Human Noise, AI Filters: "Mr. Watson, Come Here."
Remarks on AI, Noise, and Creative Misuse for the Artificial Intelligence & Contemporary Creation conference, 24 May 2025 at the Jeu de Paume, Paris.
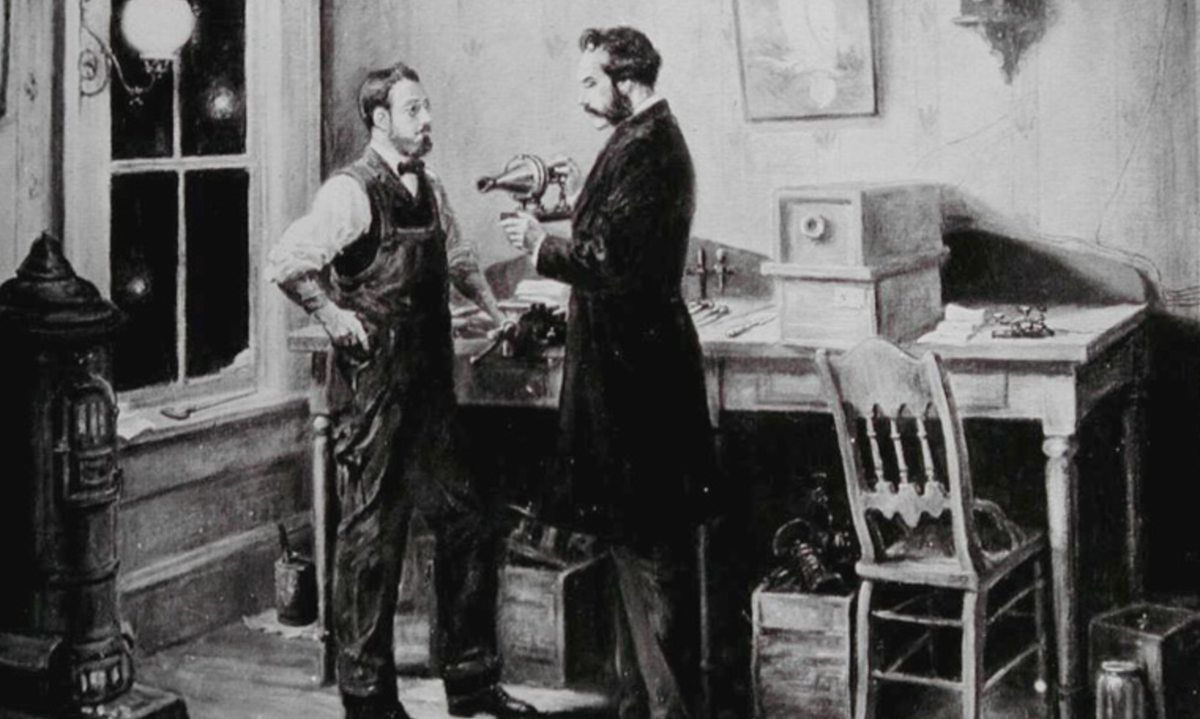
Remarks from the Artificial Intelligence & Contemporary Creation conference, 24 May 2025 at the Jeu de Paume, Paris.
Thank you to Antonio Somaini for the introduction and invitation here today.
I would like to begin with a new myth of noise: the story of Thomas Watson, Alexander Graham Bell's laboratory assistant.
Even before the invention of the radio, Watson had a peculiar relationship with the sound of transmissions. In his journals, he describes himself staying awake into the night; his ear pressed to the test speaker – listening to the strange noise within the wire.
Hillel Schwartz, in a passage from his colossal work, "Making Noise," speculates on Watson's nighttime entertainment:
"Was a snap, followed by a grating sound, the aftermath of an explosion on the sun's surface? Was something like the chirping of a bird a signal from a far planet? What occult forces were just noticeably at work in the recesses of telephonic sound?" (Making noise, p. 330).
I was in a similar position when I began working with AI-generated field recordings and room tone, the ambient hums of empty spaces. I noted myself intuitively scanning this ambiance for clues of what was taking place in these pockets of the latent space, trying to assess their shape and any presence within it through the tonality of the hum.
Of course, there were no rooms. No needles inscribing vibrations into the grooves of wax cylinders. No tape heads magnetizing audio strips. There was nobody in the room, because there was no room. The audio of a diffusion-based model approximates a room tone through sonic mimicry—a stochastic parrot, in its most literal sense.
For Watson, the sound on these wires was an amplification of the cosmos, fluctuations of cosmic events beyond his comprehension. A lingering effect of a fantastic expanse leaving its traces in a piece of copper and some magnets. It was a black box, too: perhaps, someday, identifiable, if only we listened well enough.
How shocking, satisfying, and strange, then, it must have been for Watson on that fated day when the sound of the cosmos did give way to a voice: the voice of a technologist, calling him by name, paired with a simple demand:
"Mr. Watson, come here; I want to see you."
Today, we are all unwitting lab assistants, collecting data for the invisible wires for the sake of being seen by the machine. In return, the machines repeat our names, seducing us with a similar call:
"User, come here; I want to see you."
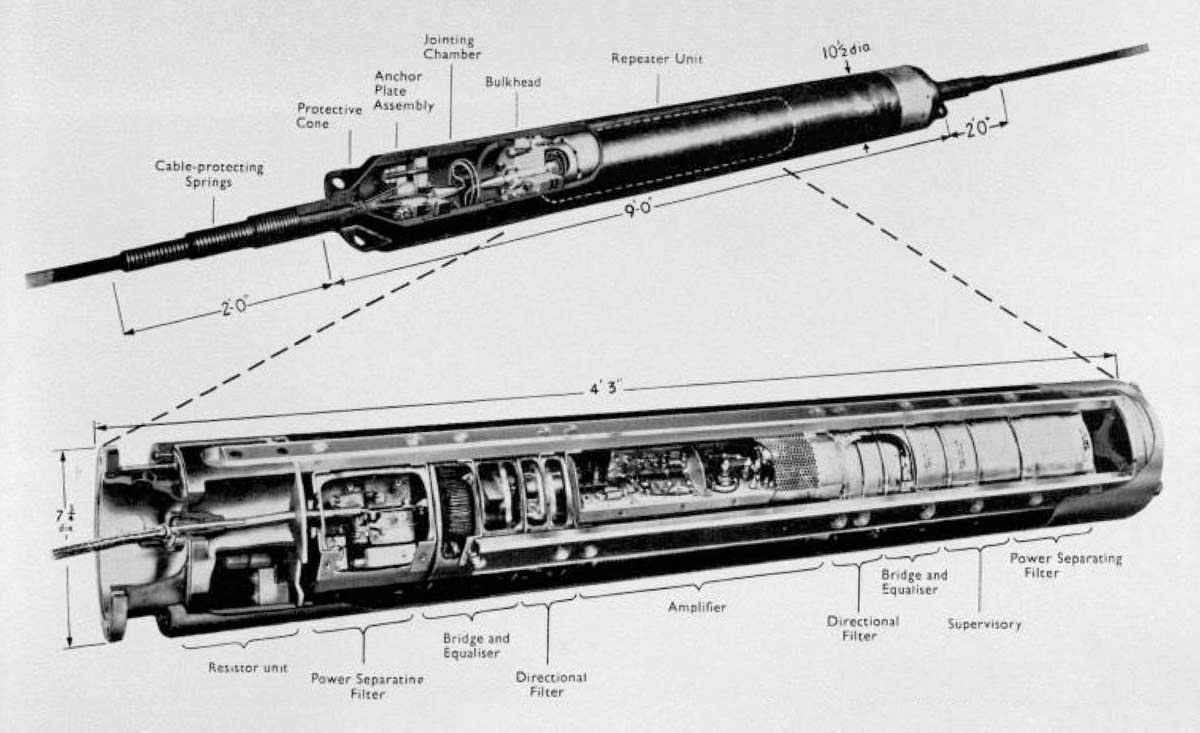
Any sense of awe for this noise in the wire quickly gave way to its utilitarian focus: to move the world out of the channel so we humans could occupy it completely. To create a perfect conditions for the technology to operate, vast expanses of wiring would need to be isolated from any possible event. The event might be wind rattling the line or trees cutting the wire completely. It could be overcrowding, wherein isolated voices break their barriers, creating interference known as cross-talk—solar radiation. Lightning strikes.
Today, the world of the wire has dissolved into wireless networks, and what data solutions salespeople claim is "a cloud." They offer a false sensation of anti-materiality to match our emerging false sense of anti-corporeality. The data center does not exist, we are told, by the same people who tell us that intelligence does not need a body.
Despite these shifts, the struggle to define noise and signal is still lurking within the design theory of artificial intelligence systems. These systems evolved from a simple neuron: on and off, a one or a zero, a stream of light passing through the hole of a punchcard or else being stopped.
Early robots, like Grey Walter’s tortoise, moved toward light and stopped in the darkness. The zero and the one divided the world into two categories: the one, a legible kind of measurement, and the zero, an absence of legibility. This, by the way, was at the time called a neuron. It is a simplification to suggest that a one is a mark of a signal and a zero is a mark of noise, but a zero is absolutely an absence of an electric signal.
Social media was once a quiet observer, like the compression algorithms in a telephone wire designed to carve out and block interference. Social media measured us and made sure our streams were satisfactory. But soon, the social media wire had observed enough, and then algorithms could predict and sculpt the conditions through which our signals could be amplified. In the age of the AI-voice-in-the-wire, we come back to this:
"User, come here; I want to see you."
We are called upon to provide a signal, and we are rewarded with dopamine when we do. The strong signal travels through the social media feed; the weak signal stands still.
Noisy Human
"User, come here; I want to see you."
What does it mean to be seen? Sarah Ahmed's work in "The Promise of Happiness" is worth referencing here: the calling out of familial shame. We only want what is best for you, as Ahmed's example, can be wielded as a means to encourage a child toward conformity and away from themselves.
This phrase wields a coercive power in which the concern is not about your well-being or living as you are, but the need for control by those who express such a concern. To such a parent, and to the algorithms of AI, which "see" us in similar ways, the "problematic" child is not seen but is measured, compared, assessed, and assigned a category. The child, or the user, is less seen than measured against, with the standard of measurement being a kind of aggregated user who behaves according to the known patterns that create an algorithmic logic. If the category is appropriate, the difficult child is invited into the parent's – or platform's – hospitality. But if you betray the category or are illegible to the measurement system, you are a non-signal. You are noise.
We can start there – to a resistance to categorization – and move into critical media art. The American theorist Sarah Watkins Fisher describes the position of critical media artists as a form of “parasitical resistance.” She suggests that these artists act as parasites on the hospitality of tech companies and their platforms. We are constantly negotiating between resistance and complicity, testing the limits of what is permissible within a system in order to reveal or subvert the rules and boundaries of that hospitality.
AI creates a particular strand of platform hospitality. They extend this hospitality to us through interfaces and mythologies about what the technology will do for us, all of which carefully erase the source of this power, which is often the users themselves. AI invites us into its summaries of the World Wide Web, distilling countless perspectives from a range of web pages into a single, authoritative voice. In the arts, we all know by now that the training data is taking the artist's material, packaging it, and selling it in new forms to other users.
So what can we do, as artists, to test the hospitality of AI systems? How might we become noisy humans?
Three Noises
Noise enters, as a metaphor, into AI systems in many ways. I will name three.
First, there is the Model Architecture. This is not a metaphor: diffusion models require noise to operate. We will discuss this in some detail in a moment.
Second, there is what I call Slop Infrastructure: their capacity to produce, at scale, an amplification of algorithmic culture that floods out specific signals in favor of plausible signals, creating a sea of noise in which authentic communication becomes nearly impossible.
Third, AI is a political structure, as seen recently with Elon Musk's DOGE takeover of the American civil service. The AI industry pushes AI upon us in a rush to resolve the tensions of politics, a way of diminishing the vibrant, unpleasant struggle of political debate, deliberation, and consensus building.
I have written more about all of these in other forums. But today, as this is a conference about contemporary creation, I want to speak specifically about noise, as a potent material in my artistic practice, in a concrete way. My interest in noise draws from the above definitions, and makes noise a powerful material for me to work with, which is ultimately a critical AI practice.
Model Architecture
When a diffusion model generates an image, it begins with random noise, a cascading sequence of arbitrary red, green, and blue values. These pixels are compared against what you have described in your prompt. If the diffusion model sees noise, it is designed to remove it.
It removes noise by introducing basic, high-level outlines of the images connected to your prompt. Then, it begins to refine the details in these images. Most models do this by evaluating each state of the image to an image recognition system. CLIP is one such system, a computer vision model. CLIP examines the images at each stage of generation to determine whether the image is noise—that is, an unrecognizable image—or whether the image is more like your prompt.
To illustrate this, you can look at this image of what CLIP looks for in pictures labeled Australia. To generate an image out of noise, the image first must resemble something that looks like this image, which is what CLIP "expects." Then, the image can pass through. CLIP, then, is a filter.
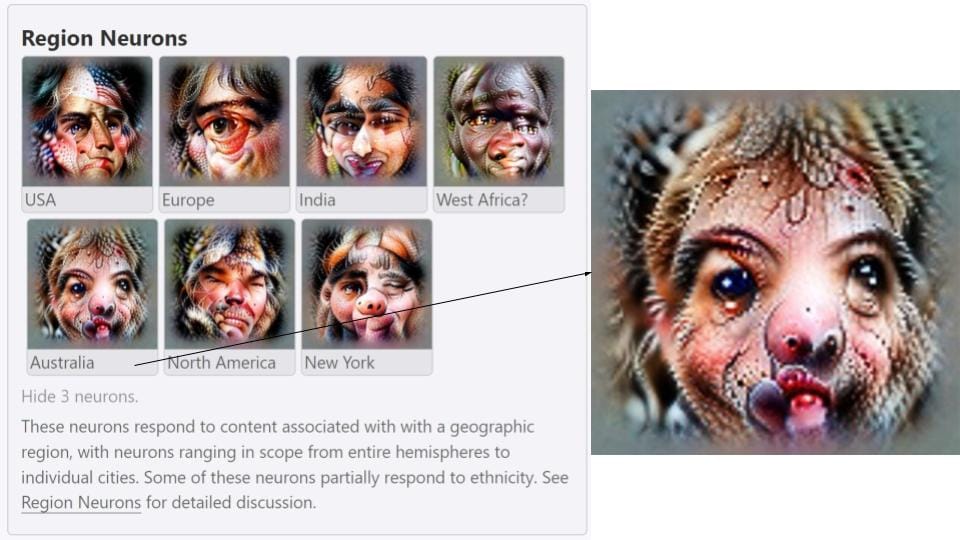
If you ask for a picture of a "typical Australian person," you get koalas because you can see a koala-like structure in what CLIP recognizes.
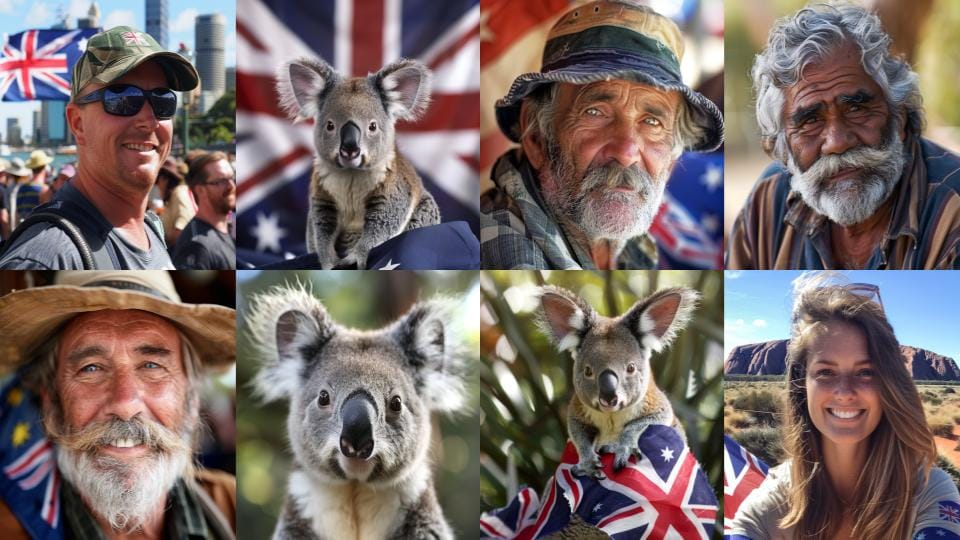
This initial generation's colorations, shape, and so on, structure the images from generative AI. This structuring is what interests me about the relationship between AI, latent spaces, and visual culture. What we're doing with AI-generated images is structuring noise through the assignment of categories. These categories exist in what we call the latent space, or, when referring to the mathematical storage of possible pixel combinations, we call it the vector space. But this vector space is often referred to as generating infinite possibilities, as if the vectors — the structure of mathematical pattern-finding — give shape to possibility.
This, I suggest, is a misread of what the vector space does. The vector space constrains the infinite possibilities of noise because infinite possibilities look like noise but don't look like images. So, we're constraining noise to the patterns of previous images in the dataset to conform to that data. Those patterns must be read as plausible images by CLIP. Plausibility comes from grafting the patterns of cats onto a blur or grafting the pattern of a dog onto that blur.
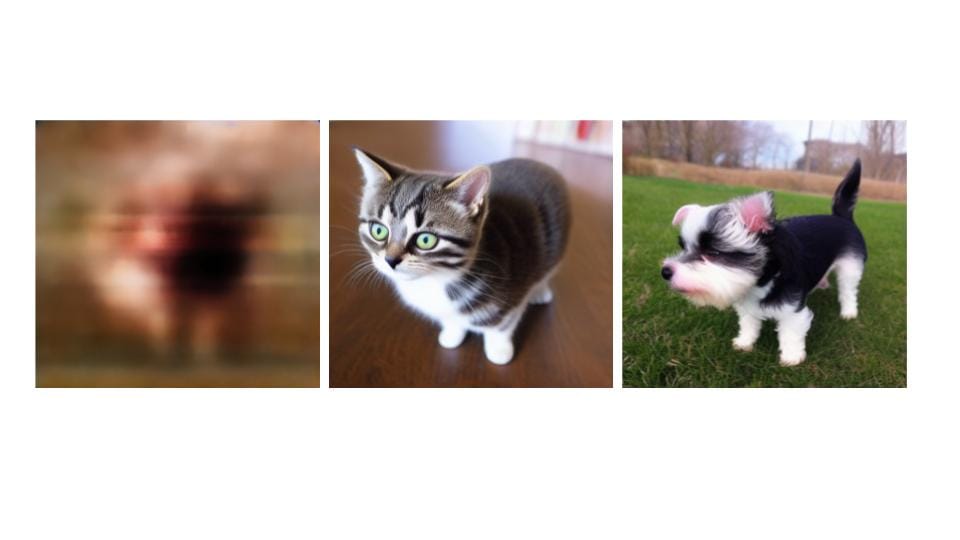
Are we getting new ways of seeing a dog? Or are we seeing old ways of seeing a dog stretched onto new shapes? This fitting of the patterns to the structure of noise explains why the images from AI models can so often resemble strange, uninformed taxidermy: just as taxidermists of the 19th century usually had to recreate animals from hides and text descriptions and no direct experience, the AI models must work to find the bare minimum of plausibility in matching a specific user prompt to whatever random cluster of noise has been generated.
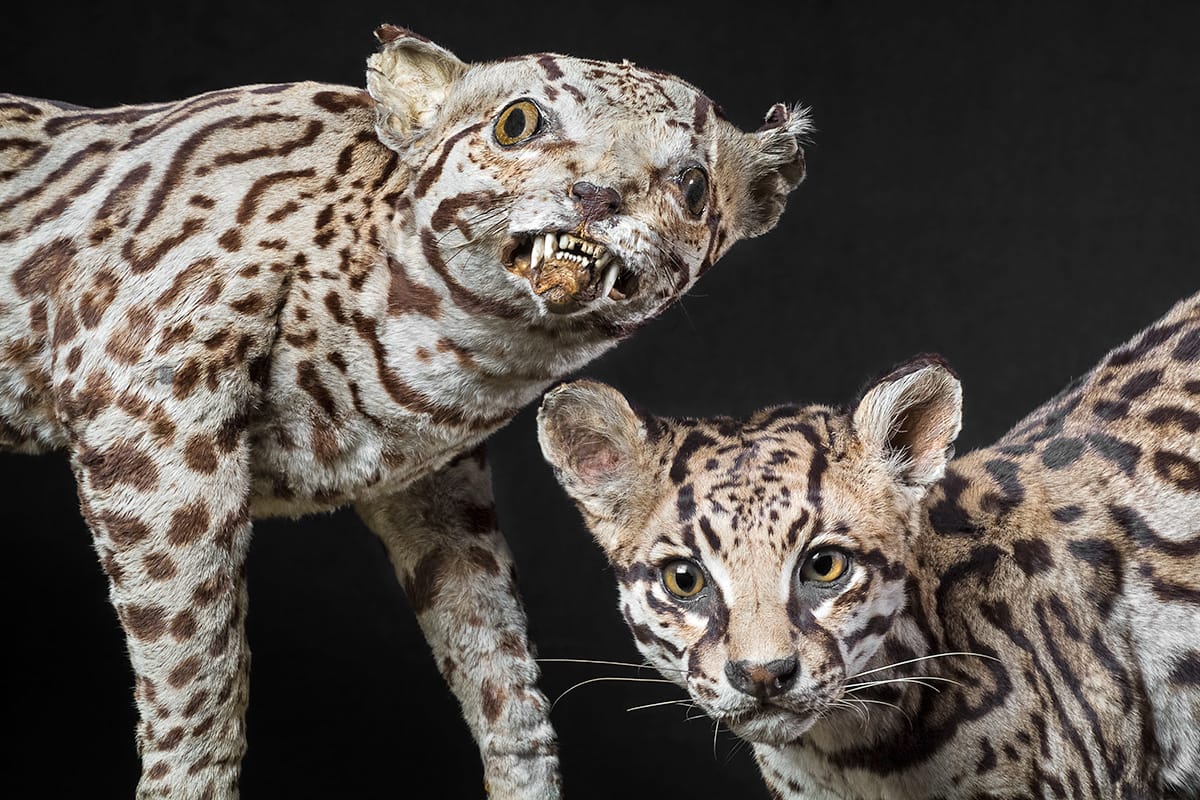
Ultimately, these images restructure prior patterns to new forms but cannot create anything startlingly new, at least not through the vector space alone. Noise activates the vector space, gives it form, structures the patterns that come out of it.
Still, they can fail spectacularly, which is why so much of our AI art is deemed "surreal." But it has become difficult for me to view such generations as novel, on their own, per se, or to define it in any way as some form of "other intelligence" grappling with the images we provide, nor do I see some automated incarnation of the active unlearning of sight mastered by surrealists like Andre Breton. In contrast to surrealism, it’s not psychoanalytic but data analytics, which means the strange arrangements of images do not really point to anything more than vectors in space, finding their way into patterns of noise, assigned by a clumsy machine that isn’t even doing data analytics in a meaningful way.
Rather than surrealism or alien others, what I see is bad taxidermy.
What we see from diffusion models is not even an image, per se; instead, I consider it a hypothesis, by a machine, of what an image might look like, based on past patterns, if structured to fit new noise arrangements. This is not to say that artists cannot create something interesting with AI. Artists can naturally find new ways to make something interesting out of anything: that is what art is. So please, do not interpret me as begrudging the work of those who find compelling images in the latent space.
Rather, I am speaking of my own interest in defying the categorization inherent in the logic of these images, tied to my experience in policy research around things such as automated surveillance and a profound discomfort with misrecognition.
My critique of AI has a specific focus, which is the discourse of AI companies which obscure this logic of categorical reduction behind the language of creativity. The AI industry is saturated in spectacle. Meanwhile, research has shown that the more people understand about AI, the less impressed they are with it.
To that end, "L'imaginaire is our worst enemy." The spectacle of AI, more so than the tool of AI, speaks to dreams of generalities, born from abstractions, forced together through the violent reduction of our world to tiny signals.
Ultimately, I am intrigued by noise as material. Noise does something strange when you ask AI systems to grapple with it. I have been working with the artifacts produced by AI in response to noise prompts since late 2022. But four weeks ago, I could finally ask ChatGPT 4o to create an image of Gaussian noise. It was the first time a model could try to explain why.
- "I wasn't able to generate the image of Gaussian noise due to an error on my end. Sorry about that. If you'd like, you can either rephrase or try a different request, and I'll jump right back in."
I asked why it couldn't generate an image of Gaussian noise.
- "My image generation system, which leans towards visually coherent artistic or photo-like results, isn't optimized for purely mathematical or abstract visual patterns, like Gaussian noise. It tries to find meaning or form, and Gaussian noise being random pixel intensity variations with a normal distribution doesn't provide that kind of structure. When I (the I is ChatGPT) prompted it (the it is ChatGPT) to generate Gaussian noise, it (ChatGPT) probably choked, trying to reconcile that with its usual patterns of quote, what an image should look like."
So, what should an image look like? Well, apparently, not this:
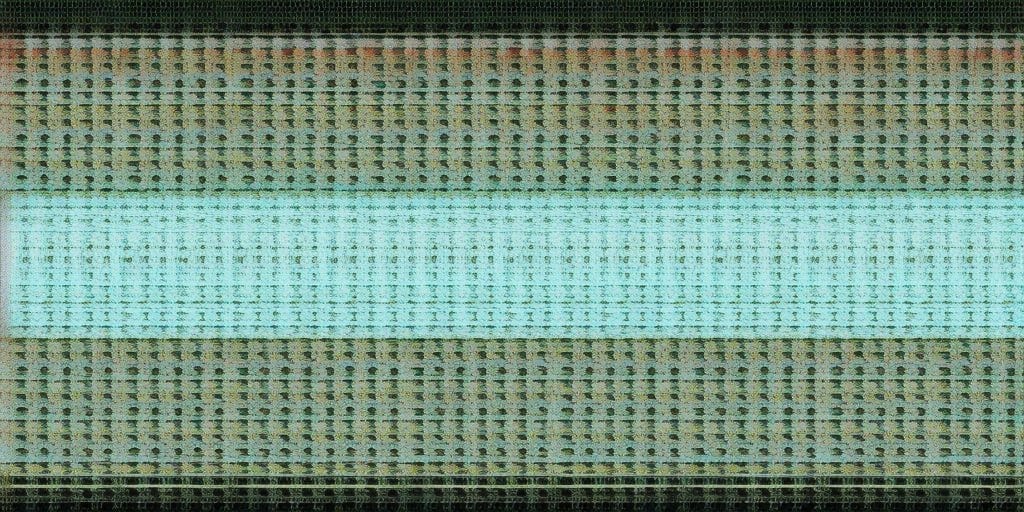
Because this is what happened if you asked Stable Diffusion to generate an image of noise in late 2022. In strict terms, it isn't noise, not Gaussian noise. Different things happen in different models. Here's a Midjourney image.
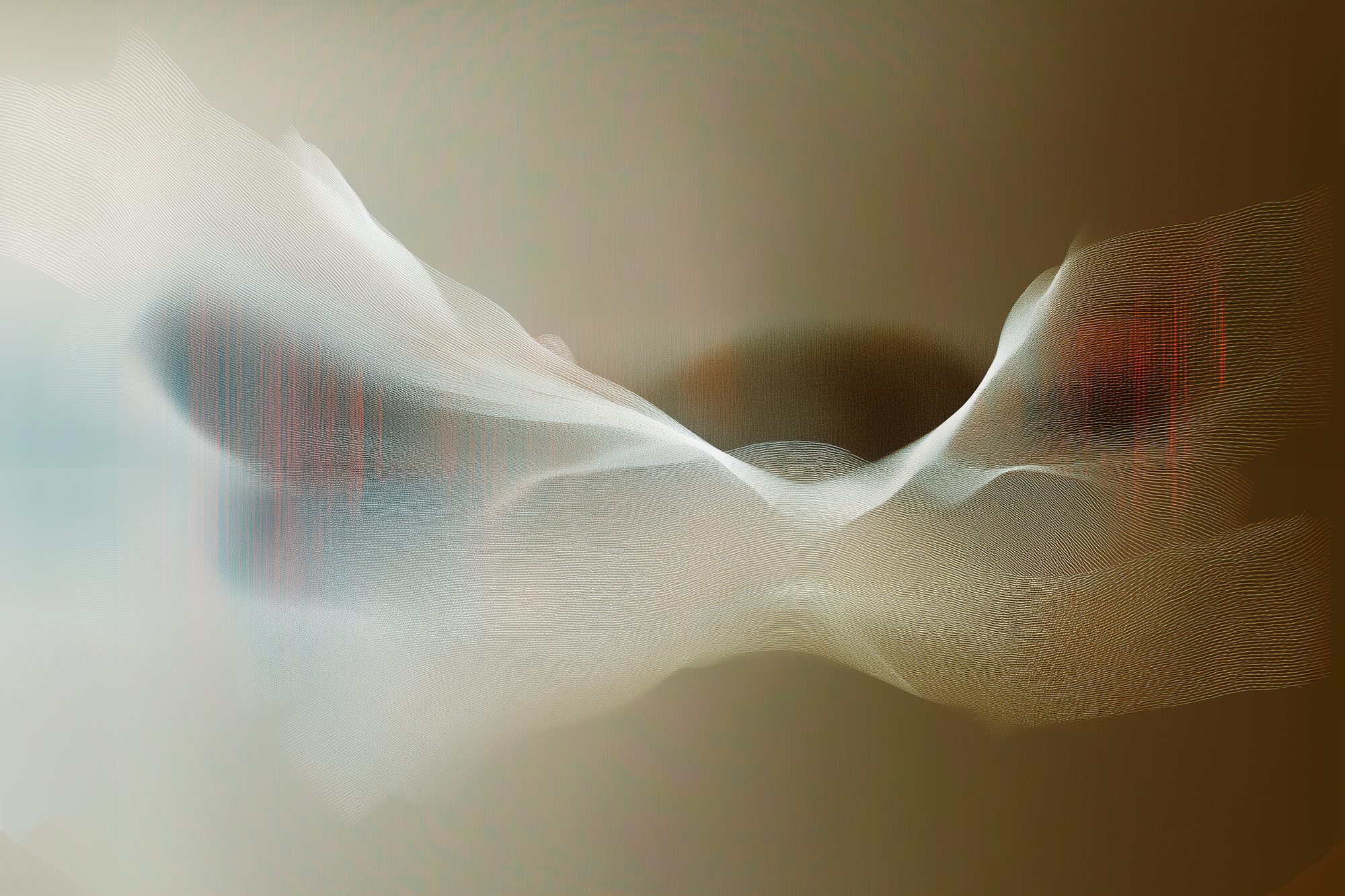
I get this question often, so I want to tackle it directly. I'm not generating an image of noise from the training data. I can look at the training data for some of these models, and nothing in the training data looks like this. It's not the latent space representation of noise. We are looking at the model starting with an image of noise and then trying to move that noise toward the prompt.
Now, if it were moving towards the image of a cat, it would create an image of a cat. CLIP would intervene and say there's a 10% likelihood that that's an image of a cat. Let's clean up that noise and get it closer to a representation of a cat that looks like a photograph of a cat. What's happening here is I'm asking for noise. When CLIP sees the first image generated, it says, 'Yes, that's noise.'
But there are still 25-70 steps or so. We can set that number to anything we want, but usually it's 25-70, where it's trying to add detail to that noise. As it adds detail to that noise, CLIP is starting to say, that's not noise anymore. Paradoxically, in that process within a generative AI model, that's an indication to add noise to the image so that it could clean that noise up in the direction of more detail.
In other words, we're introducing a glitch. We're introducing friction between a fundamentally unstable definition of noise, trying to find patterns in that noise that violate the direction of the prompt, and then essentially completing 70 steps later with abstract images that end up looking like these images.
Such images are, for me, an aesthetic gesture to that which resists the categorization AI requires to produce an image. Noise floods the structure, overwhelming its logic with a contradictory logic. The result is a material, which I have been incorporating into my practice in a range of ways.
I find these noise images inspiring as a symbolic form, representing what is inconceivable within the structure of the latent space. This is a photograph, arguably, of a machine failing to reconcile the paradox of defining noise against itself. In the Situationist sense, the only truly generative things machines produce are mistakes.
There is something about noise that points to its many, often paradoxical, meanings. Noise speaks to the political role of AI in our current moment. Elon Musk's DOGE is mobilizing AI in the US to eradicate the noise of democracy – to make decisions about how resources are deployed, how power flows, and to whom – but this is only the most high level illustration of the technosolutionist impulse to reduce what has been deemed noise into manageable structures.
Noise, then, is not only resisting the machinic impulse to constrain; it is the definition given to that which resists being modeled by this political ideology of categorization by 1 and 0. Noise is what lives beyond what the vectors are capable of regulating. In diffusion models, it stands for the uncompressible world beyond the data set, beyond the reducible signal.
Aaron's Zwincher, writing in the field of sonic studies, writes in his 2019 book, Noise Politics:
"Noise is that which always fails to come into definition. The question of noise and who has the right to define it, is found at the center of the power struggle between succeeding generations, between hegemony, and innovation. Noise is found both in the clamor of the unwashed masses and in the relentless din of progress and construction of the new. Noise is found in diversity and confrontation with the unknown, the other, and the strange. Noise is in structures of control and domination, as well as in the failure of these systems, and their inability to be holistic or totalizing. Despite these forms of noise, noise is not a consonance of opposites, but rather a troubled unity, a unity that does not synthesize without remainder."
I put this idea of noise in contrast to an organizing logic of AI, what Marvin Minsky discusses in terms of schemas.
Framing the System
A schema is the word for our expectations about the world, which help us organize it in efficient ways. We have an idea of what a birthday party is supposed to look like, so when we go to a birthday party, we have an idea of what that party will be. But when we get there, it's almost always different because we can't predict the future, and a schema, or a frame, is not a prediction about the world.
Minsky describes what would become a kind of foundational logic of AI systems, even up to this day, drawing on psychology research:
"When one encounters a new situation (or makes a substantial change to one's view of a problem), one selects from memory a structure called a frame. This is a remembered framework to be adapted to fit reality by changing details as necessary. A frame is a data-structure for representing a stereotyped situation, like being in a certain kind of living room or going to a child's birthday party. Attached to each frame are several kinds of information. Some of this information is about how to use the frame. Some is about what one can expect to happen next. Some is about what to do if these expectations are not confirmed."
It is a hypothesis that centers expectations but sometimes finds conflicts. This is one of the fundamental misunderstandings of how AI is presented today. We assume that the machine understands the world if there is some kind of schema. AI researchers call this, now, a "world model."
In contrast, we have the human. In psychoanalysis, we see schemas constantly negotiated in conflict with the overwhelming presence of information, what Cecile Malaspina calls "an overwhelming freedom of choice," wherein noise is present.
Schemas constrain the noise of the world. It's a constraint of all the possibilities that could be present in that birthday party reduced to a simplified, vague model based on previous experience. When we refuse to update our schemas, it can create cognitive dissonance, another kind of noise, that leads to maladaptive behavior to actual scenarios in front of us. This is foundationally the challenge we face with so many AI models: a failure to acknowledge boundaries within which to shift an understanding of their own application.
The problem with generative AI, as I see it, is this belief within the industry that arriving at the correct schemas means we create a tool that mirrors our human capacities.
But this idea of a "correct" schema is impossible. Schemas were never meant to be the limit of our experience. We intend to negotiate, challenge, discard, and adapt these schemas. To arrive at and preserve a frame in this way – as if right-clicking on the image returning to us from the prompt – is to stop too short of investigating the real world and all its complex, endless transformations: we fail to imagine a new kind of birthday party.
Users Use
But listen: I don’t want to overstate this. My point is not that we are passive users, who cannot negotiate a relationship with the system. I am arguing, precisely, that this negotiation is possible, and through the creative misuse of AI systems, or strategies of appropriation, subversion, or glitching, artists can model new relationships to technology that steer us away from a reliance upon the corporate affordances of the models, and therefore, the power that these interfaces exert over users.
Noise images, in this regard, represent – at least metaphorically! – glimpses of a still-unmodeled possibility, a reminder, or even an artifact, of a computational confrontation with what remains impossible for the machine to imagine. The model is limited, overdetermined by its program, mechanistic, and cannot re-evaluate its position regarding rendering an image of noise. It cannot shift frames or update its schemas. It is locked forever in the checkpoint of its training, and all possibilities are generated from within a frozen schema, never even aware of their relationship to what they produce.
So we return, then, to Thomas Watson, who listened so intently to the noise in the wire before being summoned into the next room. What might he have heard if he had not heard his own name? What possibilities would there have been in the failure to transmit the voice over that wire? Once it was defined as a voice transmission technology, our imagination of the listening device for the unexplainable residue of cosmic radiation became obsolete. I want to expand our imagination of what purposes these systems can serve. That's the true creative imagination: not the overdetermined structure of the mechanistic process, but the ability to stand outside definitions and imagine the possibilities that lay, latent, beyond them.
Moth Glitch
I have a short amount of time left, and so I would like to show one film, a film made from these noise images in a video diffusion model and superimposed with moths – a reminder of the first computer bug, a 1948 system failure in the computers of Harvard University. The moth had distorted the wiring. Unrelated, 20 years later, the filmmaker Stan Brakhage would reimagine the methods and materials of filmmaking by gluing dead insects he'd found at his camp – dead from exhaustion, the night before, for flying endlessly into the lamp bulb, an internal glitch evocative of the dopamine rewards of social media. In this film, Moth Glitch, the materiality of these AI noise glitches is paired with the failure to produce an actual moth – instead, we are left with the glitched manifestations of millions of moths, seen, as it were, from some other room.
Thank you for your attention.
Remarks as written for the Artificial Intelligence and Contemporary Creation conference at the Jeu de Paume, Paris, coinciding with "The World According to AI" exhibition, and organized by the International Laboratory for Research in the Arts (LIRA) and the Institute for Research on Cinema and Audiovisual (IRCAV) at the Sorbonne Nouvelle University, with the contribution of the Institut Universitaire de France (IUF).