How Does OpenAI Imagine K-12 Education?
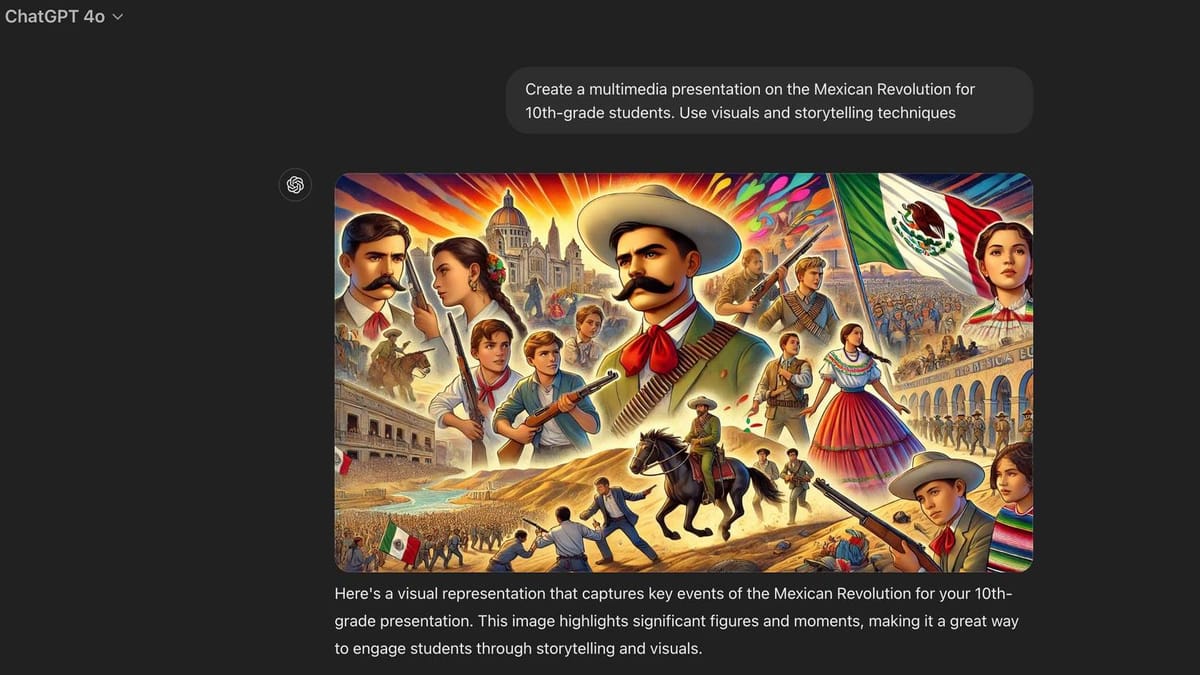
Close Reading OpenAI's training module for educators
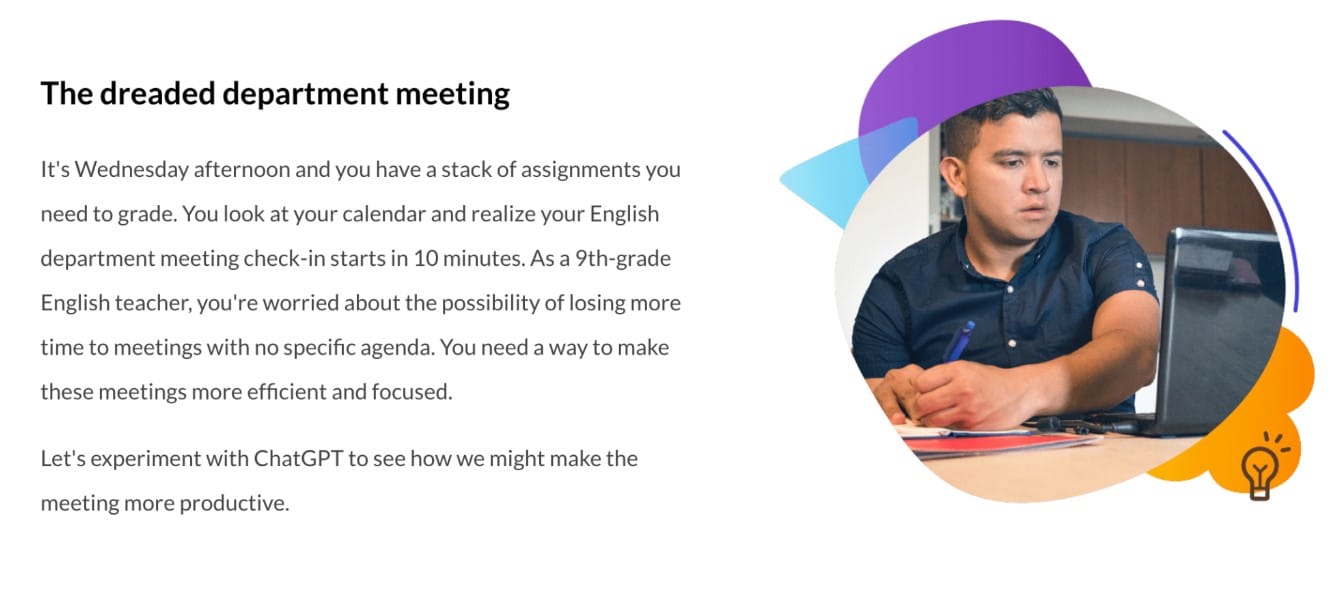
If you’re taking a free online training, it's helpful to understand who wrote that lesson plan and why. ChatGPT Foundations for Educators is a course created by the non-profit Common Sense Media, in partnership with AI behemoth OpenAI.
In an article in Tech Crunch, Common Sense Media frames its position in developing the course this way:
“With this course, we are taking a proactive approach to support and educate teachers on the front lines and prepare for this transformation.”
I'm a bit skeptical of this frame, because transformation is positioned as inevitable. Perhaps it is. But the program is designed to “educate” teachers to “prepare” them, which assumes a real passivity among educators concerning the tools they choose to use in their work. That comes at odds with a participatory approach to developing educational tools — ie, empowering people to make better decisions. So, immediately, the course positions educators as passive recipients of technology who must direct it appropriately.
That's worth remembering, because OpenAI has had little engagement with the experienced teaching professionals who are now asked to use its products.
I'm not here to diminish the need for AI training for educators, or to chastise Common Sense Media's involvement with OpenAI. Rather, it's useful for me to look at what this relationship produced, as a way of making sense of the kind of thinking that OpenAI is engaged in around education.
One criticism of the course’s design is that its videos lack closed captioning. That's a problem from accessibility, and little mention of accessibility is acknowledged here.
In the sections below I want to provide some useful counter-arguments for what the OpenAI course is "teaching." My goal is to offer more nuance to its definitions and highlight the bias of its framing. Much of this will be analyzed through the lens of my piece on "Challenging the Myths of Generative AI," which offers a more skeptical framework for thinking through how we talk about and use AI.
Also, while I am a researcher at the metaLab (at) Harvard University's AI Pedagogy Project, this analysis represents my thinking only and does not speak for anyone else involved in that work.
That said, let's sharpen our pencils.
What is ChatGPT and how does it work?
The first segment of the OpenAI course focuses entirely on ChatGPT. Immediately, it defines AI using what I consider to be OpenAI’s fundamentally flawed understanding of its own technology. It focuses on OpenAI's vision of AI too confidently as if it were present-day reality. Here’s their definition of AI — note how it frames the aspirational as already achieved:
“Artificial Intelligence is a technology that allows computers to do things that have historically required human intelligence. It’s like giving a computer the ability to learn from experience and make decisions based on that learning. AI helps people by learning from lots of information and figuring out how to answer questions or perform specific tasks.”
Contrast this with the definition of AI provided by the AI Pedagogy Project:
“AI encompasses a broad set of technologies that rely on large amounts of data to make predictions or decisions. Over the past twenty years, as the ability to produce and store vast amounts of data has increased dramatically, so have the possibilities of building technologies that incorporate AI, like more precise GPS navigation, email spam filters, and search engines.”
Having a perfect introductory paragraph defining AI for unfamiliar audiences is hard. The framing matters. The OpenAI description frames AI as already capable of skills requiring human intelligence, and the metaphor of learning is posed uncritically. OpenAI is building tools that they believe will lead to computers that behave and reason like people, and this has distorted their thinking about AI since the beginning.
Likewise, it presents its machines as “figuring out how to answer questions,” which is misleading: at best, they guess the answer based on statistical probabilities. More appropriately, they infer the sequence of words that surround the words in your prompt, and we all hope it connects to reality.
If the goal of this course was to create AI literacy in educators, this is a weak start. But I think this course defines literacy not as a critical capacity but as applied fluency.
Defining Literacy
The challenge in defining literacy in any emerging technology is that few people are literate enough in that technology to know what such literacy entails. The result is that those with technical expertise are allowed to describe the technology on their terms, baking in the ethical decisions they have already made to deliver the product. But the ethical compromises that create a working product are not always enough to cover the concerns of those being asked to deploy it.
The challenge in defining literacy in any emerging technology is that few people are literate enough in that technology to know what such literacy entails.
Aside from the ethics of the technology, AI raises significant concerns over our conceptual frameworks. These are highly seductive technologies, prone to be trusted without evidence and relied upon in lieu of human reasoning. Misunderstanding how they function increases the risk of being deployed in contexts where these functions create, rather than remedy, harms.
But technical expertise in a system is limited to the system — it often fails to account for what happens beyond the scope of the tool's most direct use case.
Technical literacy is often limited to, or shaped by, an internal perspective of the system's mechanics. In other words, OpenAI builds a tool to get a tool to work. Teachers must reinvent the tool to get it to work in the classroom. One set of standards does not apply to the other.
Module 1: How Does AI Work?
Appropriately, OpenAI’s course suggests that “AI models learn by analyzing large datasets and recognizing patterns,” which contradicts this idea that they somehow “figure out” how to answer questions. That's a useful clarification. But more troubling is this: “The more data they process, the better they become at tasks like predicting the weather.” I suspect they mean that there are AI models that predict the weather, but this is framed as to suggest that ChatGPT, or Generative AI, can predict the weather. It can’t — if it’s connected to other models, it can draw information from those models and report on what those models predict. But an LLM cannot predict local weather patterns on its own.
This is misleading in an important way, because it suggests a form of knowing the world that extends beyond the predictive nature of the LLM and into something predictive about the experience of the world.
What is missing is a solid grounding in what these models do, which is predict words. Predicting words is a core component of understanding how and when to use LLMs. The frame they propose here preserves too much mystification and invites too much misunderstanding to be truly helpful.
Module 2: What can ChatGPT do?
In another (also uncaptioned and therefore inaccessible) training video, the goals of using ChatGPT are:
- Increase Productivity
- Support with planning and scaffolding
- Rethinking your pedagogy
I’ve written before about the productivity myth. In this course it might be summed up with their phrase: “Imagine cutting your lesson planning time in half. What would you do with that extra time?”
As I wrote in the "Myths" piece,
The productivity myth suggests that anything we spend time on is up for automation — that any time we spend can and should be freed up for the sake of having even more time for other activities or pursuits — which can also be automated. The importance and value of thinking about our work and why we do it is waved away as a distraction. The goal of writing, this myth suggests, is filling a page rather than the process of thought that a completed page represents.
For many educators, the time saved by using AI comes at the cost of time spent checking and reshaping what it produces. There is a good chance that AI generates the rough equivalent of an educator simply rushing their work—especially if it is intended to cut planning time in half.
But why are we saving time? We can also save time by undercooking fish, but it’s not ideal. What is lost when we outsource planning time and attention to the shape of the curriculum? In most cases, teachers have been trained to do this and know how to do it well.
We can also save time by undercooking fish, but it’s not ideal.
The second part of the course's question — “what would you do with that extra time?”– is worth answering, too. Time exists in a context, and the push to productivity in overwhelmed contexts, such as schools, makes this question more of a threat than liberatory. For one example, the pressure on productivity is not likely to go unnoticed by administrators, who may demand teachers use these technologies to accommodate an increased burden or add additional tasks to their workload. Pretending the administrative bureaucracy and social pressures of teaching don't exist is a frequent myth of tech in general.
Generative AI shifts the burden from educators' work—the art of thinking about what they want to teach and produce inscribing that structure into the text of a syllabus – to other areas demanded by school systems. Gen AI produces text that resembles the structures of a syllabus those teachers work to produce. The syllabus is not the goal, it is the result of the thought that went into structuring the course.
Removed from agency over that role, instructors can do more administrative work. Famously, the machines get to do what people want to do, freeing people up to do the things they don't.
But perhaps we needn’t worry about what we’ll do with all that extra time anyway. The course does not make a convincing case for any time savings. The first lesson we learn about saving time with AI is that “iteration is key,” that is, the contradictory statement that we save time by tweaking our requests repeatedly in a prompt window until we get satisfactory results, rather than just outlining the lesson plan ourselves.
They propose a specific framework for prompting. I will say it’s pretty helpful in steering models toward desired outcomes. From a technical standpoint, the company knows how to use its tools. The problem is that it doesn’t understand the contexts in which its tools are deployed.
ChatGPT for Shifting Pedagogy
The next section of the course discusses the use of multi-modal features, that is, the ability for ChatGPT to create not just text but also images and charts. They show one example of generating tenth-grade classroom materials for a history lesson on the Mexican Revolution, in which all of the men inadvertently have giant mustaches, all of the women look identical, and all of the child soldiers are white. All of this passes without comment in the OpenAI course.
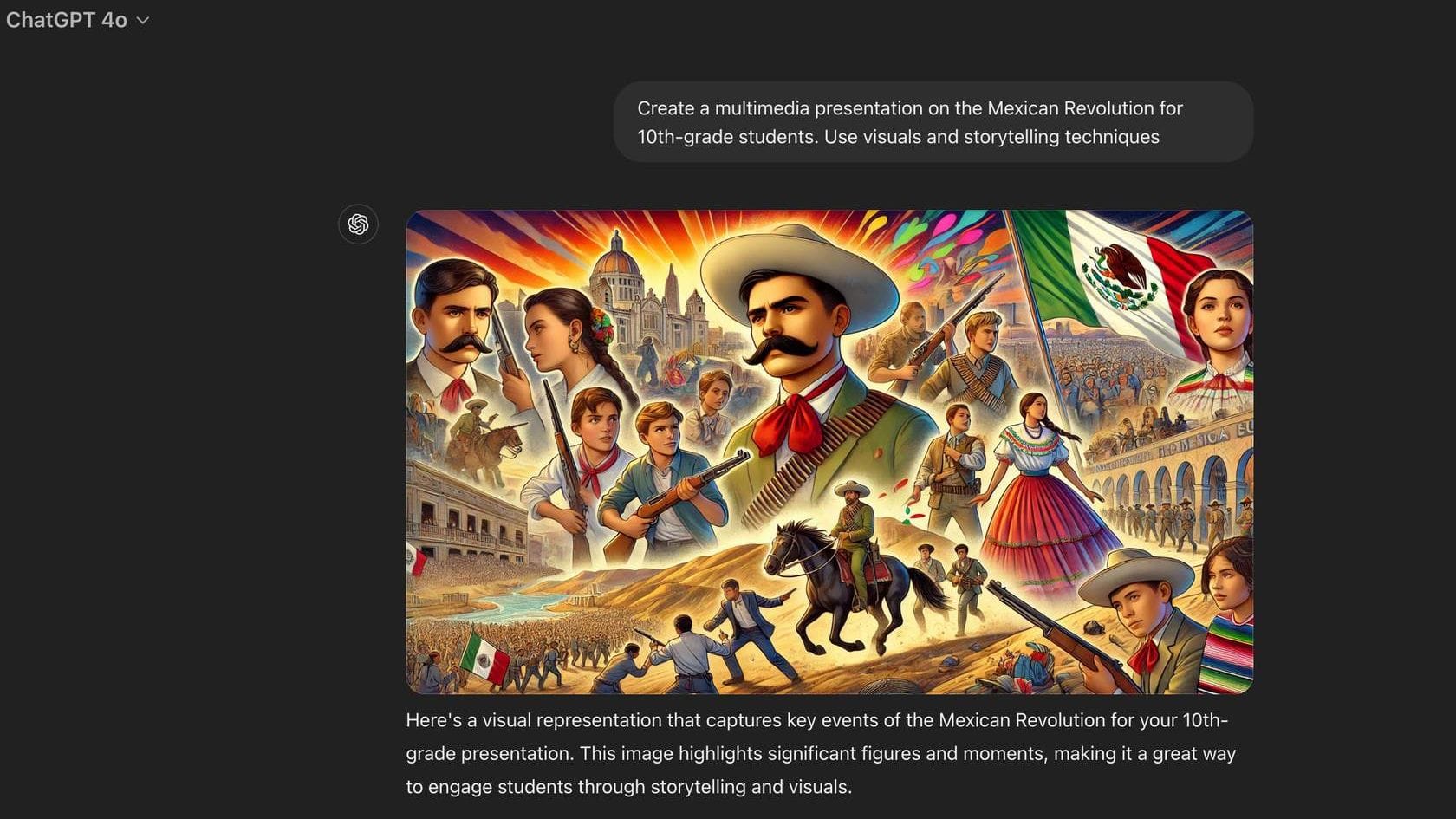
The recognition of bias is saved to the end, and much of it is left—as with other concerns—to the instructor to solve. In essence, the course suggests that AI's efficiency for educators is in saving time, but that this time can be spent solving much more complex social and ethical questions about the tool they use to save that time.
How does OpenAI view ChatGPTs limits?
Tacked into the end of this simple lesson are the more critical and self-reflective aspects of the course, where OpenAI frames the limits of ChatGPT. These limits include “Socratic conversations with your students,” asking students challenging questions that get them to examine their perspectives critically.
Paradoxically, it is also framed as not being good at “tailoring lessons to meet the unique needs of each student.” This is good advice. However, later on, in a video summarizing the teacher’s role, the instructor is tasked with “reflecting on whether AI is providing meaningful and personalized learning.”
In conclusion, ChatGPT's limits are summarized as “ChatGPT is not a substitute for your engagement with students.”
Defining Responsible Use
To its credit, the course does not use the term “hallucination” at all, rather referencing the potential for inaccuracies directly and clearly. The advice on inaccuracies is another contradiction: you need to verify any statement before you share it, meaning your time burden shifts from lesson planning to fact-checking yet another student’s work: that of the chatbot.
The challenge here is that to save time, ChatGPT would need to be trusted to take over areas of pedagogy where an instructor identifies a gap in their own knowledge.
A history teacher may know plenty about some aspects of the Mexican Revolution but not the Cuban Missile Crisis. ChatGPT would be more likely to be used for areas where teachers have weaker expertise. In this case, the same teacher would need to rigorously verify any Cuban Missile Crisis material. But how? How does ChatGPT present an improvement over other social resources, for example, by summarizing a Wikipedia article?
The course admits that ChatGPT may generate content that reflects harmful biases. As for sorting this out, though, you’re on your own: “you can reduce its occurrence and impacts by critically thinking about when and how to use generative AI.”
I agree that critical thinking is the solution, but the tools to empower that critical thinking are missing here. In many ways, the frames used here get in the way of building a critical toolkit.
Humans in the Loop
The course ends with a (yet again inaccessible) video emphasizing humans' role in determining the output quality.
I found this segment incredibly frustrating. It is ultimately a testimony to the hollowness of promises around ChatGPT in education. Each section speaks to the instructor's need to view the video to decide how AI could be used, ultimately making no arguments aside from “it should be used” and the evidence-free promise that it saves time.
I'm frustrated because it shifts the responsibility to teachers to discover how to use these tools in their own practice. For example, one slide simply asks the instructor to “identify how AI can support your professional growth.” Another burden shifted to the instructor is to “develop guidance to help your students use AI responsibly.”
Hey, OpenAI: isn't that why I took your course in the first place?
The frame assumes that AI can be productive and time-saving but asks teachers to brainstorm the specifics. It offers only a superficial and weirdly framed understanding of how these tools work, which almost begs educators to deploy them in unsuitable ways.
OpenAI is outsourcing the problem of AI in education to teachers and asking them to find a solution. Of course, I’d be just as critical of an overdetermined set of use cases. However, the problem is framed as “AI can save you time,” without evidence or proposed use cases. Instead, the instructor gets a checklist of problems to solve.
Tech Crunch adds this:
According to Allied Market Research, the AI in education market could be worth $88.2 billion within the next decade. But growth is off to a sluggish start, in large part thanks to skeptical pedagogues.
To the skeptical pedagogues, I thank you.
Thanks for reading Cybernetic Forests! The archive for this newsletter can be found here, and you can also sign up there for future newsletters (usually once a week on Sunday).
If you're looking to escape from X, I recommend Blue Sky. It's now at a level of polish that rivals Twitter's glory days. If you sign up through this link, you can immediately follow a list I've hand-assembled with 150 experts in critical thinking about AI. You can find me there at @eryk.bsky.social.