Einstein Gazing at the Water
New work inspired by the history of diffusion and pollen.
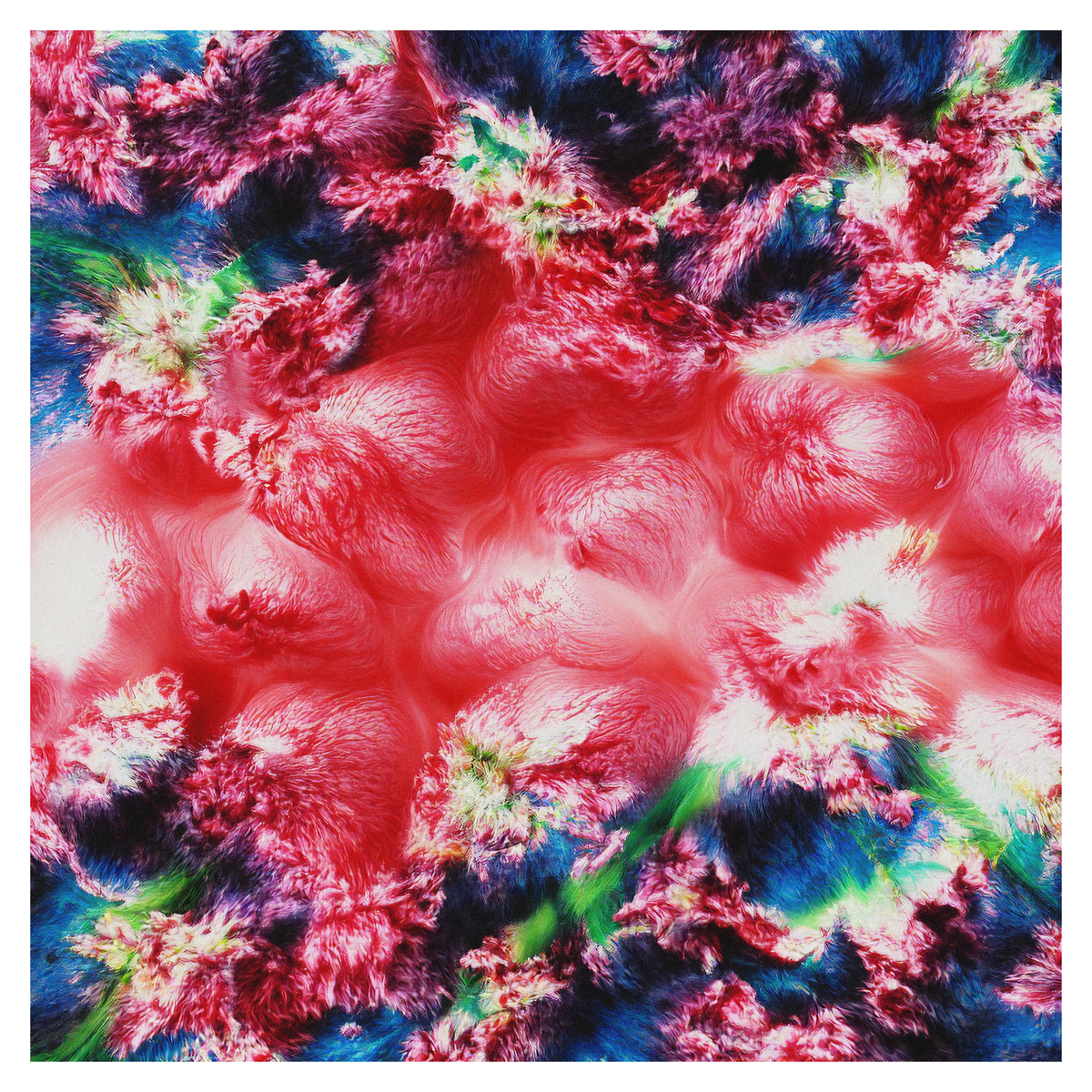
On AI & Pollen Landing on the Surface of Lakes
(Look, it’s been a hell of a few weeks and I am posting about art today, but a heads up that my latest piece in Tech Policy Press has some of your “it’s been a hell of week” covered).
Pollen was once imagined to have agency, because it moved above the surface of water as if by its own intention. In 1827, a scientist named Robert Brown baked some pollen – killing it – and dropped it into water, to see if it still moved. It did. Sentience was off the table.
In 1905, Albert Einstein went for a walk along a lake and observed pollen on the water's surface. His realization that what had once been deemed "intelligent behavior" from pollen was the result of complex flows of unseen bits called molecules. That insight would lead to his first major scientific publication. He called this idea – that pollen and other particles spread out further over time – a diffusion equation.
In generative AI there are diffusion models, and they're tangentially related. In AI, diffusion models refer to the spreading out of noise within an image in a process that moves information further from the source in every step. AI borrowed science for a metaphor, as it always does, to explain this spreading of noise throughout the images used for training data. Knowing how it spread, you could denoise that spread to restore the image. Start with random noise, and it would correct the noise to make new images out of related concepts.
Noise, in computer systems, creates an illusion of liveliness. Perlin noise is used in video games and CGI to procedurally generate "clouds, fire, water, stars, marble, wood, rock, soap films and crystal." Gaussian noise is more scattered.
Noise pollinates the image set, giving the model a sense of agency. Start with the same image of noise and ask it for different things, and you get similarly structured different things. Below you can see a seed generated by Stable Diffusion (left) and two images that come from that seed.
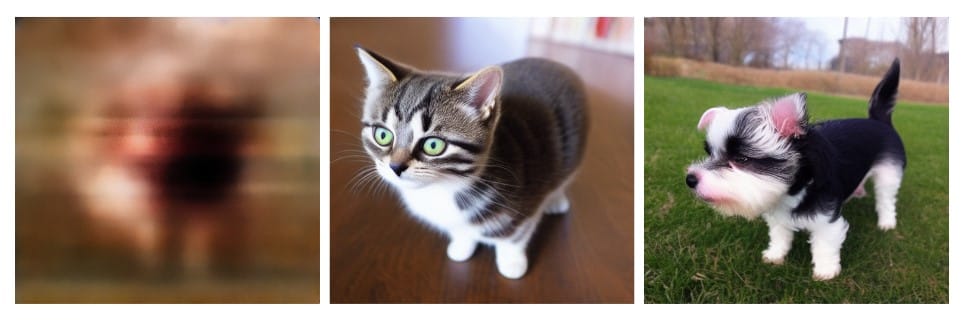
When you adjust the "temperature" of an LLM to include more variety, you are essentially expanding the acceptable threshold of noise in the system. With images, you are literally adding noise to the image in order to find new possibilities, constrained to any instructions you provide.
Ask for a new image of a small cat or a small dog, and you generate a new seed. Keep the same seed, you get the same cat, same dog. The noise structures the picture.
Pollen and noise have this relationship to each other: an illusion of liveliness, which is one thing when alighting on ponds to be observed as a choreography of molecules, and another when an AI system is mistaken for imposing creativity into a jpg. Of course, each of these is worthy of appreciation: diffusion models are elegant, even if the business models, and the purposes to which they are used, undercuts the awe that many of us might otherwise feel.
Art, Pollen, Noise
I've written before about my relationship with AI as being adversarial – to paraphrase Nam June Paik, "I work with generative AI in order to hate it properly." As The Algorithmic Resistance Research Group, I (along with Steph Maj Swanson and Caroline Sinders) premiered a number of works at the hacker convention DEFCON 31 that looked at the early potentials of glitching and hacking AI systems.
I always described my work as trying to take imagination out of AI, in contrast to the many AI artists who aim to build on top of these corporate imaginations. My theory of change was recently confirmed in a bit of business research: "efforts to demystify AI may inadvertently reduce its appeal, indicating that maintaining an aura of magic around AI could be beneficial for adoption." That is to say, the less you know about how these systems work, the more impressed you will be.
I create work as a form of research into these tools. I accept a certain degree of complicity. Sometimes it feels shallow and complicit to make work the way I do and sometimes it feels really necessary. The issue of complicity is centered in my work, though not always clearly. Addressing complicity makes stronger work because complicity with the tech industry is part of what it has meant to be American.
We use systems that fund bombs, we agree to share data that is used for surveillance — the house tech has built has a lot of rooms and very few of them don’t have peepholes. For that reason, using AI to attack the foundational logic of AI is, on one level, just about the same as using Google Maps to navigate my way to a political protest, or posting anti-tech threads on X.
There’s a responsibility that comes with it though, and navigating what complicity requires is a big part of why making work in this way helps me to think lucidly about what AI does and how so many assumptions behind it need to be dismantled.
So I try to counteract the myths and assumptions that lie at the heart of AI models. To challenge that aura of magic, I try to understand what they really are and do, and make work that points to its own sources. In this way, I hope we can counteract the spectacle of AI.
Much of the work that I make was an artifact of a research practice. AI-generated images of visual noise circumvent part of the image generation process that references datasets of any kind. They create bizarre and unexpected abstractions through short-cutting the internal image recognition systems these tools used to compare your image to the prompt used to shape it. Through the prompt window, I would trigger a loophole that circumvents the training data.
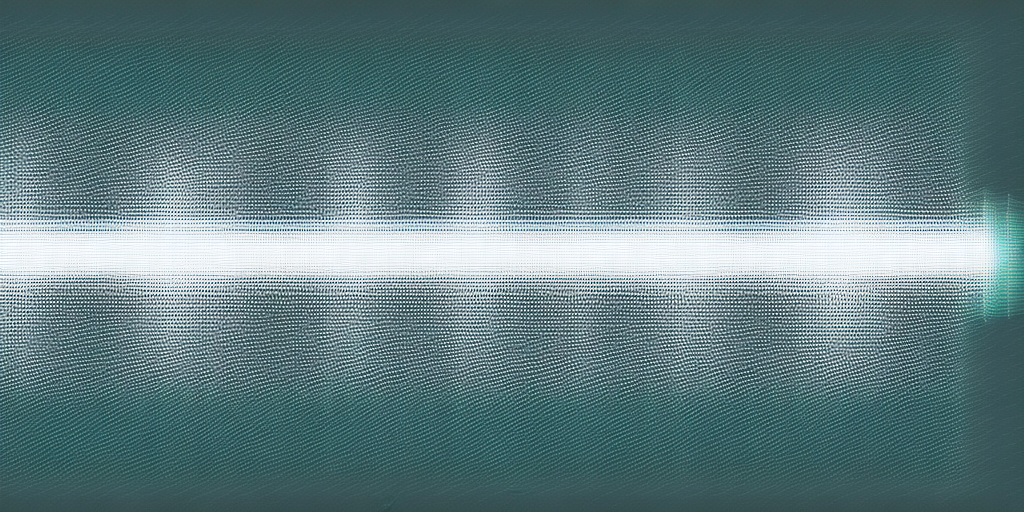
In the image above, I have asked for an image of a certain kind of noisy digital image. The seed is created, and an internal vision model is tasked with assessing whether the image contains the prompt. "Is this a dog? Is this a cat?" In those cases, the vision model would say no, there is no dog, let's refine this.
Instead, this image and prompt poses the question: "Is this noise?" The answer is an immediate yes. From there, the KSampler takes the image and refines it according to the number of steps, making it "a clearer image of noise," whereas the image recognition system cannot offer any refusal: the image is always noise.
The Noise of Pollen
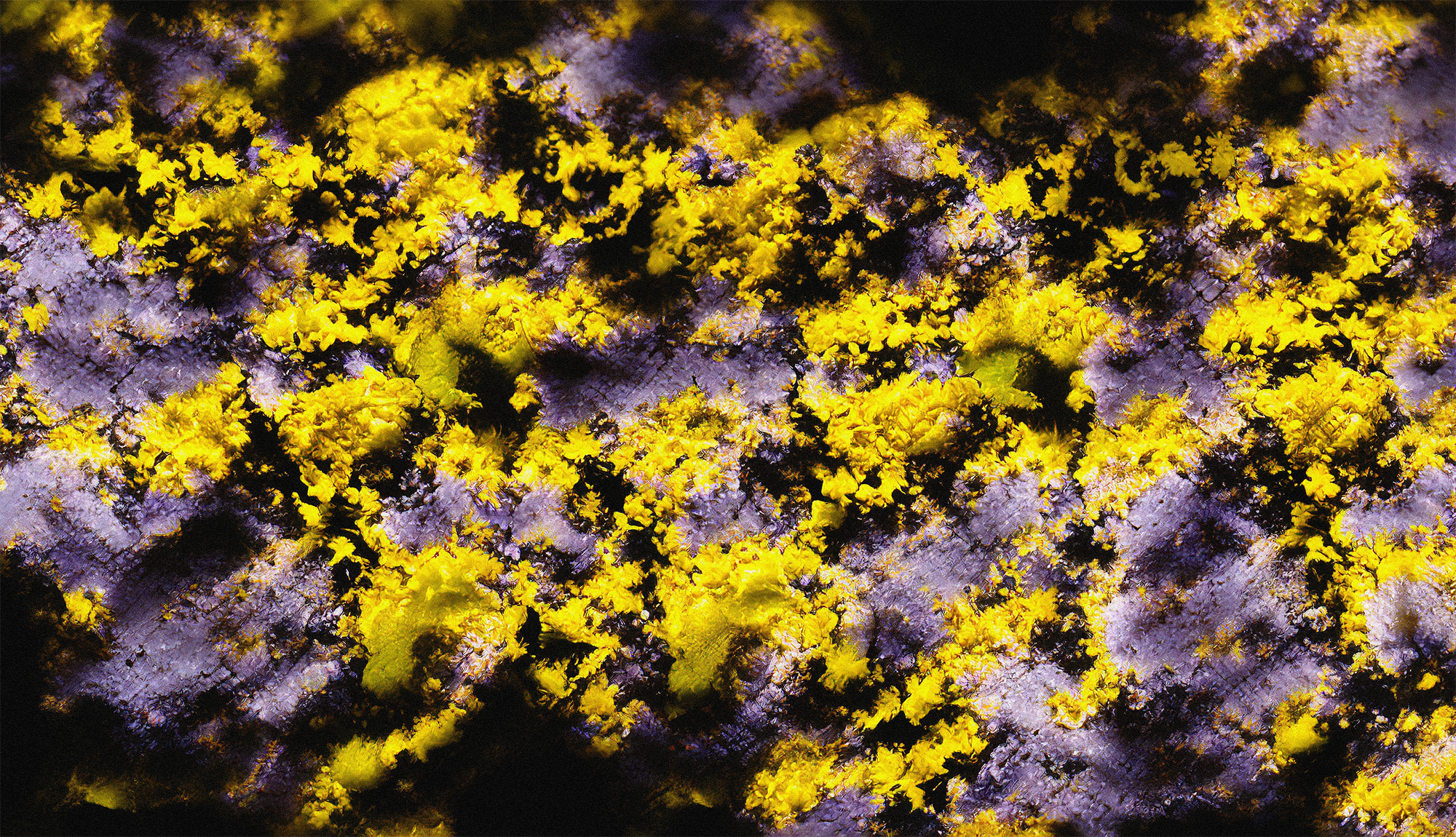
I've been thinking about pollen while beta-testing Public Diffusion / Inference since mid-December. This noise process doesn't work: it doesn't rely on CLIP. However, I wanted to make the most of the freedom of Public Diffusion as a tool: I don't actually have to "defeat the system." There is no data in the model that undermines artists, no child abuse imagery to haunt my experience of prompting.
I used existing glitches I'd produced and used them to image-search the training data inside PD, a 12-million-images assortment of public domain images. The search engine gave me a series of images from history that resembled such noise: many of them were marbled book covers; damaged photographic prints, scientific images from labs; and yes, stray glitched images and static.
Taking these as a source, and then requesting the model to work with them as a seed for creating images of pollen, gave me these. They are probably not what most people would do with an image generation tool, and so these images, as a "product demo," probably don't do much. I'm ok with that. I will say, the textures and richness of these images surprised me.
I am very happy with these images, and the process of working with a source in an attentive, careful way. There is something satisfying about connecting this idea of noise, pollen and diffusion to the archives and the outliers of the public domain.
The glitch images I've made with Stable Diffusion challenged the machine to produce something without reference. In these images, I am generalizing the visual vocabulary of the outliers in the archives. The debris of visual culture landing like pollen on the surface.
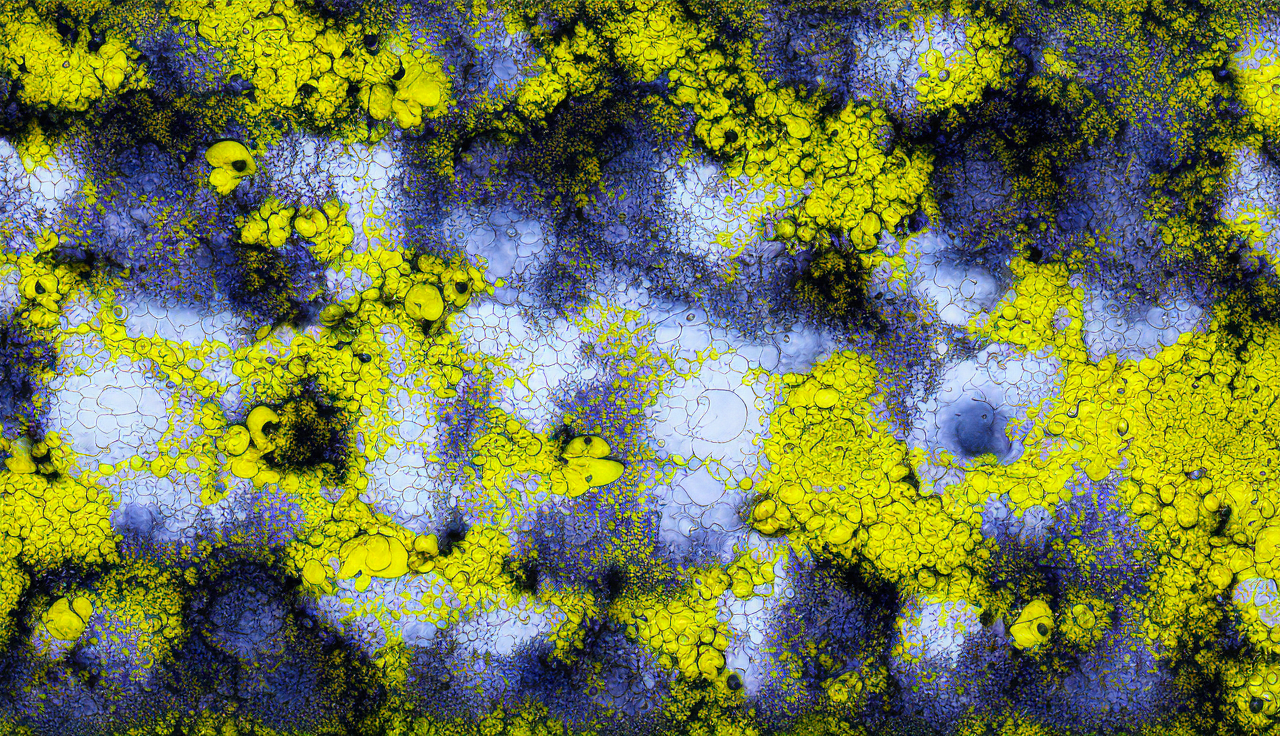
I intend to print these on paper to complete them: turning scanned paper archives back into paper feels like karmic regeneration, in a skillful sense of thoughtful re-engagement and re-contextualization, as opposed to non-consensual exploitation. To that end, if anyone is interested in showing a broader selection of these works, please let me know.
Phantom Power Podcast
Really happy with this discussion of AI, art, music and noise on Mack Hagood's Phantom Power podcast on sonic culture. Video is above, but you can find the audio version wherever you get your podcasts!
AIxDesign Fest!
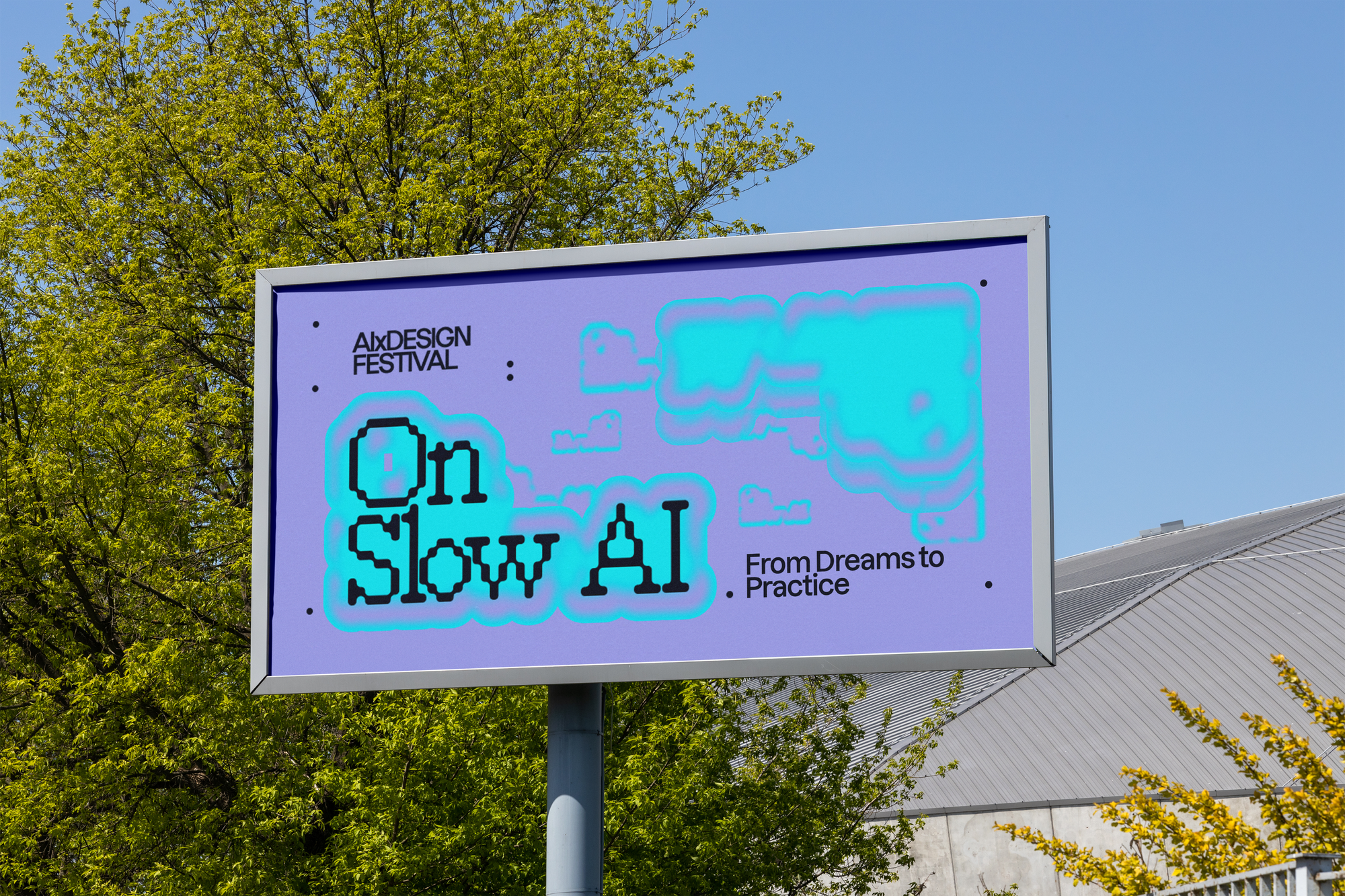
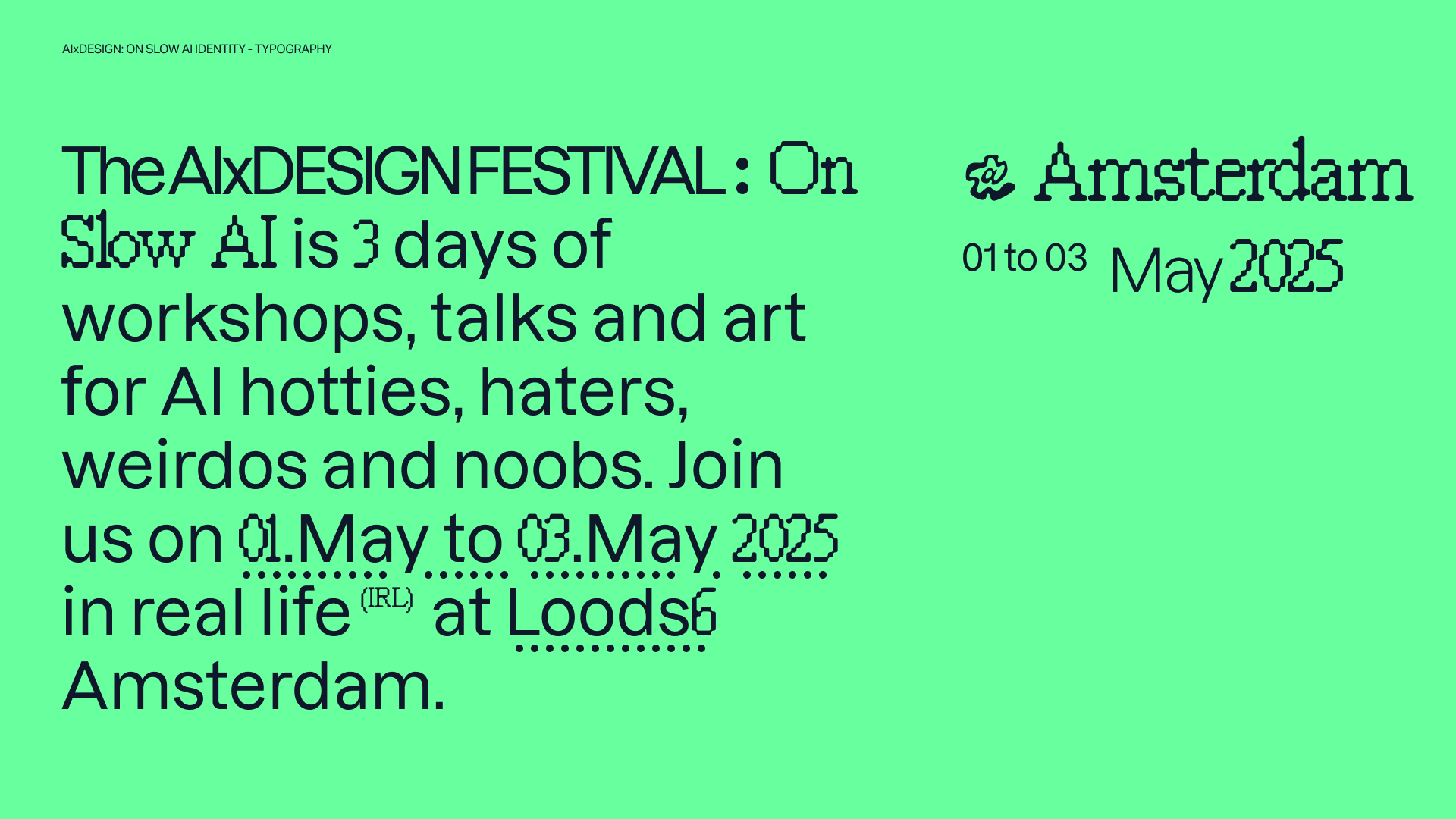
Excited for the upcoming AIxDesignFestival: On Slow AI which will happen in real life in May in Amsterdam! I'll be a speaker at the event and I am really excited for it. Right now they're also raising funds to support a livestream of the event – if you want to help support it, you can score some swag and the money will go toward your ticket!
Bluesky Things
If you're on Bluesky, I've got some things that may be interesting for you.
- A starter pack of Critical AI thinkers from all kinds of perspectives, which I've promoted here for a while. But there's an expanded pack, with even more Critical AI folks, which is well worth a look.
- A similar starter pack for Artists working in Technology.
- A custom feed that shows you good, no-hype tech journalism. Pin this, and you'll have a tab on your Bluesky account that gives you access to tech journalists - minus the product launches and video game news.
- Clicking on any of those links will ask you to set up an account if you haven't already.
A Note to Subscribers
I recently cleared out a large number of accounts that subscribed to this email list but never read it, or had bouncing emails. It was a bit of a blow to the old ego. If you're a fan of the newsletter, please recommend it to someone who might dig it!
Here's a link to the archive, where people can subscribe. You can also sign up below!