On AI, Polling & Hallucinations
Statistics, Disinfo and Election Day.
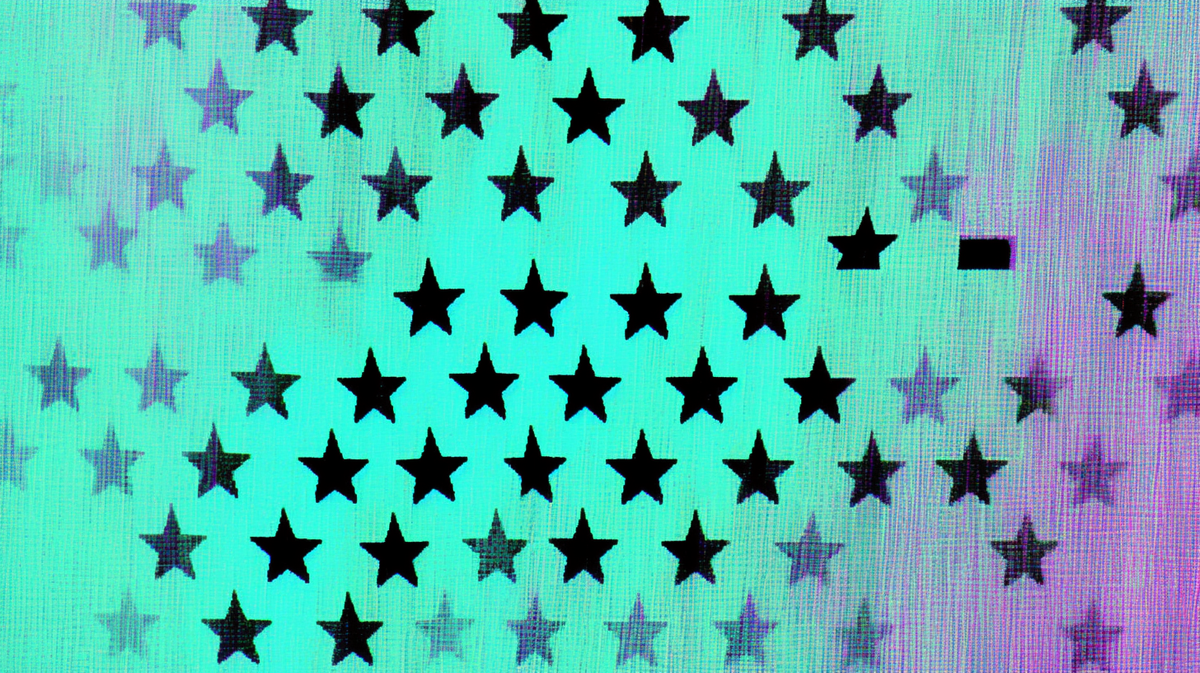
There’s a little political referendum called an election happening in America today, and with it comes some degree of anxiety about its outcome. One very likely outcome is that some polls will prove to be wrong, perhaps shockingly wrong. We also know that one party has become convinced that an election is about to be stolen and is likely to point to current polling data as evidence.
So, it seems worth discussing these polls and how they might be wrong before the election — and contextualizing what a poll is. While this newsletter focuses on artificial intelligence, there is a surprising overlap in the language and processes of polling and machine learning.
Both involve sampling, reducing the world into a sample, and its extrapolation into a prediction. Both are subject to human bias and errors during the data-gathering process. Both assume that scale doesn’t transform the rules set by the sample. And both are prone to miscalculation: a margin of error in election polling tells you the likelihood that a poll result is, in AI parlance, “a hallucination,” that is, an extrapolation from existing data applied onto non-existing data.
What is a model a model of? In this case, it can only model voters who pollsters contact. Everyone else in these models is a hypothesis, a prediction of voter behavior based on the available sample. When demographics are missing, then available data can be weighted so that it represents the broader population. But this is a distortion of the data, an extrapolation of a small number into a broader one.
It used to be that you could expect people would gravitate toward similar behaviors and that, with a large enough sample, you could capture enough to predict a pattern. The hypothesis of statistics holds up. The problem is that the models are only based on who we talk to — and talking to people has become much more complicated. Perhaps it’s the fragmentation of media. Or maybe it is that the only remaining undecided voters — people who look at Harris and Trump and aren’t sure which way they lean — are themselves decidedly eccentric outliers and, therefore, unpredictable.
The number that comes out of a poll is an average, and the margin of error tells you how far away an existing average might sit from that result. Surveys with 1,000 people may say there is a margin of error of 3 points, for example. If the survey shows that the candidate is winning 48% of voters, this margin of error suggests a range of nearly equal possible outcomes, registering 3 points above and below that 48%. This means a candidate with 48% support in a good poll, purely based on error rates baked into the nature of statistics, could be expected to register anything in the range of 45% to 51% support.
But this is only the baked-in variance that comes from polling. Other errors abound, one of which is whether or not your sampled population is truly representative of the population you are trying to model. If you apply a filter — deliberately or not — that excludes sections of the population, your polls reflect the population you have polled. They stray from an actual model of the population. One of the burdens of election polling, for example, is that the younger you are, the harder you are to reach: retirees are more likely to be at home, answering their phones and chatting with a pollster. Other biases in sample selection could include leaving messages in English for people who don’t speak English.
We also know that there are external pressures for pollsters not to get it wrong. Pollsters missed many pro-Trump voters in 2016, and many pro-Democratic voters in 2022. We know that they are often working to calibrate their models in order to adjust for past failures. This year, one of these methods is to assume that unlikely voters — that is, voters who did not vote in 2022 but did vote in 2020 — are more likely to vote for Donald Trump. The idea for this is that unlikely voters may turn out for Trump, but not turn out for some local politician. As a result of this hypothesis, many pre-election surveys are building models wherein unlikely voters are weighted more heavily as a Trump vote when they scale up the polls.
This may or may not be correct, though one issue with this, of course, is that unlikely voters are by definition less likely to actually turn up at a polling station. The only way to see if any hypothesis is correct is to wait and see the outcome of the actual tally. But past polling errors are, in fact, often greater than even the 3% margin of error. Errors not be universally applied, either: if there is significant state-level polling, polls in that state may not be similarly biased to national-level polling.
Pollsters also interpret models differently, and with the crisis in polling around Trump’s performance in past years, we’ve seen pollsters herd around similar results to one another and reject surveys that go astray from others in the field. In an uncertain race, this bends the polling closer to these tight 50-50 margins because the safest bet is simply not to make one.
In essence, polling is like any prediction: it’s always wrong because the event it aims to describe has not yet occurred. Harris and Trump sit at more or less 50-50 in the most critical swing states. It would not be remotely unusual if reality presents a 56% - 44% result toward either candidate. Particularly if Trump underperforms, it is worth flagging that the polling models have typically been designed to provide him with an edge in the hypothetical results — out of frustration from pollsters with finding his voters. For this model to be correct, it would need to mean that since 2020, Trump has not lost all that many voters, and Harris has not gained many more than Biden.
We simply don’t know which will be true. Your answer is more likely to reflect your personality, desired outcome, and even the political environment of your neighborhood than anything else. But if someone points to the polls to say the election has been rigged, or if you believe that either side could not have won without fraud, you must have more than the polls as evidence. Polls are predictions, and predictions never come true exactly as we expect.
This morning, it is hard to know if we’ll see a fast or slow count, if the results will be a blow-out—in either direction—or when and how people will propose that voter fraud has occurred. We know it’s pretty likely that they will. However, one thing I would not count as evidence of anything is the current state of the pollsters’ hypothesizing about outcomes. Every pollster worth their weight tells us that the polls aren’t telling us anything right now. Polls being close does not mean an election will be close.
On election night, we will start to see counts, many representing batches from specific counties and districts. They will come in and be added over time. In 2020, many say this as evidence of vote tampering. It is, literally, just what counting is. You count sections at a time, then bundle them up and report. The numbers may rise disproportionately depending on where these bundles come from — each bundle represents a geographical area, and geography is highly correlated with party behavior. We have some sense of how these areas will vote: it would not be surprising, for example, if a large batch of mail-in votes from a suburb of Philadelphia is overwhelmingly Democratic and features nearly 90% votes in that direction or that a large batch of votes from a rural town will go 90% R. Neither jump in the incoming vote count is evidence of manipulation; these are expected and normal.
Other avenues for misinformation have already been laid down. A recent video portraying a Haitian man bragging about casting multiple votes for Kamala Harris in multiple jurisdictions has been debunked. Assume anyone bragging on social media about committing crimes isn’t serious. This has little to do with AI: images can be paired with descriptions suggesting they represent nearly anything. Just because someone says a video portrays something doesn’t mean that’s what they describe.
With any luck, we won’t see violence, but if we do, it pays to withhold judgment on any videos that emerge from it. Reporting in the early moments of a crisis, especially a politically fraught one, is likely to contain mistakes and misreporting. Be careful of what you see and read that purports to explain what you see, and be especially mindful of what you circulate. Assume the simplest explanation for whatever comes your way: resist embracing conspiracy theories based on social media posts. Refuse to trust anything that three independent sources haven’t verified. Do your best to stop yourself from connecting imaginary dots.
Today — and tomorrow — could be a shitshow. It might also be wrapped up much quicker than we think.
The models are useless. We have to wait for reality to show us what it is. Try not to get lost in what it isn’t.