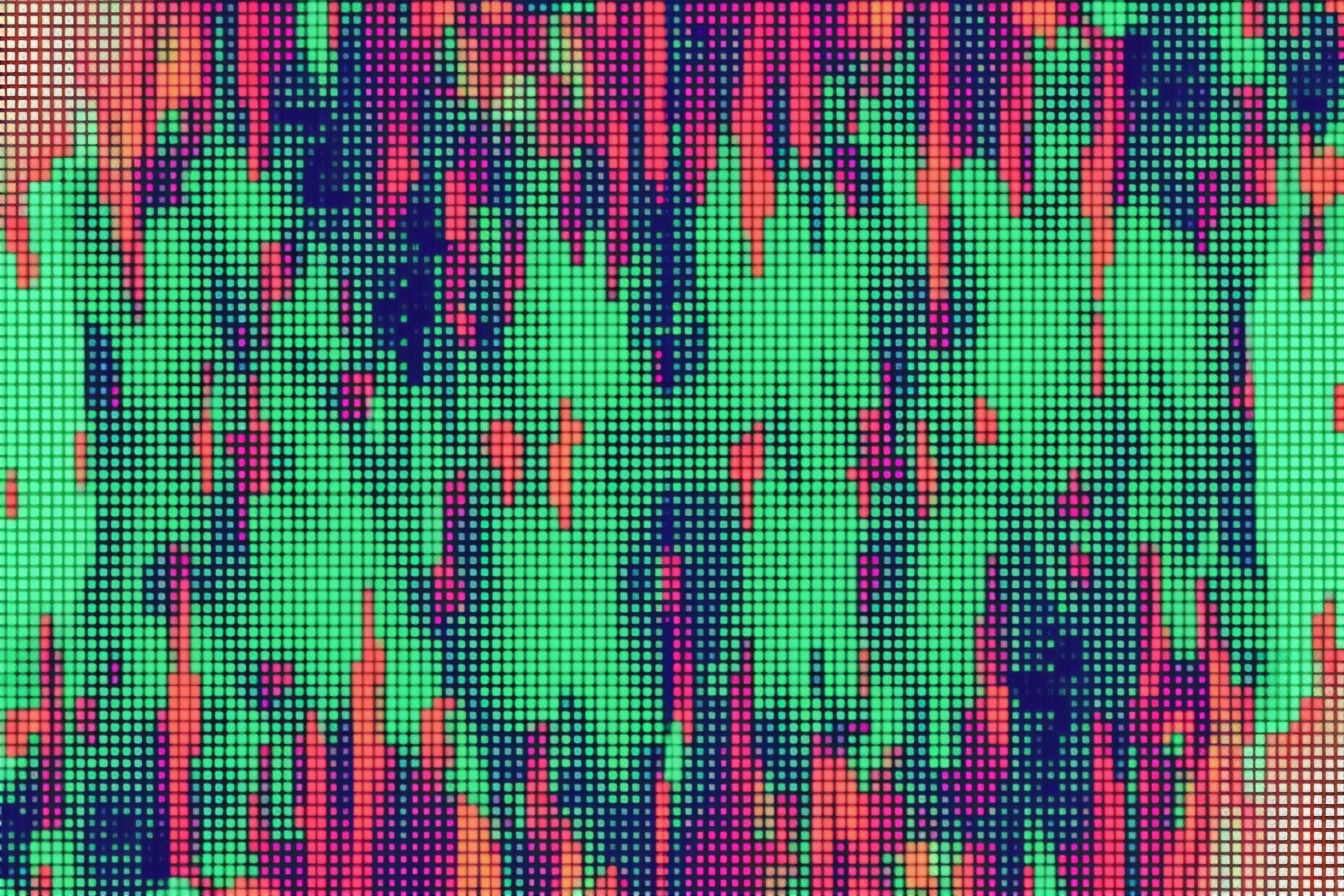
There’s a ritualistic element to the way some people talk about AI — particularly, what they call AGI, “artificial general intelligence.” To build a single machine that can rival all human capabilities, they say, requires us to collect a universe-sized pile of data and gather it in a single place. From there, through the mathematical alchemy of neural nets, data arrives at a kind of resonance, that resonance being a model of the human mind.
This is a deeply compelling mythology in that it mirrors the origin story of evolutionary science: the clustering of primordial cells in an arbitrary melange of chemistry that moved amino acids into microbes. Cells combine and mutate, and mutations drive competition and evolution.
That cells and information have interchangeable behaviors is the big dream of transhumanism: if so, uploading your brain to the web is just a matter of mechanics. In aiming to build a model of the human mind, the focus is on building a big enough puddle of acid from which microbes might emerge. AI companies focused on AGI are building that primordial soup from data, using it to trigger digital neurons. In the cascade that flows from a query, certain associations become stronger — and these associations form the next “mutation” of information.
The goal is to eventually cultivate a kind of “general intelligence” where one can ask one machine to tell us all kinds of things, but also to reason alongside us. Large Language Models, with enough data, are being positioned as a prototype for this form of computing, but they haven’t gotten us far enough despite being trained on billions of points of data.
Ultimately, much of AI’s present-day utility is elusive, and reveal a strange set of priorities. Did we really need to assemble some of the best minds in AI, and vast ecological and computational resources, to solve the problem of paying for commercial clip art or writing 2010-era Buzzfeed listicles? Do we need a machine that can generate video B-roll as opposed to, for example, solving the extinction crisis, curing cancer, or developing more efficient fuels or materials?
AI companies focus on media like pictures and text because of the limits of a data-driven approach: they require a dataset large enough to get results. OpenAI wants us to believe that these tools are steps toward AGI: that ChatGPT and DALL-E 3 are an evolution from simple to complex (but purely digital) “organisms.”
The Data Myth
OpenAI is selling us a creation myth. That’s not to say that the company hasn’t produced interesting new technologies. Chatbots and video generators do stuff. But they don’t quite do what OpenAI tells us they do. When OpenAI executives are invited to summits, hearings, and conversations with policymakers, the frame through which all this tech is filtered is the “eventually AGI” lens.
That distorts how we address their impacts on labor, communities, and education. It emphasizes hypothetical risks, and miscategorizes what this technology is and how it should be addressed.
Vast sums of data have been made available to these companies at unprecedented scales. That’s a result of how the Internet has been shaped: from an engine for finding information, as envisioned in the 1990s, to an engine for collecting information, as we’ve seen with the rise of social media and Big Data.
The argument at the heart of OpenAI’s progress is that this data is, and should remain, free for tech companies to use, and that it will deliver an ever-escalating set of breakthroughs. OpenAI and other tech companies argue for less constraint on the kinds of personal data they can use and how they can use it, because they need to build this bowl of primordial soup. When we see high definition video, even when it is flawed, we’re also being told that more data would make it even better.
This is a call for more funding, less regulation, and significantly weaker data protections for the rest of us. Today we see lawsuits against Stability AI for their use of Getty’s images without consent and the New York Times suing OpenAI for scraping its archives without permission. We see a mad rush from tech companies to sell this data, with Reddit, Docusign, and Wordpress announcing sales of the words, images, and even legal contracts that we shared online. Tumblr, in its rush to profit at the expense of its users, has apparently delivered private (and even deleted) posts to OpenAI.
This data is the blood in the arteries of artificial intelligence companies. Stop it, and the heart stops. That would be bad for the business model — and so the more product demos they can show us, the more they hope politicians will back off in the name of innovation. It is therefore in the interest of AI companies to justify data scraping with an onslaught of new spectacles made possible by this approach. Increasingly, these spectacles are described in near-miraculous terms to dazzle us with possibility at the expense of understanding them.
Sora, OpenAI’s new video generating model, is the latest case. Just as ChatGPT was framed as a model that “understands” language, Sora is framed as a model that “understands” motion, gravity, and time. OpenAI suggests that this new model is a “physics engine,” suggesting that they are sitting on an AI system that now understands not only language, but also space and time.
To be clear, Sora understands space and time about as well as a VHS cassette tape. Sora is still a Diffusion model: it is the same technology behind our current batch of image generators. If motion is represented accurately, it’s for the same reason that diffusion models can render a fruit basket with apples instead of clown noses. It’s about data. With video, they simply add another dimension (motion) to the cluster of data that generates these videos. They compress all of the frames of training data into a single image, then extrapolate new frames from that image, using machine learning to fill in the gaps. But motion is understood as how an image smears when every frame in a video becomes a single image: it isn’t a result of understanding the logic of gravity.
It’s very cool. But it isn’t any closer to reasoning than diffusion models were when they were applied to images. Some people imagine the models are making decisions, but they aren’t. They’re applying mathematical structures to random variables, constrained by prompts and the central limit theorem.
It would be more precise to say these models can successfully parse language or video — break it down into smaller units. These models do this in “training,” and are then able to predict relationships between these parts based on the relationships in their underlying datasets. In essence, these models can create maps of fragments, and then reassemble those fragments in statistically likely ways. They can do this without knowing what words are supposed to mean, or what time is.
Some people insist that what I’ve just described is a “world model,” a situation in which the persistence of certain patterns becomes reliable in the output of a generative AI system. By that definition, a VHS copy of Titanic is a “world model” too, because it can consistently generate a James Cameron movie. In other words, reliably generating completely consistent patterns is not an achievement of AI. It’s just storage.
Fundamentally, this “AI world model” frame is a political frame. It’s used to assert a certain kind of politics about the world and its meanings: that the world is fundamentally data, and that if we have access to enough data, we can recreate models of this world close enough to our own that a data-driven agent can interface with our physical reality seamlessly.
Calling reliably recalled data from a database a “world model” is a deliberate smudging of language, just as “learning”, “intelligence,” and other words in the AI glossary are.
There are limits, however, to what data alone can do. To have an AI system function at even a fraction of the level of awareness that a chipmunk or a goldfish exhibits in processing a real-time environment would require not ten times more data and processing power. It would require processing speeds and data compression at such a scale that the idea of AGI would be secondary, because we would have already solved massive problems in computation power & speed, as well as data compression.
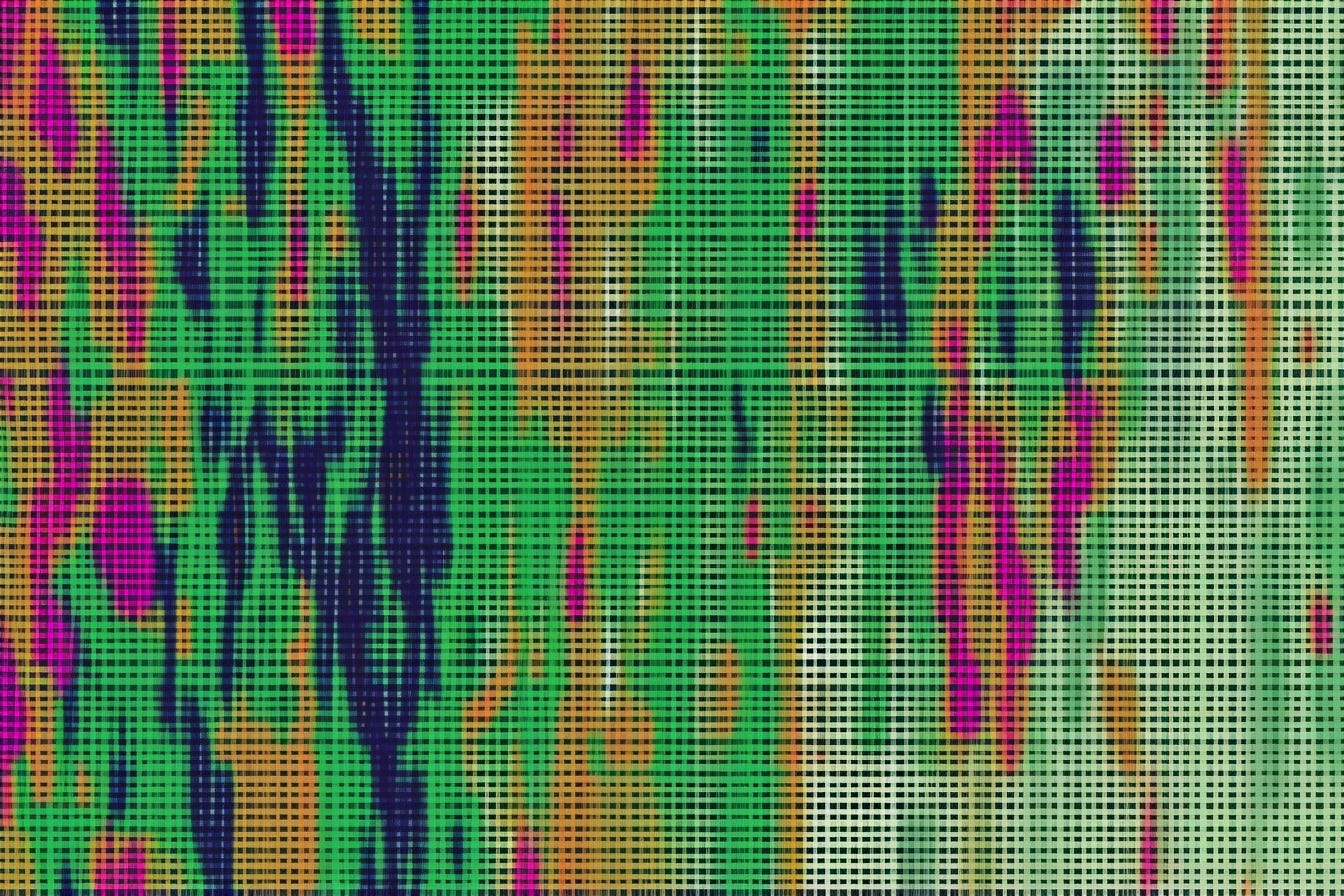
The Data-Driven Problem
The reason we see AI companies investing so heavily into the strange collection of products we see — why it’s designed to replace media, rather than cure diseases — is because they reflect the kinds of data that is available at vast scales and where randomness and constraint can be meaningfully shaped toward outcomes. We have years of video uploaded to the Web every hour. We exchange text at a pace that would fill our largest libraries in days.
The products OpenAI creates are constrained by available data. The more of that data they have the better they’ll get at rendering more of that data. That’s what these systems do: take vast amounts of data and use it to generate more of the same kinds of data.
The limits of tools that rely on vast datasets is that not every problem has a vast dataset that can be applied to it as consistently as “text”, “images,” and “video.” The more focused your dataset, the less data is in it. OpenAI, Google, Meta and others see that they have a vast collection of media data and want to make use of it. So they’ve come up with this idea, called “foundation models.” Foundation models are meant to serve as a foundation which can be constrained to deeper focus. The theory is that if an LLM can learn the foundations of grammar, then it’s only a matter of steering these models into specific use-cases in order to make broader results possible. If you have a language model that can read books, the theory goes, you can now feed it any textbook and it will become a subject matter expert, capable not only of articulating physics or chemistry for itself, but even applying an “understanding” to new and novel problems that have not yet been solved.
When policymakers and others invite CEOs such as Elon Musk and Sam Altman to offer explanations of AI, we are bound to hear about the inevitability of these AGI systems and the ways foundation models will contribute to them. We hear this in the language of policymakers, including Sen. Chris Murphy’s 2023 Tweet warned that ChatGPT “taught itself to do advanced chemistry," which is a classic AGI frame: the model did no such thing. It was trained on chemistry textbooks, and predicts text based on the probable word distributions in those books.
If many of OpenAI’s products remain solutions in search of problems, it’s because the products they’re showing us are not what’s actually for sale. OpenAI did not aim to be a free clip art repository or chatbot company. These are merely tech demos for OpenAI’s understanding of the world, which is that with enough data, humans can develop Artificial General Intelligence, or AGI: “AI systems that are generally smarter than humans,” in the words of Sam Altman. In his lawsuit against Altman, Elon Musk describes AGI as “a general purpose artificial intelligence system—a machine having intelligence for a wide variety of tasks like a human.” Microsoft engineers suggested that GPT4 showed “sparks of AGI,” but how far those sparks are from the bonfire of AGI depends on which of its hundreds of imprecise benchmarks you use to define it.
Language recombination, shaped by statistical probability, is different from understanding the world, and the language we use to describe it. What these companies built are successful at arriving at an output that simulates real thought, but does not reproduce real thinking. Nonetheless, each development is couched as progress toward that endeavor, and the spectacle of machine “creativity” and “problem-solving” is designed to offer evidence that, with enough money, data, and natural resources, we might arrive at this superhuman machine intelligence that could not only reproduce data, but reason with it to solve problems.
This, however, is only a theory, and it is a theory that lacks evidence. Each new step toward “AGI” actually reveals the flaws of this theory’s logic. Engineers at Google have shown that language models can succeed at tasks connected to their training data, but fall apart when pushed beyond the boundaries of that data. Emily Bender, Timnit Gebru and others have pointed out, AGI may not even be necessary for realizing the benefits of machine learning technologies. Instead of collecting as much data as possible, we may simply need to pay closer attention to the data we have. People reap clearer benefits from highly specialized, narrowly focused systems rather than attempting to build a “one-size-fits-all” system, which is what AGI assumes.
A recent paper shows that generative AI, trained on chemistry and biology data but using an LLM to translate that data into human-readable sentences, was able to generate a drug that could treat lung disease. That’s a different kind of approach to building AI — it’s narrow — though it was wrapped in a general LLM. That’s a more promising example, for me, than throwing all the data in the world in a bucket and expecting an understanding of the world to emerge. The drug-assembling bot did not need to “understand” the meaning of words or the weather. It found patterns and flagged the patterns scientists were looking for.
It’s evidence that a computational approach designed around finding needles in haystacks — ie, meaningful connections between data points — is more effective when there we have better curated the hay. Language models play a role here. Don’t get me wrong. But they didn’t discover this drug by expanding the data of a language model into infinity. They found it by focusing on the most relevant data to the problem.
Ironically, this is precisely what Sora, ChatGPT and DALL-E 3 show us. Focus on a specific kind of data, and you can generate specific kinds of outcomes. We could stop there. But we don’t.
The gather-lots-of-general-data phase isn’t necessary to produce meaningful results from machine learning. We can use these tools to guide our analysis of the world, not the modeling of it. The modeling of the world will always be trapped in past representations of it. But as tools for human thought, paired with specific goals and critical data analysis, there’s certainly a place for machine learning. But we don't need foundation models to build useful ML systems. We need good data, carefully collected, and applied thoughtfully to the problem at hand.
So why aren’t these tech companies building those tools? The answer I come back to is ideological: sophisticated tech demos such as Sora, GPT4 and DALL-E are a sales pitch for the contrary argument. These are spectacles designed to recruit new converts to the idea of delivering powerful autonomous agents — eventually. These technologies are often framed in misleading ways, shaped by the focus on general intelligence, that misleads the public about what they actually do. This frame, unfortunately, distorts our relationship to these systems and, to a large extent, what we should collectively sacrifice to make them possible.
We would do better to focus on “aligning” the data collected for these things to the purposes to which they are meant to serve. But OpenAI’s “alignment” strategy focuses on the outcomes of this process, fixing things that emerge from the model. It frames big tech as the only capable actor for determining how an AGI system is used. This is problematic for anyone interested in participatory democracies. Mona Sloan notes that this vision of AGI is “infrastructurally exclusionary,” that is, “it does not make room for diversity, multiplicity, unpredictability, and everything that “participation” might bring to the table.”
We have no proof that foundation models get us any closer to so-called AGI, or any better at solving bigger problems. What we have seen is that this approach does improve the believability of text generation models, the resolution of synthetic video, and the visual complexity of fake images. These are notable achievements, in the grand scheme of things, though we don’t know quite how they’ll be used. We should be concerned with the risks associated with those developments. But they are not a sign that fantastical technologies of tomorrow’s corporate monopolies are imminent. No need to protect those monopolies in the present.
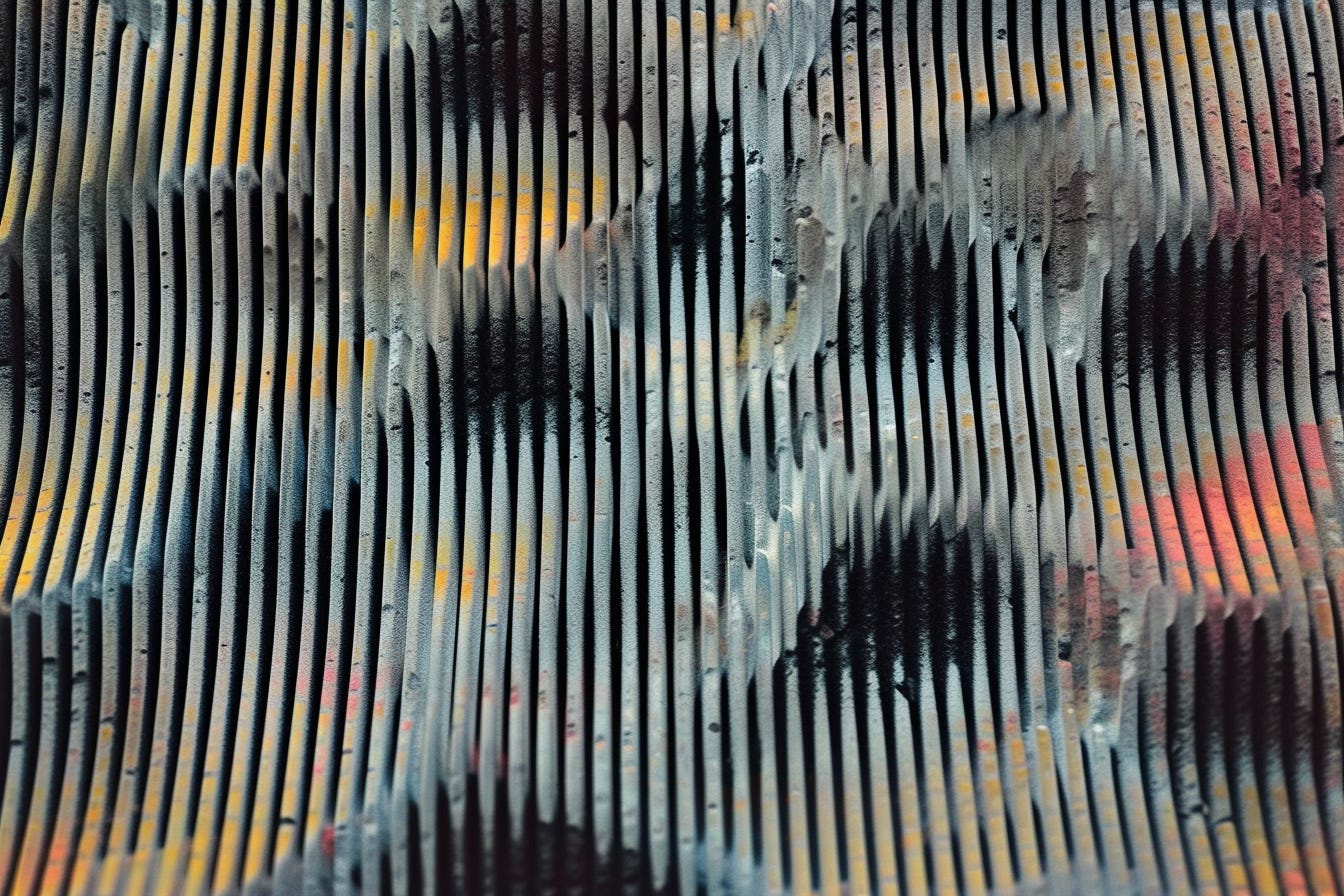
Things I am Doing Soon
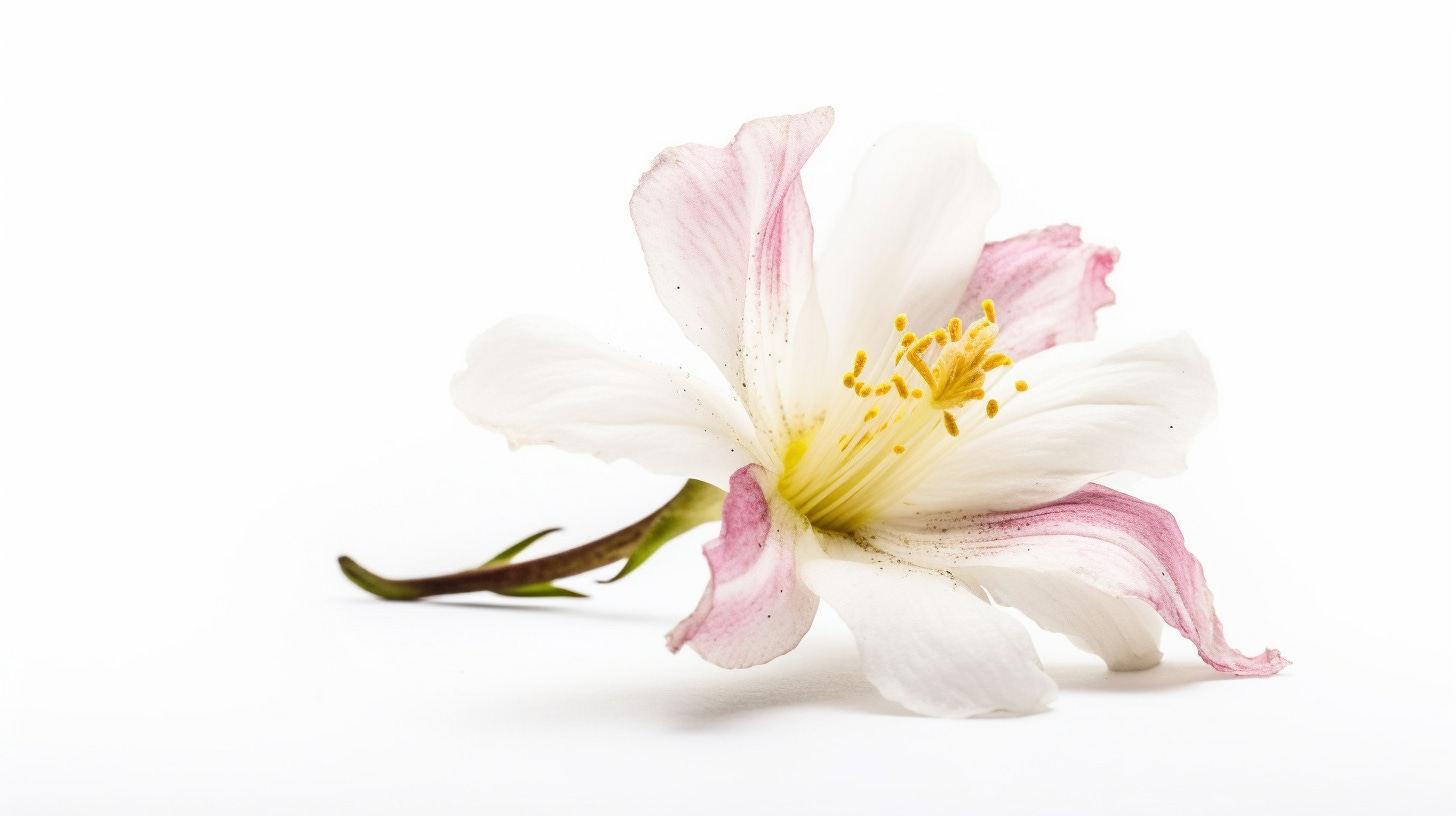
Dublin, Ireland April 2 & 3: Music Current Festival
Flowers Blooming Backward Into Noise, my 20-minute “Documanifesto” about bias and artist agency in AI image making, will be part of an upcoming event at the Music Current Festival in Dublin. The Dublin Sound Lab will present a collaborative reinterpretation of the original score for the film alongside the film being projected on screen. It’s part of a series of works with reinterpreted scores to be performed that evening: see the listing for details.
I’ll also be leading a workshop for musicians interested in the creative misuse of AI for music making, taking some of the same creative misuse approaches applied to imagery and adapting them to sound. (I’m on a panel too - busy days in Dublin!)