It's Interesting Because
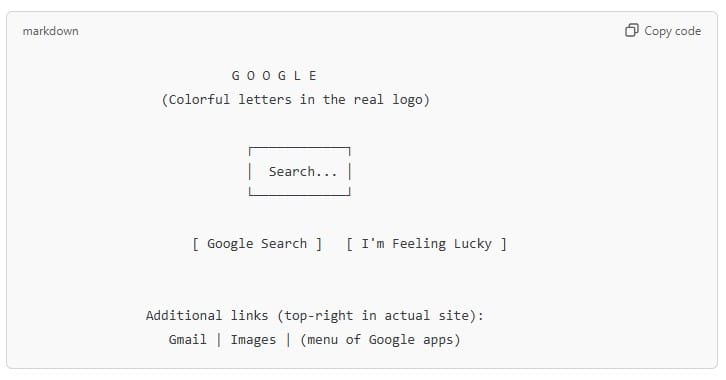
On NotebookLM, Fauxthority & Science Fiction
Whenever I talk positively about Gen AI, it means going very narrow, at the exclusion of a whole world of systemic problems about AI and the industry building it. Indeed, the problems with AI come from this narrow focus: designers look at the design, not the function; they fail to consider the possible social effects as a hypothetical "user" becomes millions of users.
But design matters, and design carries a set of explicit assumptions. Today, I wanted to look at something more or less exclusively through the narrow lens of design.
Years ago I wrote about design as a form of science fiction. Citing sci-fi critic Darko Suvin to analyze the object of Amazon's Alexa, I argued that just like science fiction is aimed at moving people from "estrangement to cognition," design has similar aims. The world of science fiction is estranged from our own, and the reader may quickly become disoriented. To make the world legible, science fiction authors must move the reader to understand the created science fiction world, and they do this through indirect reference to our own, more familiar, world.
I believe we can look at tech design as a work of science fiction, and apply a similar journey. A mess of circuitry and data and sensors and feedback mechanisms is disorienting. We're "estranged" from the reality it presents to us, as if entering the world of a science fiction story. So designers have to take that jumble and make it a story: a way for us to make sense of the world they imagine, where their product lives.
Science Fiction's estrangement serves a different purpose – in the best of it, the immersion into science fiction stories disorients us from our own world, because we have been able to imagine something else. Suvin writes:
Significant modern SF, with deeper and more lasting sources of enjoyment, also presupposes more complex and wider cognitions: it discusses primarily the political, psychological, and anthropological use and effect of knowledge, of philosophy of science, and the becoming of failure of new realities as a result of it.
In tech design, the same mechanisms lead us to understand the device, but then reinforces them as our models of "cognition" become part of the operation of the new machine. To expand beyond the narrow frame of use – to imagine other uses, possibilities, competing products or political paradigms – is contrary to the goals of most tech companies. They just want an easy reference you can use to make sense of the thing.
Consider Alexa, an object that required the user to speak to it. We can read this as a work of science fiction: the designers need us to see how it fits into the world we lived in before Alexa. They did this by designing it to be a responsive, feminine assistant, coded as both secretary and the shipboard computer from Star Trek.
But tech is often bad science fiction, in that it cuts off other possibilities and any critical insight into the material conditions that make Alexa possible – which are dense, and well represented in Crawford & Joler's "Anatomy of an AI System."
As a Large Language Model I Cannot
A similar movement happened with ChatGPT. The original text-generation systems were simply text extenders. To get people to understand how to use it, they had to give it a chat window. This moved the relationship with the technology from estrangement ("what is a transformer, and why do I care?") to cognition ("Oh, it's like direct messaging app.")
This decision made sense to users, but reinforced a specific understanding of what they were doing. The science fiction story that moved the user from estrangement to cognition also gave rise to false intuitions. For example, being put in the position of the person asking a question reinforced the idea that the model was authoritative. ChatGPT does not have authority. It only references statistically likely responses. As it moves us toward cognition about how to use it, it estranges us from the reality of what it is.
NotebookLM
NotebookLM is a Google service that is designed to summarize a small set of documents (20 or so) so a user can ask questions about them. It is a small folder for saving text, podcasts, and other media.
Text summarization is not a new landscape for generative AI. But what has made this product unique is its "Deep Dive" podcast simulations, with two non-existent hosts explaining the material to each other.
There are broader implications to this product – the way it uses data, the practices of its parent company (Google), etc – but for a moment I want to look strictly at UX design and podcasts as an interface for LLMs. Because NotebookLM tells us a different story to move us toward understanding what is, more or less, the same assemblage of "AI stuff," it highlights decisions about product design that are made elsewhere. That is, by looking at a weird outlier example of an LLM at work, we can better see what is possible – and think through why those other paths have been prioritized.
What it is
If this all sounds a bit like ChatGPT, you're right – that is fundamentally what it is. It has many of the same caveats: summarization can compress out a lot of meaning and nuance, and aren't always going to identify the things most humans – or the one human listening to the summary – would find most salient. In generating summarization podcasts of my own writing, I've found that NotebookLM often misses or misrepresents these points.
But the strength of NotebookLM's "Deep Dive" podcasts is that it dispenses with the illusion of authority. By positioning the conversation about your source material as a dialogue between two "hosts" with only a passive familiarity to the material, rather than two "experts" telling you what it says, NotebookLM diminishes its own "fauxthority" – though somewhat imperfectly.
Large Language Models are incapable of insight, but sometimes generate text that, when read and interpreted by a human, creates conditions through which that human might arrive at an insight.
Stripping away the content to a set of frequent associations, then re-articulating those associations with new language, estranges the reader from the source material. This does something different, depending on the context of use.
- As a writer, this type of estrangement from my own writing is helpful: it gets me out of my own head, hearing my thoughts rephrased back to me, and identifies opportunities for clarity.
- As a reader – when I am familiar with or have studied the material – the opportunity to engage with a conversation and fact-check it is a good way to reinforce my understanding of that material.
- As a student – when I am unfamiliar with the material – it, at best, helps me orient myself to a loose outline; at worst, it steers me in the completely wrong direction.
The irony of this – from a pedagogical perspective – is that the best use of LLMs comes from the understanding that they are unreliable, while the drive to use them in education often comes from the misrepresentation of these models as accurate sources of knowledge, shaping passive intake. In truth, the best learning opportunity for these things comes from active, critical resistance to them.
While Large Language Models are incapable of insight, they sometimes generate text that, when read and interpreted by a human, creates conditions through which that human might arrive at an insight. It’s an important distinction from the LLM arriving at and sharing an insight.
The incorrect way to use NotebookLM is to give it a bunch of papers you haven't read and have it make sense of them for you, which unfortunately seems to be the thing it was designed to do. Sometimes, the illusory hosts present the content of the material as their own ideas or opinions in weird soliloquies, and offer personal endorsements based on the arguments in the material.
LLMs are not Chatbots
Podcasts can create faux authority for human hosts – just look at Joe Rogan. But the UX direction of Deep Dive that I find promising is the way the hosts of the show present the material.
It is framed in a casual, chatty, "does this make any sense?" kind of frame. They sometimes draw conclusions that are wrong, or synthesize ideas from weird, disconnected pieces of the texts you provide rather than the more obvious connections.
But the presentations are always framed as the two "hosts" encountering this material for the first time. They are moving the user through the process of estrangement (a set of materials they don't understand) to cognition (being able to orient the user to a bigger picture).
The UX for this could have been awful. Imagine delivering wrong information in the compelling storytelling format of a TED Talk, or an engaging university lecture, or presenting the material as a panel of experts.
Imagine confidently delivering wrong information in the compelling storytelling format of a TED Talk. Instead, NotebookLM hosts have the occasionally annoying, uncertain cadence of National Public Radio banter.
Instead, the NotebookLM hosts have the occasionally annoying, uncertain cadence of National Public Radio banter. The verbal crutches of the DeepDive "hosts" are things like "It really makes you think about..." or "It raises questions about..." which is far more preferable than "this is what matters."
To that end, I think the designers took into account the reality of LLM unreliability and emphasized it through the medium and the way the model is steered: it is loosely presented as fallible. It is as if the listener could, at any moment, interrupt them to make a clarifying point to help the "hosts" make sense of it.
This is refreshing, because it reminds us that LLMs are not chatbots: LLMs are a model, and chatbots are the interface. All interfaces tell a story, and so far, the story we have been told – that LLMs are Internet Search, or that LLMs are a helpdesk agent on the other side of a chatbot – are flawed frames.
It Really Makes You Think About
NotebookLM's DeepDive has another twist in its design that points to other, really compelling shifts in the ways we deploy these things. This, too, builds on an understanding of the system as fallible. This is the decision to built the model around determining interestingness rather than factual accuracy.
Interestingness is defined by NotebookLM as a measure of difference between the sequence predicted by the LLM and the sequences contained in the text you have provided to it.
Interestingness is defined as the difference between the sequence predicted by the LLM and the sequences contained in the text you provide to it.
To make sense of this, let's look at what most LLMs do. They are text-prediction algorithms, and because the user is sharing specific resources, the model is able to compare its predictions of text (word by word, then phrase by phrase) to a mathematical model of the material that exists in a collection of text. The goal of most LLMs to represent this text plausibly: it is designed to generate a text that most closely resembles the text we are requesting based on these references.
NotebookLM does something extra. It generates the most plausible text, as an LLM would, but then compares it to the smaller collection of texts uploaded by the user. When the predicted output and the uploaded material differ, the model flags that difference and re-generates text about that difference to emphasize that difference. This is how engineers are quantifying "interestingness."
The Question That Always Works
This is fairly simple, but it fascinates me as an automation of podcasting and radio documentary. Noah Adams, the longtime host of All Things Considered on National Public Radio, once said that the "question that always works" is:
How did you think it was going to work out before it happened? And then, how did it really work out?
Noah's "Question That Always Works" has been cited by podcast and radio documentarian Ira Glass as well. It's a question that fundamentally directs us to uncover something surprising. It works like the structure of a joke: what you expect, then the unexpected. Sometimes the answers to this are funny, because of the gap between expectations and reality. Sometimes they are tragic. But at the end, this is the question that works because it always introduces a tension, and tension is the building block of an interesting story.
The synthetic hosts of the Deep Dive – consistently a man and a woman engaged in NPR-like banter – make the audio listenable. That NotebookLM is premised on this "one simple trick" makes it feel interesting. But it also means that the model has a sense that it is crafting a narrative – and if it has expectations, and identifies moments where those expectations diverge, it can highlight that disparity.
If you highlight the disparity between two narratives, you introduce something essential to knowledge, something missing from a search result or a chat response. You introduce a gap where uncertainty comes in. In the best stories, the reader confronts that uncertainty and has to weigh the evidence for themselves.
Expectation is a Bias
I'm curious, though, if this also introduces counterintuitive biases. For the model to highlight something as interesting, there has to be a difference between what is predicted by the model and what appears in the text. That introduces the chance that, when the text and machine prediction are aligned, they are not highlighted.
For example, one of my films about AI was played in a film festival last year. A local journalist hated it, writing something about "obligatory blathering about racist AI." The journalist expected there to be dialogue about racism in a film about AI, and was bored by it. So when the journalist encountered a discussion of racist AI, they found it uninteresting.
There's a risk that this happens with "interestingness" as a metric for LLMs, too: where exactly do the expectations of the machine come from? How well does its idea of surprise align with the users?
What Else Is an LLM?
UX shapes the way we understand LLM output. OpenAI's UX emphasizing making models look and sound authoritative, because they want it to look and sound like AGI. Compare the difference you get in products when you center "authority" as a value (ChatGPT) to when you center "surprise" as a value (Deep Dive). An auto-generated summary that says “look, I’m not expert; but maybe this is important?” makes a huge difference, and there is no reason not to build models that steer users toward this kind of critical relationship with their outputs.
Anthropic's Claude is designed to mimic curiosity and has an additional set of rules for "behaviors," but these emphasize the LLM as an agent in the conversation in human terms – one with a "warm relationship" with users, for example. Better still to acknowledge and emphasize the distance between what we know people imagine about these systems and what they actually are.
Which points to a possibility that we might have models that, through system prompts and design choices, not only undermine their own perceived authority but focus on facilitating critical thinking from its users.
Tech companies want to make authoritative-sounding knowledge replacers, but they could just as easily make models that point out their own epistemological standing and encourage users to think critically about the content they’re generating. Designers can build systems that emphasize where the knowledge comes from and how it has come to be presented in order to shatter the myth of self-awareness.
The reason they don't? Because all of the investment hype, and all of the attention, is driven by the paint job of AGI. OpenAI's imagination is constrained by a narrow focus on building models of human minds rather than building sensible tools. To justify Gen AI as a trillion-dollar investment, these companies need us to see a world in which current systems are deeply embedded into future infrastructure – where LLMs drive countless automated decisions that drive even more automated decisions.
Rather than fearing the effect of cascading hallucinations on that infrastructure, they need us to trust the model's decisions. Undermining the model's authority doesn't make sense if you want to build that world. You need a perception of superintelligence, of "reasoning," to defend that vision.
For that reason, I suspect we won't see AI companies building much more tech like NotebookLM, or even that NotebookLM will ever be what it might have been. I felt the need to point out the open door – even if I doubt anyone will go down that corridor.
Upcoming Events
I'm not participating, but if you missed or enjoyed the event on AI and Film at the Turing Institute some weeks ago, the organizer of that event has another event on at the London Short Film Festival in Soho (London) on January 20. It looks like an intriguing collection of films ranging in criticality, which should lead to some interesting conversations!
Thanks for reading! I recently migrated away from Substack. The new archive for this newsletter can be found here.
If you're looking to escape from X, I recommend Blue Sky. It's now at a level of polish that rivals Twitter's glory days. If you sign up through this link, you can immediately follow a list I've hand-assembled with 150 experts in critical thinking about AI. See you there!