The Age of Noise
Video & Text from FACT 2024 at the Australian Centre for the Moving Image
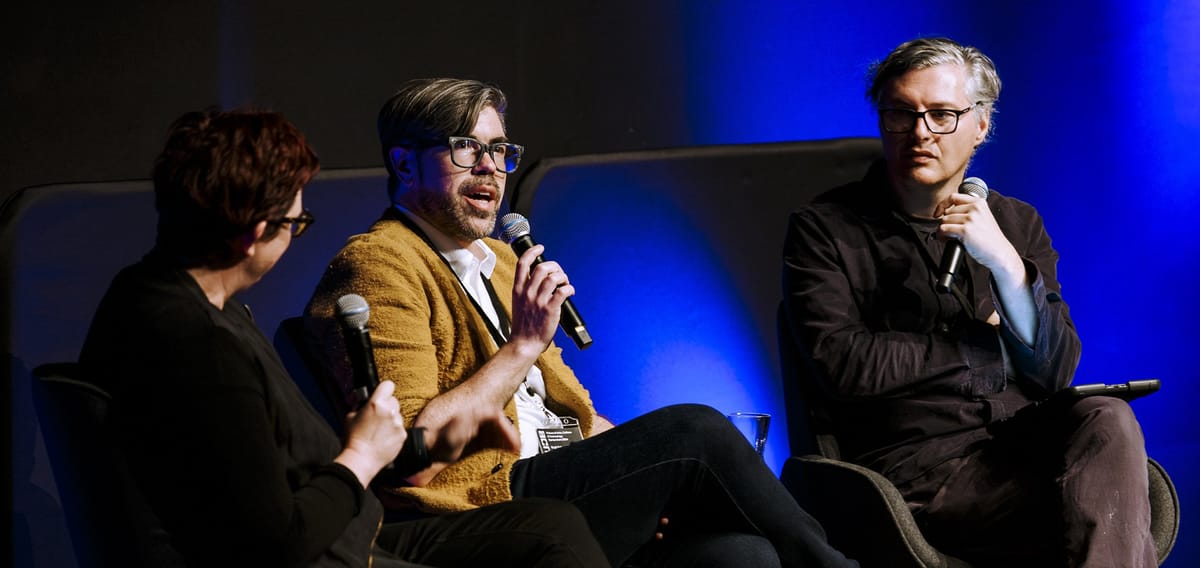
I’ve just returned home from Melbourne, Australia, where I was the first presenter at ACMI’s Future of Art, Culture & Technology (FACT) symposium. The panel, “Looking at the Machine,” also included Joel Stern (RMIT) and Katrina Sluis (ANU), focused on the questions:
What are the actual affordances, complexities and realities of artificial intelligence for the arts and cultural sectors? How do we ensure that creators are able to experiment and are not exploited? What does this mean for arts and cultural workers, who need to possess the technological and aesthetic understandings that ensure wise, forward thinking and make ethical choices?
You can watch the video above. My section runs about 30 minutes, and was prepared as a video lecture, so the visuals are important (more important than my accidentally emo hair style).
The Age of Noise
Noise is a slippery word. It means both the presence and absence of information. Today it's in the urbanisation of our world, the hum of traffic and jet engines. Noise is also where we go to escape noise. In August of 2023, Spotify announced that users had listened to 3 million hours of white noise recordings. Noise to sleep to, noise to drown out noise.
Noise is also the mental cacophony of data, on social media, of smartphones, and the algorithmic spectacle. The age of noise is a logical conclusion, a successful ending for the information age. And information, which was once scarce, is now spilling from the seams of our fibre optic cables and airwaves.
The information age is over. Now we enter the age of noise. We can pin the information age to the invention of the transistor in 1947. The transistor was quaint by today's standards, a mechanism for handling on-off signals. Engineers built pathways through which voltage flowed, directing and controlling that voltage in response to certain inputs. We would punch holes into cards and feed them to a machine, running light through the holes into sensors. The cards became a medium, a set of instructions written in the language of yes and no.
In other words, it all started with two decisions, yes or no, one or zero. The more we could feed the machine, the more the decisions the machine could make. Eventually it seemed the number of decisions began to encroach on our own. The machine said yes or no so that we didn't have to. By the start of the social media era, we were the ones responding to these holes. Like or don't like. Swipe left or swipe right.
It all began with that maze of circuitry. The first neural networks, our adding machines, the earliest computers, were designed to reveal information. Noise meant anything that crept into the circuits, and the history of computing is in part a history of noise reduction. The noise in our telephone wires and circuit boards, even our analog TV broadcasts, was background radiation. Energy pulsing invisibly in the air, lingering for millennia after the Big Bang exploded our universe into being. Our task was to remove any traces of it from our phone calls.
Today millions of on off calculations can take place in a single second. Put enough of these signals together, run them fast enough and you can do remarkably complex things with remarkable speed. Much of that has been harnessed into lighting up pixels. Put enough pixels together and you get a digital image. You get video games. You get live streams. You get maps, interfaces and you collect and process responses to live streams, maps and interfaces.
With what we call generative AI today, we obviously aren't using punch cards. Now we inscribe our ones and zeros into digital images. The data mining corporations behind social media platforms take these digital images and they feed them to massive neural nets and data centres. In substance, the difference between punch cards and today's computation is only that our holes are smaller. Every image that we take becomes a computer program. Every caption and every label becomes a point of information.
Today's generative AI models have learned from about 2.3 billion images with about 24 bits of information per pixel. All of them still at their core, a yes or no decision moving through a structure. I don't say this to give you a technical overview of image processing. I mention it because the entirety of human visual culture has a new name.
We used to call these collections archives or museum holdings or libraries. Today we call them data sets. This collected culture has been harnessed to do the work of analog punch cards. And these cards, these physical objects, were once stamped with a warning. Do not fold, spindle or mutilate. Our collected visual heritage in its digital form carries no such warning.
We don't feed our visual culture into a machine by hand anymore, and the number of decisions that we have automated are so large that even the words are ridiculous. Teraflops. We upload images to the internet, pictures of our birthday parties, our weddings, embarrassing nights at the club (not so much me anymore). Our drawings, our paintings, these personal images meant to communicate with others are clumped together with other archives.
Cultural institutions share a wealth of knowledge online for the sake of human education and the arts history and beyond. And in training an AI model, all of these images are diffused, a word that is so neatly parallel to this diffusion of unfiltered information that we surround ourselves with. And for once, it's a technology named in a way that describes what it actually does. Diffusion models actually diffuse! This word means what it says.
It dissolves the images, it strips information away from them until they resemble nothing but the fuzzy chaos of in between television channels. Images are diffused into noise. Billions of good and bad images all diffused into noise for the sake of training an artificial intelligence system that will produce a billion more images. From noise into noise, we move from the noise of billions of images taken from our noisy data-driven visual culture, isolate them and dissolve them into the literal noise of an empty JPEG, to be recreated again into the noise of one more meaningless image generated by AI among the noise of billions of other images, a count of images that already overwhelms any one person's desire to look at them.
The information age has ended and we have entered the age of noise.
We often think of noise as a presence. In America, we call it snow, the static. I've heard of other things as well. It's called ants in Thailand. Other places have other metaphors. But snow is a presence. We see snow. We see noise. We hear noise.
Noise from a communication engineering perspective is the absence of information. Sometimes that absence is the result of too much information, a slippery paradox. Information which cannot be meaningfully discerned is still noise. Information has been rushing at us for about two decades now, pushing out information in the frame of content to such an extent that almost no signal remains that is worth engaging with.
Here's a map of the internet visualised 20 years ago. Since then, it has only grown, today becoming a disorienting flood of good and bad information coming through the same channels. And what we are calling generative AI is the end result of a successful information age, which in just 24 years has rewritten all cultural norms about surveillance, public sharing, and our trust in corporatised collections of deeply personal data.
Server farms mined this data through regimes of surveillance and financialisation. The guiding principle of social media has always been to lure us into sharing more so that more data could be collected, sold, and analysed. They've calibrated the speed of that sharing to meet the time scales of data centres rather than human comprehension or our desire to communicate. And all this data has become the food for today's generative AI.
The words we shared built chat GPT, the images we shared built Stable Diffusion. Generative AI is just another word for surveillance capitalism. Taking our data with dubious consent and activating it through services it sells back to us. It is a visualisation of the way we organise things, a pretty picture version of the technologies that sorted and categorised us all along.
Instead of social media feeds or bank loans or police lineups, these algorithms manifest as uncanny images, disorienting mirrors of the world rendered by a machine that has no experience of that world. If these images are unsettling because they resemble nothing like the lives they claim to represent, it's because that is precisely what automated surveillance was always doing to us.
The internet was the Big Bang of the information era, and its noisy debris lingers within the Big Bang of generative AI. Famously, Open AI's chatbot stopped learning somewhere in April of 2021. That's when the bulk of its training was complete, and from there it was all just fine-tuning and calibration. Perhaps that marks the start of the age of noise, the age where streams of information blended into and overwhelmed one another in an indecipherable wall of static, so much information that truth and fiction dissolved into the same fuzz of background radiation.
I worry that the age of noise will mark the era where we turn to machines to mediate this media sphere on our behalf. It follows a simple logic. To manage artificial information, we turn to artificial intelligence. But I have some questions.
What are the strategies of artificial intelligence? The information management strategies that are responsible for the current regime of AI can be reduced to two, abstraction and prediction. We collect endless data about the past, abstract it into loose categories and labels, and then we draw from that data to make predictions. We ask the AI to tell us what the future will look like, what the next image might look like, what the next text might read like.
It's all based on these abstractions of the data about the past. This used to be the role of archivists. Archivists used to be the custodians of the past, and archives and curators, facing limited resources of space and time, often pruned what would be preserved. And this shaped the archives. The subjects of these archives adapt themselves to the spaces we make for them. Just as mold grows in the lightest part of a certain film, history is what survives the contours we make for it.
We can't save everything. But what history do we lose based on the size of our shelves? These are a series of subjective, institutionalised decisions made by individuals within the context of their positions and biases and privileges and ignorances. The funding mandates, the space, and the time. (No offence!)
Humans never presided over a golden age of inclusivity, but at least the decisions were there on display. The archive provided its own evidence of its gaps. What was included was there, and what was excluded was absent. And those absences could be challenged. Humans could be confronted. Advocates could speak out.
I'm reminded of my work with Wikipedia, simultaneously overwhelmed with biographies of men, but also host to a remarkable effort by volunteers to organise and produce biographies of women. When humans are in the loop, humans can intervene in the loop.
Today, those decisions are made by pulsing flops. One of the largest data sets we have is a collection of 2.3 billion images, LAION-5B. It is the backbone of training most open source image generation models and likely most proprietary models. It is a scrape of the common crawl index of the web, and its curation was done by a machine learning tool called Clip.
Clip's assessment criteria was simple enough. Using machine vision, compare the assortment of pixels in an image to the text in its caption. If the clusters of pixels looked like others that shared words in those captions, call it a match and include it. If it looked like a duck and it's labeled a duck, it's a duck. That was the end of the curatorial intervention into the data set behind generative AI.
People seemed to think that humans were involved in curating this collection. They were not. Instead, a group of volunteers created a tool to collect these images, made decisions about what that tool would do, which I just described, and then they deployed it.
These were the folks with decent enough intentions, by the way. They wanted to build a data set that people could look at and understand and evaluate. The data was out there online, and when you look at online culture exclusively through the lens of data to be analysed, it's not hard to see why they would grab all they could. Nobody looked at the result. How could they? It's 2.3 billion images. But the data set was collected, it was put online, and then used as training data for image synthesis.
Of course, humans were involved in this data set's curation in an indirect way. These images were the noise that defined the tail end of that information age. It is online culture. It included samples of nearly every genre of visual evidence, memes and pictures of our pets and children and our drawings, but also photographs of Holocaust victims, of Nazis on holiday in France, images of comic books and pornography, Taylor Swift and Abu Ghraib.
The Stanford Internet Observatory noted that the data set contained up to 3,000 images of child sexual abuse. The researcher Gary Marcus found that it contained countless examples of SpongeBob SquarePants and The Joker and other copyrighted material, while the researcher Abiba Berhane has counted a long list of racist, misogynistic and violent content in the data set. Given what we know of internet culture, this should be no surprise.
The information age's relentless spread of images were all reduced into one very challenging stew. And from this noise came a promise, a seductive but dangerous promise. That is the promise of new possibilities, a paradigm shift.
AI's integration of noise is not just on a metaphorical level. Noise is inscribed into these systems socially, culturally and technically. These images are diffused, dissolved into static and the machine learns how that static moves as the image is degraded. It can walk that diffusion backward to the source image — and this is how it learns to abstract features from text into generated images. Our training data constrains what is possible to the central tendencies in that training set, the constellations of pixels most likely to match the text in the description.
In other words, it navigates averages, trading meaning for the mean. But the original context is irretrievable. It reminds me of what Don DeLillo writes in White Noise — that “the world is full of abandoned meanings.” Meanings emerge from relationships. Words in the dictionary don't tell a story until they are arranged in certain relationships. This is why memory matters.
There is something disturbing to me about reducing all of history to a flat field from which to generate a new future. There are echoes of colonialism there. To take history, erase it and rewrite it in the language of new potentials, opportunities, prosperity, without deferring to those who built its foundations.
AI offers us a possibility of what? Opportunities for who? Prosperity for which people? Who gets to build a fresh start on stolen intellectual property? Who gets to pretend that the past hasn't shaped the present? Who has the right to abandon the meaning of their images?
Of course, all of these images still exist. Nothing is eradicated. Nothing gets destroyed. But neither is anything honoured or elevated or valued. Nothing in the training data holds any more meaning than anything else. It's all noise. Images of victims and perpetrators fuse together for our enjoyment. As noise, tiny traces of trauma inscribed for the sake of seeing something to post online.
But I want an imagination that moves us towards a resolution of the traumas of the past rather than simply erasing them. The AI image is not new in the sense that it creates something. Rather than new, the static is random, it is old patterns adapted to random noise. That's distinct from newness. It is more true to say that the image is wrong. It is a hypothesis of an image that might exist in the static, based on all that has come before.
The image that emerges is also noise, constrained by language and data. It references language and data to find clusters in that static. It is a prediction of what the image might be, a hypothetical image, constraining every possible image through the filter of our prompts.
And all of these abstractions are wrong. No image made by image synthesis is true to the world, but every image is true to the data that informs it. It would be lovely to think of AI as creating something new. The age of noise offers us only a false reprieve from the information onslaught. This is not merely imaginary, though the imagination is what we are fighting for. The images themselves contain discernible traces of our past. The data that constrains that noise is shaped by racism, pornography, stolen content, images of war and abuse. It is shaped by the way we label our images online.
The training data for the prompt girls contains thousands of images of white children from Victorian era portraits. The training data for black girls depicts sexualised adult women, including explicit pornography, which I have censored here as black boxes. Some companies are trying to navigate this by inserting diversifying words into our prompts without telling us, which solves the problem through the interface, but the models still produce stereotypes.
British people are almost always elderly white men. So are doctors. So are professors. So are a lot of things. Mexican men are typically depicted with sombreros in Midjourney. The training data for these bodies informs that outcome, and they weigh on the representations of their outputs. This diversity of human bodies cannot really make it through a machine that constrains images to their composites. In the white noise of AI, we are all fused together into one and sorted by the weight of the most common.
Today, all of these training data sets are offline. We can't look at them. We can't analyse them or figure out what's in them, figure out the biases that are shaping the images that come from these products. We can no longer examine them for their traces. We can't study their genealogies. The AI image is dressed up as dreams or imaginations of machines, but few people dream of their own overt sexualization or dehumanisation into racist caricature.
Humans have biases, but humans also have a consciousness. We work to raise the consciousness of people. You cannot raise the consciousness of a machine. So we must raise the consciousness of those who designed them. We must intervene in the shape of the data sets, and we must propose new strategies beyond reduction and prediction to counter the hostility of building a future from the reduction of history into an infinite automated remix.
That is not to say we should dispense with the past. Far from it. The idea of the remix is that we choose elements to arrange, and we move that culture forward with thought, arrange the pieces to the moment. The remix is not random pastiche. It is a thoughtful engagement. AI images are not a remix. They are the constraint of random noise through prediction. They don't engage the past or understand it. They reanimate it like a seance, but then they lie about what the ghosts tell us.
The shapes of these models sketch into noise are constrained by the shapes of the past. I liken this to a haunting, and I know that Mark Fisher has described the phenomenon of sonic hauntology, a music that referenced the past visions of the future, a future that seems to have been canceled. It reflects an inability to dream of the future on any other terms but nostalgia. And I would say that AI images are hauntological. The structures of our collective past shape them.
This is true not only for the prediction of images, but for any system which relies on previous data to predict future outcomes. If we look to the census, that starting point of data collection efforts, what we see in the data is marred by what could not be written into it. The data sets are haunted by what they did not measure, children who were not born because mixed-race couples were forbidden, home ownership records that don't exist because black families could not buy the home.
Data contains only the trajectories of history that have been allowed to us up to now. If we feed that information in the service of future decision-making, then these ghosts become police and the living become sorted by the dead.
Noise is the residue of the Big Bang. Noise is where the past lingers. Noise is where the ghosts are. It's the absences that haunt us, and we ignore history at our peril. When we talk about data and generative AI models, we are talking about images. When we talk about data sets, we are talking about vast collections of images. It would not be a mistake to say that a vast collection of images is an archive. But we don't say archives in AI. We say data sets.
An archive, I think, proposes a greater degree of responsibility. Archives are curated. Collections rely on humans to examine and assess images. Archives contextualise while data sets strip context away. Archives find relationships between people, places, and things. These data sets link objects only to resemblances of shape and colour. The data set is an archive diffused, and these tensions are the subject of great fascination to me.
In my work, I think about the age of noise and how it has been constrained by the age of information. I use found footage and archival images as a way of thinking through these tensions, placing the archive in dialogue with the noise that AI uses to make sense of it. In my work with AI, I've begun to think through this diffusion, selecting elements of visual archives and placing them into a tension site — marked by generative AI models that I've tricked into circumventing trading data, generating abstract patterns.
Ideas like this allow me a way to escape the contempt of data-driven abstraction and to pursue some version of uncharted possibility from inside this tension of static and definition. I want to see if new languages emerge. I want to know what the avant-garde can be in an age when noise is mainstream. I'm visualising this liminal space between archive and datafication, between home movies and prompt injections.
I don't know if I'm resisting or embracing AI by hanging out in this space, by thinking like a diffusion model, but I'm working alongside it and within it in a bid to understand it as best I can. In 2023, I was invited to present some of my methods for hacking AI art systems, the largest hacker convention in the world, DEFCON 31, as part of a, surprisingly, White House-backed AI Village. (I did not know that Joe Biden would be involved in a hacker convention when I said yes).
I was able to share and learn some strategies to make work that the tools weren't designed to make, most notably asking it to generate images of noise. It actually can't do that. It's not the way they're designed. They have to strip noise away from the start point of noise in the direction of a prompt. So if you ask it to give you noise, it's constantly trying to remove noise, and it gets stuck in a feedback loop that generates these kind of abstractions. How ironic that these machines literally cannot make noise!
I find these beautiful and elegant as a result of that messy contradiction. Not beautiful in the usual sense that we evaluate an AI image. There's none of that pristine lighting or supermodel faces from composites of all supermodels and all lighting techniques.
Rather, they're the residue of a system that has been set adrift without data about the past. It's a rendering of an image from a machine that has been told, “you don't actually know anything!” SWIM is a work from this year that I created while trying to visualise all of these ideas. Of information from the archive dissolved while shaping the future, the past stretched out endlessly into the present until it is perceived as something new.
In SWIM, a swimmer, from archival footage, frolics in a pool, now transformed into animated frames of an AI-generated glitch. Over the course of nine minutes, the footage is dissolved, as is all training data, to be quantified and linked to words. Swim is a description and a prompt.
The archives used to be where history went. Now it is where the future is made. Here I've blended the resulting noise with a real body, a body from the archives, the image of a swimmer. I'm well aware to the presence of the male gaze in this footage. The swimmer is a body being analysed, studied, traced, and this film was labeled in the archive as a form of erotic entertainment. The male gaze moves into automated form, enacting all the leering but at a remove. The analysis of the body in the archive is what makes deepfake pornography possible. If we want to look at bodies and AI, we look at them through the historical gaze, itself a masculine gaze.
And yet, this swimmer in the midst of this surveillance, in the midst of being dissolved, in the midst of being translated into media, strikes me, quite simply, as enjoying herself. I don't know, of course, but a part of me longs for that weightlessness, that ability to move freely while submersed in what might, in any other context, drown us.
This work is a critique of AI, but it's also a hopeful refusal of my worst fears about these systems and their relationship to creativity. That is, that our techno-social future reflects contemporary AI's techno-cultural forms. We can use these tools to push back against their logic, to reveal what is concealed by the interfaces and the language and myths of AI, to surface what swims in the archive, beneath the prompt, in all its messy, contradictory human complexity.
I'm often asked if I fear that AI will replace human creativity, and I don't remotely understand the question. Creativity is where agency rises, and as our agency is questioned, it is more important than ever to reclaim it, through creativity, not adaptability. Not contorting ourselves to machines, but agency — contorting the machines to us.
I fear that we will automate our decisions and leave out variations of past patterns based on the false belief that only repetition is possible. Of course, my work is also a remix. It has a lineage. To Nam June Paik, who famously quipped, “I use technology in order to hate it properly.” And this is part of the tension, the contradictions that we're all grappling with. I'm trying to explore the world between archive and training data, between the meaningful acknowledgement of the past and the meaningless reanimation of the past through quantification.
Archives are far more than just data points. We're using people's personal stories and difficult experiences for this. There's a beauty of lives lived and the horrors, too. Training images are more than data. There is more to our archives than the clusters of light-coloured pixels. Our symbols and words have meaning because of their context in collective memory. When we remove that, they lose their connection to culture.
If we strip meaning from the archive, we have a meaningless archive. We have five billion pieces of information that lack real-world connections. Five billion points of noise. Rather than drifting into the mindset of data brokers, it is critical that we as artists, as curators, as policymakers approach the role of AI in the humanities from a position of the archivist, historian, humanitarian, and storyteller. That is, to resist the demand that we all become engineers and that all history is data science.
We need to see knowledge as a collective project, to push for more people to be involved, not less, to insist that meaning and context matters, and to preserve and contest those contexts in all their complexity.
If artificial intelligence strips away context, human intelligence will find meaning. If AI plots patterns, humans must find stories. If AI reduces and isolates, humans must find ways to connect and to flourish. There is a trajectory for humanity that rests beyond technology. We are not asleep in the halls of the archive, dreaming of the past. Let's not place human agency into the dark, responsive corners.
The challenge of this age of noise is to find and preserve meaning. The antidote to chaos is not enforcing more control. It's elevating context. Fill in the gaps and give the ghosts some peace.
Thanks.
You can watch the video (and video piece) as well as tightly related presentation by Dr. Katrina Sluis, which followed mine immediately, and a Q&A at ACMI’s archive of the talk. Or check out other talks from the two-day symposium. Much thanks to Seb Chan, Joel Stern and Rachel Bugg for their excellent input and support in this conference and during my time in Melbourne!