In Defense of Human Senses
And/Or: Letting Machines be Machines
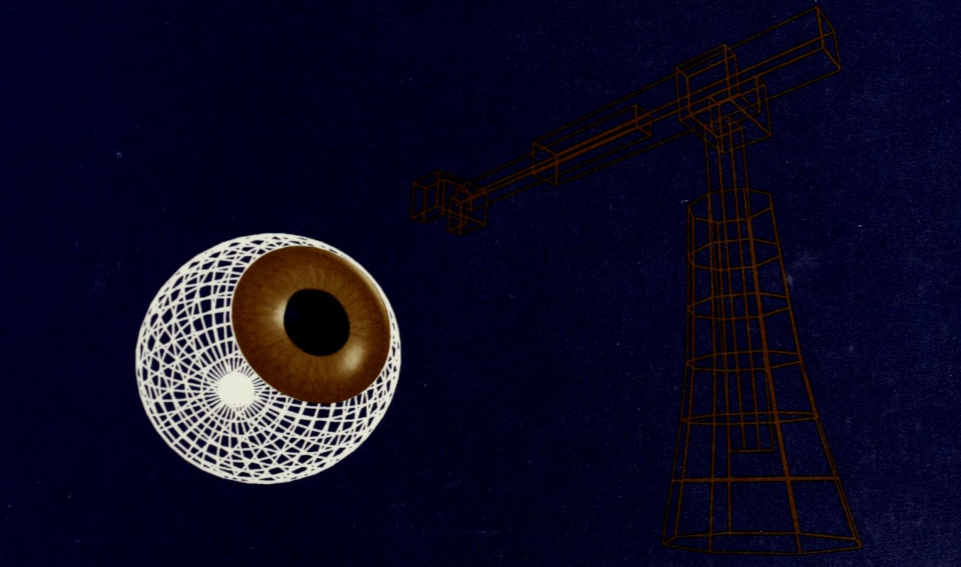
Does an AI see the way a human sees?
You’ll hear that they do. I’m told that AI systems see the world of images, and learn from them. That they create new images from what they have learned. I’m assured that this is precisely what human artists do.
At SXSW, I was asked if there was a difference between: a) a person going to look at the Getty images website and then taking photographs and b) a machine looking at the Getty images website and then making photographs.
It was a rhetorical question. The assumption is that there is no difference. But I think there is. I think reducing what artists or photographers do to what a machine does is tempting as shorthand. But ultimately, it obscures the actual mechanics of the machine, and it obscures the experienced reality of the artist.
Let’s start with a brief summary of what it means to be human.
What Humans Might See
Every encounter a human has with an image occurs within a context. We see images on billboards. We see them in museums. We see them online or on TV. We see them when we are on our way to an exciting date and when we are coming home from a disappointing one. We see pictures of our dog, and we see pictures of dogs we haven’t met. We see medical records in a TV drama and we see an X-ray of our uncle’s lungs inside a doctor’s office.
The experience of an image matters. It creates associations and feelings. It creates emotional responses. When images are juxtaposed, or placed in a sequence, we might read them as a story. The story of those images may be a projection of our associated experiences with similar images: it reminds us of something. We connect them to previous lived experiences. We interpret them based on their alignment to those experiences.
Are the people in the photograph meant to be us, or someone else? Is it meant to be seen by us, or by someone else? Who has crafted this image and what do they want me to do with it? Do I want to read this image in the way it was intended? Would I like to read it differently?
We don’t just encode and decode images. We use them to tell our own stories. Without those stories, images are data: latent, unactivated visual information. But because we activate these stories, we negotiate what these images mean against the meaning that was written into making them.
That is the individual interpretation of these images. Hall notes there is also a hegemonic understanding of the images: the ones shaped through frequent use and description by authorities, from the law to politics to media. These hegemonic understandings are naturalized: in the US, a red traffic light means “stop.” To function as a society, with a shared understanding of the world, this hegemonic reading matters. But we also naturalize it: we say “red means stop.”
Red, of course, has no “meaning” outside of how we’ve learned to read it.
We process images through our eyes and into our memories. But we also process images through our memories. We see emotionally. We see through lenses we’ve learned and unlearned. When artists “recreate” what they have seen, it’s been molded by those experiences. We might see an image, abstract it, and regenerate it in some new form. But there’s more than information in the mix.
30 Seconds and 30 Years
There’s an old saw about a designer who could sit down and design a brilliant logo in 30 seconds. Someone asked how they could do that so fast. Her response was that it didn’t take 30 seconds — it took 30 seconds and 30 years. In other words, it was her experience, the way she learned to see, that lead her to create the work so quickly.
Yes, AI generates images quickly. I don’t think that is a reason to discount it as art. What’s missing from this instantaneous generation of a picture is any lived experience. Humans bring that. And we may choose which images make sense to us through our own experience.
But that isn’t the AI’s experience, it’s ours.
I would even issue a caveat that this definition of experience is not in and of itself a requirement for art.
The avant-garde have played with methods of stripping emotion out, to make “objective” art, for decades. I am not suggesting that this is not art, or that an AI cannot make images that can become art. I am simply suggesting that an AI does not see the way we see.
Despite this easy option out of the “AI can’t be an artist” trap, most AI artists argue for the opposite. That AI art should be perceived as emotional, beautiful, profound, in explicitly human ways, for what these images depict, rather than any process explored by the artist who frames them in that way.
Art emerges in the process - even if that process is the thinking behind it. That doesn’t mean spending hours figuring out what your prompt should be. It means figuring out why your prompt should be.
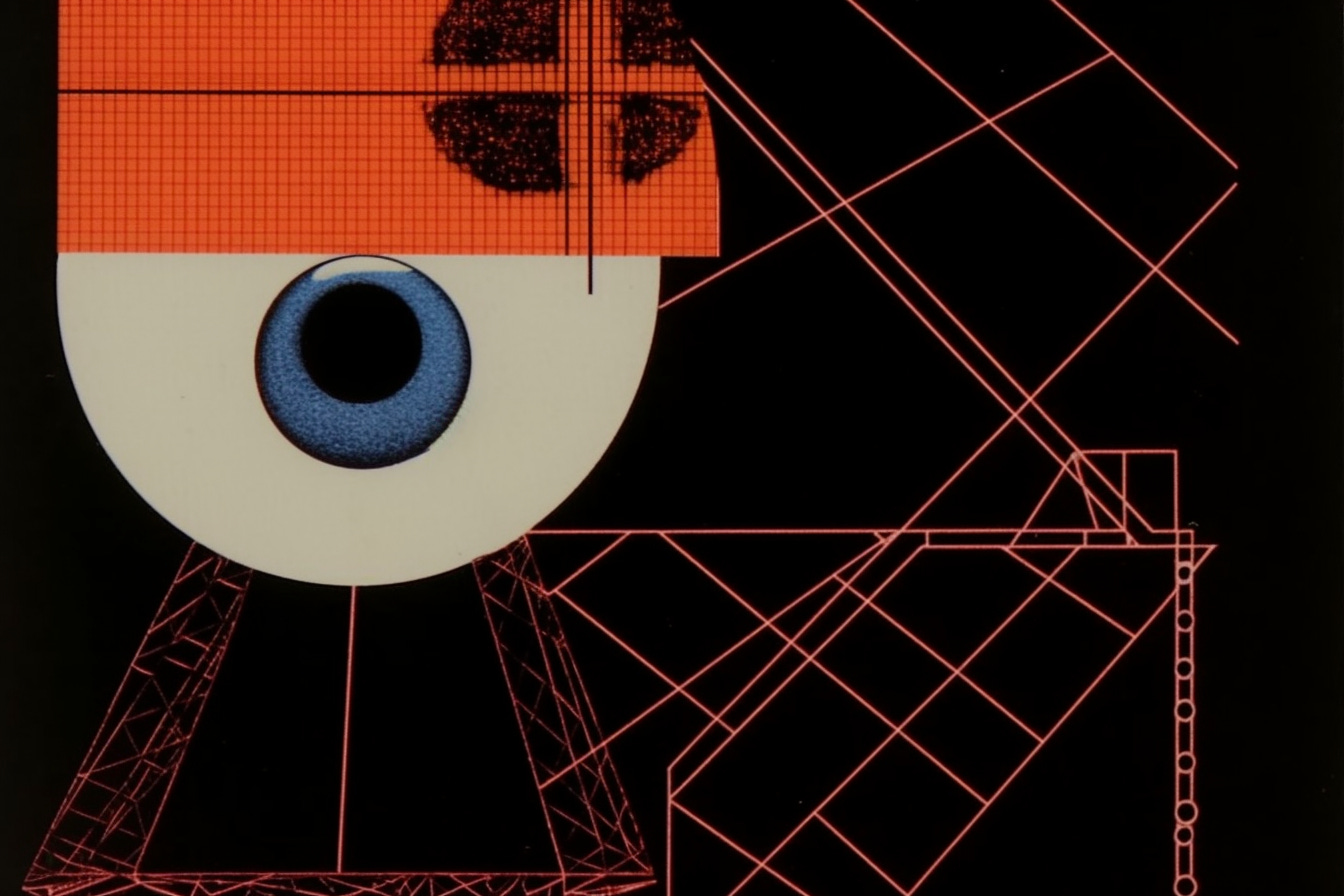
Negotiated Categories
Can we negotiate the meaning of an AI image?
I think we can, and that it is an opportunity for artists to move beyond the generation of images from the dataset — no matter how labor intensive their prompt work is, which is irrelevant to the question of art making!
One way is to negotiate with the images themselves. Because the images we get are hegemonic. This is evidenced by the fact that many claim to spend hours on their prompts. That means they are trying to find the language of category spaces created by the dataset. This isn’t a negotiation, it’s just trying to find the language of a dataset in order to reproduce its imagery. It’s trying to speak the language of a dataset to get what you want.
AI images are not collages of scraped materials, they’re collages of categories of scraped materials. There are contexts for images, but these contexts are stripped away. Instead of this social or historical context, they become categories: descriptions prized away from images, their captions shifting from descriptive to prescriptive. We no longer see an image and describe it with words, we write words and describe those words with an image.
To make a prompt into artistic expression, we ought to negotiate those categories. Ask the same questions we ask of the images we see on television and in our photo albums. We need to find ways to make the hegemonic categorization of images by an automated system into an individualized categorization.
To do that, we need to put conceptual work into the way we use these systems. It’s not enough to prompt certain categories into being. The categories themselves are the things we are making sense of and shaping images with. We can do things with categories, like collage or pastiche, even if the core images themselves aren’t legible in the final product.
Why does an image need to be made by an AI? Is it just because we couldn’t make it otherwise? Or is it saying something about our experience of these categories? How might we wrangle with and decenter the categorical logic of an AI system?
How Machines Might See
So far we’ve thought about how humans see images and negotiate those images.
How do machines see them?
Assuming that we are embodied, biological beings, this is already a complicated metaphor. Arguably, if humans saw images the way the AI sees images, we would be dissolving them with stomach acid in order to understand how the paper breaks down so that we could recreate it from a puddle of our own vomit.
Needless to say, this is not what you and I do when we are watching Succession.
But remember: A Diffusion system is built on a dataset, and that dataset is built from scraping the web. When it encounters an image-text pair, it strips information away from the image until it becomes pure noise. As the image breaks down, the model learns how it is breaking down, so that it can trace the noise back to its original image.
Once this is done, the resulting algorithm is assigned to the categories evoked by that image’s caption or alt-text description. A flower image breaks down, the model learns how, and this math joins into a vast set of abstractions associated with the word flower.
The Two Simplifications
The allure of saying that the machines see the way humans do is, I think, partly shaped by two simplifications.
The first is the simplification of how humans see. When we spend too much time coding complex problems into programs and algorithms, we begin to see everything as ultimately reducible. This is why AGI mythologies are so pervasive: if you are trained to see things as potentially reducible to a set of instructions for a machine to follow, you can be tempted to see all things as equally eligible.
The second simplification is a refusal to accept that there are, in fact, different ways to see. In other words: Diffusion models see in their own way. And despite my comparison to vomit, Diffusion systems are fascinating — poetic, even. They are able to see information break down to absolute obliteration, and then reverse it. They are able to move backward from a frame of noise into an image.
To apply the metaphor of human vision to this system is a tempting shorthand. But it’s also reductive — of humans, but also the system it describes. It is also, ironically, incredibly human-centered: closing us off to the ways of seeing beyond our own senses. It is possible to say that an AI does not think or feel, and to accept that we might find interesting new tools for our own imagination by contemplating what AI does instead.
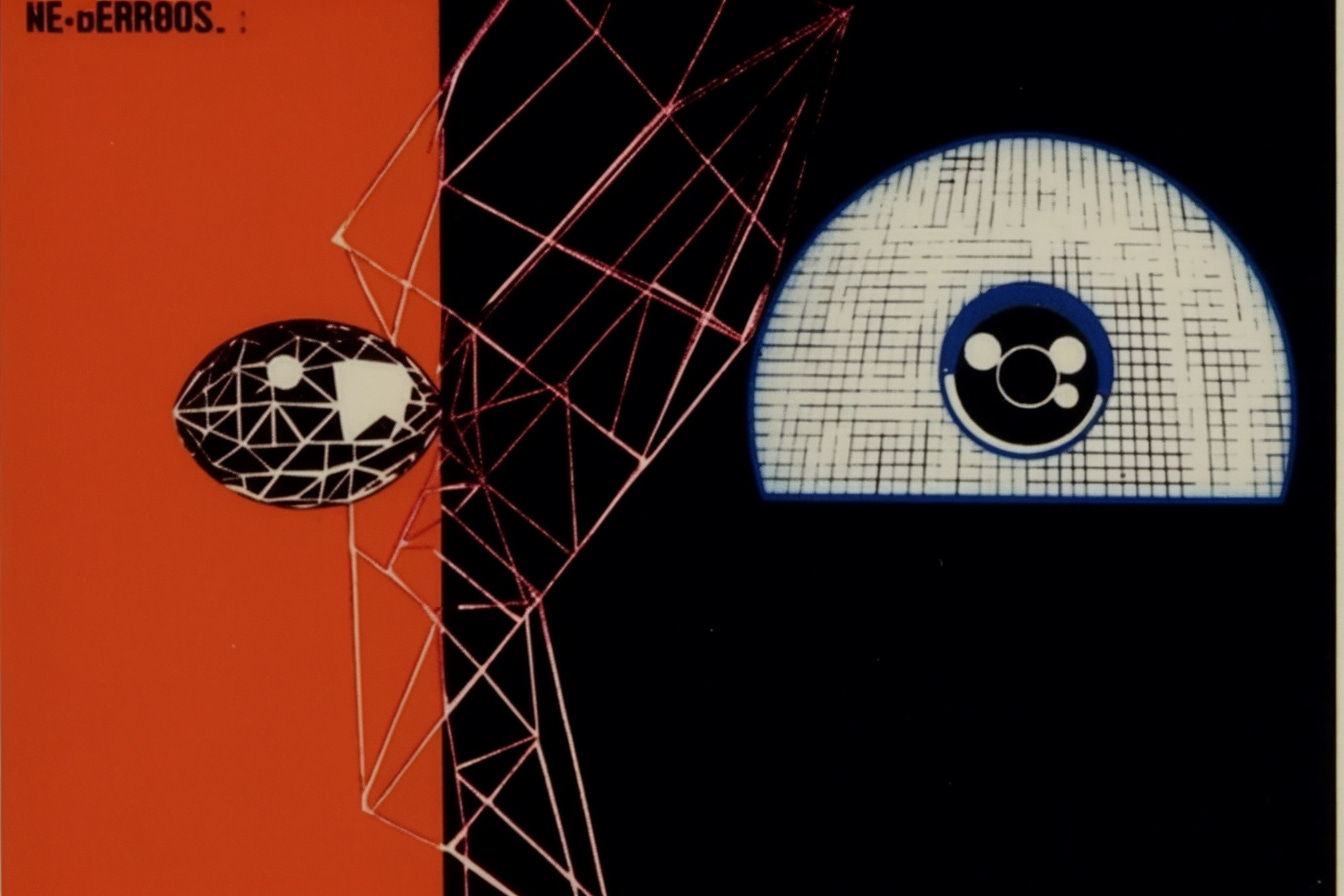
Let Machines Be Machines
It seems to me that when it comes to new technology, we should aim to see things as clearly as possible. The benefits of this clarity also skirt an inherent risk in the existing muddiness.
Seeing human vision as a mechanistic system is literally dehumanizing. It suggests that we are, in the slang of 4chan, “NPCs.” NPCs are video game characters that play out a script on a loop, there only for the sake of the one human player moving through the world. In the most abrasive use, this terminology suggests that one is the only thinking, feeling being in a world of people who are no more than machines.
The willingness to adopt the simplicity of NPC thinking is a way of simplifying all the messy entanglements of the world. We do not think like machines — we do not think like the LAION 5B dataset — and the LAION5B dataset does not “think.”
We would do well not to dehumanize humans. But perhaps we also could learn a lot by resisting the demechanization of machines. We might aim to understand the logics, structures, and patterns of the world through complex mechanized processes as part of the systems we’re entangled with, rather than a replacement for that entanglement. If we do, we might identify better strategies for managing their co-existence with social and ecological systems.
Things I’ve Been Doing This Week
LASER Talk at ROCO
On Friday I gave a Laser Talk at the Rochester Contemporary Art Center in Rochester, NY, part of Leonardo’s series of art and science talks. It’s about human decentered design and its role in making music, touching on my work with mushrooms as well as revisiting my work making music with an Eagle and three satellites. While there wasn’t a recording of the public talk, I did record my test run with my slides so I could share it with the world. Hope you dig it!
The Generative Workplace @ The University of Maine
I was part of a panel of presenters and a conversation about generative tools in the workplace, focusing on the issues generative AI is raising for creatives around representation and IP issues. You can find the video on a website through the button.
Thanks for reading! As always I appreciate your sharing these newsletters with folks who might appreciate it, or on your social media channels. I can be found on Twitter, Instagram, Mastodon and Bluesky (eryk.bsky.social) if you’d like to stay in touch.