The Guild of St. Luke
Reassessing Digital-Cultural Infrastructures
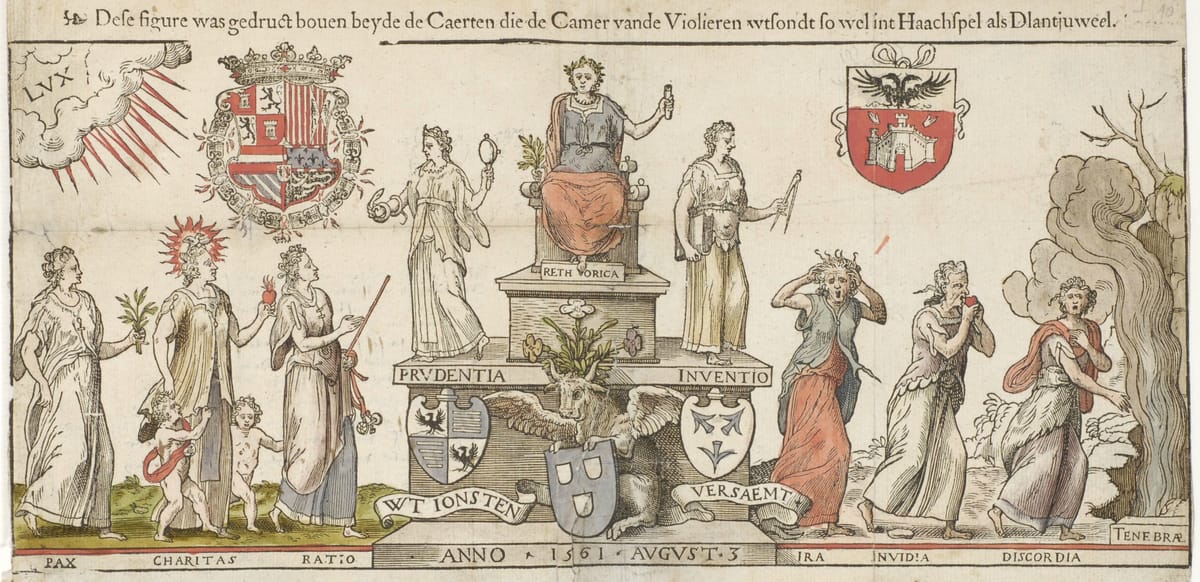
I don’t know any artists who learned to make art by looking at art. Folks learned by doing: building something, making something, whether it was on a canvas or Photoshop or a banjo.
There’s a weirdly unchallenged idea in the ether that machines learn to make art just like humans do, which supposes that humans never practice making things with their hands — which machines don’t have — but simply look at other work and know how to do it.
This might be my bad habit of picking fights with stuff teens are saying on Reddit. But I think this idea that learning is not somehow embodied is a strange vision of craft, artmaking, and even thinking. To me, the idea that machines make art from a pure logic, removed from the feedback of a body in the world, is worth exploring further.
How do artists learn to make art? The question is annoying from the start: Which artist? What art? Which media? What tradition? Which culture? But this question comes up again and again in Q&A sessions when I talk to people about AI. They tell me that in the past, artists learned to paint from masters, and that this is how humans have always learned. A human apprentice looks to a master painter’s works and duplicates it, over and over again, until they learn the skills they need to make their own work.
So what’s the difference? I want to take that question seriously for a moment by challenging the assumptions. Because when this moves into deeper thinking, it gets extrapolated into a much longer argument:
- “Learning from masters” is what AI does, so limiting a tool’s ability to learn would be akin to forbidding artists from looking freely upon other works.
- Artist apprentices had access to free art through museums, rather than paying copyright holders for the right to make that duplication.
- AI takes the position of this student when it gathers training data, only at a much larger scale. A painter of the 17th century may only practice on a few dozen paintings, while the AI trains on 5 billion images.
- AI is “learning” by looking at these images, through some parallel to human artistics practice.
I’m an artist, not an art historian, so this isn’t my area of expertise. But it made me curious. First, how common was this method of “studying the masters?” From the get-go, this is obviously a European thing, not a universal one. But even in European art pedagogy, is this really how it worked? Was it true that apprentices had free access to the work they “trained” on? What was the human student learning from this relationship, and how does it compare to how AI trains on data?
Take this all with a grain of salt. I was curious, so I went digging. Here’s what I found. The caveats hinted at apply here: the argument assumes a Eurocentric frame of how art was learned, so I’m focusing on a Eurocentric system. The first flaw of the argument is, of course, that “artists” don’t “learn” in a universally standardized way. But since the “AI learns like an apprentice” argument assumes the apprenticeship system of Europe, we’re gonna look at that.
The Masterpieces
Sometime after 1390, Cennino Cennini writes “The Book of the Art.” He’s a painter in Florence, and his book tells artists what to do and how to do it. It’s one of the first books we have that describes the formal instruction of art.
Duplicating the works of masters was part of the practice tied to European guilds and their notions of apprenticeship. It was kind of a trade union — The Guild of St. Luke — designed to keep competition at bay. That’s where we get this word, masterpiece. The master piece was the work an apprentice produced to prove they had learned their trade, whether it was painting or woodworking or some other craft. It’s like a final exam, or a master’s thesis. Once made, the artist could join the guild, and begin working and selling works under their own name.
Under the apprenticeship system, the apprentice paid for access to the folks they studied. Later, after developing enough skill to be helpful to the master crafter, they were paid as assistants. After making a successful masterpiece they could join the guild. Before that, you couldn’t really charge for your work — you could only do work for your “master,” and sign their name if they approved.
Art was synonymous with craft then. Today’s definitions of art are driven by a greater degree of conceptual thinking, rather than pure technical instruction. But it’s important not to reduce this tutelage to simple mechanical reproduction. An apprentice learned how to mix paint, stretch canvas, manipulate specific brushes for desired effects. They learned how to see paintings in the real world. They developed relationships with the master and with patrons. There was physical and emotional labor involved in navigating the world of painting, and a steady stream of feedback from those you were in relationship with.
To be clear, I’m not pining for these days, and neither should you. Apprenticeships gave people mastery of a specific tool with specific applications and style constraints. It limited expression to a traditional framework: imagine learning only one teacher’s methods and being forced to replicate them exclusively for years. It was also, as was most of European culture, restricted to elites.
Some Contrasts
The argument that AI learns just as human artists have learned is an argument that generative AI is learning the craft of image making. That’s what the apprenticeship taught: technical and formal skills through feedback. One was about tools, one was about composition.
Human artists go out into the world and learn from images, including replication of paintings and observed material, and then that work is shown to a community or an instructor, typically in search of feedback. If I am saying feedback a lot here, it’s because that is precisely what is missing from the AI training process. AI creates complex mathematical formulas from the patterns encountered across billions of images and text descriptors. Those formulas recreate the original training data, but then we give it random starting points. As a result, it applies this math incorrectly, resulting in new images.
Missing from that process is feedback. We accept or deny the image, but this does not improve the model. It doesn’t take feedback from us, doesn’t know if we’ve downloaded the image or ignored it. It keeps applying the same math to different images of random noise. Maybe that is close enough to an apprenticeship for you, but there’s one more extremely important different about AI and human artists when it comes to how they learn.
The human already exists when they look at these paintings. The AI doesn’t exist until it looks at those paintings. What exists ahead of the AI looking at these paintings are companies: groups of people looking to turn a profit through the use of GPUs. These people looking to turn a profit know that these GPUs, once trained on enough material, will produce new material. So, in an effort to build a product for profit, they collect as much of that material as they can.
If you assume “AI learns,” you are in a bit of trouble, because our mental model is well off. We’ve assumed that there was some “AI” sitting there, waiting to learn. There wasn’t. There was an infrastructure. But the model is inseparable from the data that trained it. And that data has been gathered by human beings, and then used to build a tool they could sell for profit. The AI — more precisely, the diffusion model — exists because it has been exposed to images. It doesn’t exist until it has been exposed to them. The model is, literally, a model of the mathematical composition of 2.3 billion images.
I’ll continue to use “learns” in scare quotes, but it’s so important to make a distinction here. That “learning” is best described as a complex mathematical algorithm that links words from human captions to the most statistically likely pixel arrangements in human images. It “creates” by applying those algorithms to random noise.
What the model “learns” from training data isn’t about the crafting of images — nothing about the operation of cameras or the stretching of canvas is involved. Generously, we might suggest it “learns” compositions and features of images, that is, the ways pixels are arranged and clustered. That’s what these models produce: stylized imagery which competes with professional photography and painters.
How it does this tends not to matter to people who assert it is “learning as people do,” because they believe any simulation of human activity, if the outputs are indiscernible from human outputs, is no different from the activity it simulates. The problem here is that this level of abstraction is very high. A rock can roll down a hill, and so can a child. To say that there is no distinction between that movement is to ignore the vast, complex systems attached to them. It says context doesn’t matter.
Creative Artists’ Agency
Sure, human apprentices also looked at paintings, analyzed those paintings, and practiced duplicating those paintings in a particular style. They broke paintings down into segments and learned how they worked, inscribing that learning into their own canvases. This is, arguably, correct. But it’s also a very tight boundary by which to define the system of apprenticeship: it ignores the complex systems attached to them.
This is why I am so keen to scratch away at the surface of these arguments. Because the context of a system matters. AI’s myths are founded on abstractions, appeals to a common sense that is not common nor sensical. It relies on simplified models of the world and how it operates, models that discount exceptions and outliers. That is not only lazy, but dangerous.
In the case of this argument about AI art apprenticeship, it focuses entirely on a very narrow aspect of art education, which is the observation of paintings or sculptures. Notably, this approach doesn’t transfer to other expressive domains. Few conceptual artists are trained on reproducing Duchamp’s urinal, but more relevantly, photography education doesn’t rely on capturing the scenes depicted by “masters” exactly as they once appeared.
Art education involved other activities, one of which is the skill of natural observation. That is, the artist would go into the world and draw things — nature, portraits, etc — inscribing their own insights into the process. The AI cannot replicate this, because the AI does not have access to the natural world. This may seem disingenuous — after all, the AI has access to photographs of the world! But those photographs were made by someone else. All of them.
I’m not making the case that AI images can’t be art. My argument is that AI images don’t reflect learning, and are not a universal stand-in for how art is made. My argument is that “AI learns as humans do” lacks context, and should not be an argument used in deciding how to regulate or use AI tools.
These models do not make new observations of the real world to combine with the patterns it has replicated. It can only apply math to randomness — generating hypothetical images that could exist. What it brings of its own is only a matter of scale, matched with the random noise in the starting jpeg: not choices or intent.
“Oh but it can!” I hear you say. “Isn’t that exactly what you’ve just described — isn’t the machine making choices as it projects new patterns into that static?”
No. The inscription of an image into static is very different from an artist creating a personal interpretation of what they have seen. Because the craftsman has made their own observations about the world, they are able to exert agency over the decision of the next stroke. They invent the next stroke, solve for it, choosing a path from infinite possibilities. Is this constrained by previous learning? Yes. But it’s also a unique choice, applied to what comes next. It could be as simple as extending the line. It could be as radical as poking a hole in the canvas, or lighting it on fire.
On the other hand, the machine is, in the words of Jean Luc Godard, “a slave to probability.” It cannot make a decision. It must, by rules imposed into its very structure, exert the most probable choice upon the image of noise it has been assigned. We might imagine being given a paint-by-numbers kit and being told we must draw an image by connecting those dots in the order assigned; but humans have the right to smear the paint with coffee grounds. Machines do not make choices about how to follow the numbers.
The frame of noise at the start of the image generation process is infinitely more dense — being a million pixels or so — but it is not a blank canvas. Every pixel represents a decision, but that decision is dictated not through choice but through statistics. It is only because those statistical probabilities are assigned to new, random structures at the outset that these images appear to show novelty.
It’s an accident. If the system makes an interesting or charged image, it’s a coincidence: more to do with the arbitrary chaos of a random number generator producing a noisy jpeg than with any kind of learning.
This is where the idea of “art” used in AI comparisons is remarkably conservative. It assumes that no artist breaks with tradition on their own terms. But the history of art is the history of the break: the avant-garde becomes the next canon. New modes of expression emerge incrementally but also in large full-scale rejections of what has come before. And certainly, one can make avant-garde art with AI tools: misuse, radical rejections of their assumptions, etc. But that’s through the intervention of the human user — not something the AI has “learned.”
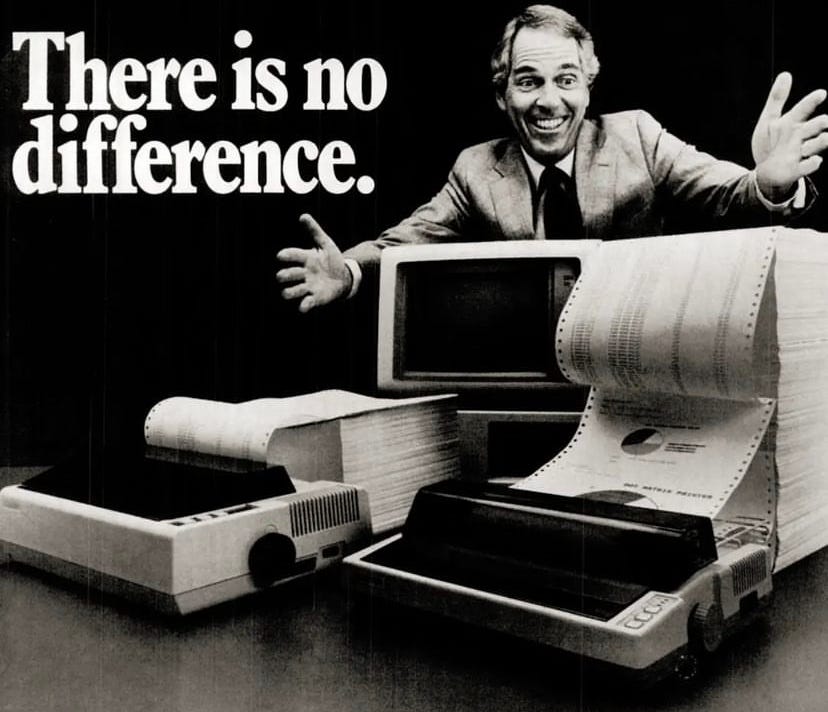
The Copyright and the Monopoly
I’ve heard the argument that artists used to go study images and copy them freely into their notebooks as a way to learn. Those artists didn’t have to pay for access to those paintings, it’s claimed, and so neither should AI companies when they build a dataset for training a generative AI model.
First, let’s assume it’s true that artists learned from free access to images. If so, there’s a conflation here. For one, the artist learned by observation, in order to eventually create an original work of their own that would get them into the guild. If they made direct copies of their master’s work, these pieces could be sold, but they had to be sold under the name of the master. If they went into a museum and picked a random image to practice on, it would be against guild rules to sell that reproduction.
Importantly, again, we don’t pay “the AI” to make these images. We pay tech companies for access to the models they make. The models produce images. So even if we asked companies to pay for their training data, we are not making the AI pay for access to these images when we demand copyright be enforced. We’re asking a tech company to do that.
We also ask curators to pay us if they want to include us in their museum. The tech company uses our data to train a model. The model learns those patterns and once it does, it doesn’t use our training data anymore. Again: the companies use our data, the models use the math derived from our data.
The companies have not learned anything from the training data. It’s very likely nobody at these companies even looks at the data. Based on the revelations about ghost workers being traumatized by images of child abuse in that training data, and the vast amounts of pornographic, racist, and misogynistic content they contain, it would be a legal liability if they did.
This data is collected even before the AI model exists, because the AI cannot exist without it. The AI model is not an agent out in the world. Contrary to popular belief, it is not surfing the internet, “learning” how to paint from what it sees, but is trained on frozen snapshots.
It is the product of a tech company’s decision to scrape our images without paying for them. At no point in the human apprenticeship history has a painter not existed until a master went and gathered 2.3 billion paintings, though maybe I’ll write that short story some day.
The oversaturation of images that we currently experience is a relatively recent blip in human history. As a result, we’re still struggling to define how we steer our legal, economic, and social systems through this noisy landscape of imagery. The debate is not about learning or art-making at all. It’s about how we build machines, or more precisely, how we want to build digital infrastructure.
Rather than aiming to romanticise the days of apprenticeships, I want to point out that this mental model doesn’t apply to the machines. The “master” that it “learns from” isn’t you and I. Instead, the rest of us are turned into apprentices by AI companies: our names removed from our work, to be taken by companies to build data infrastructures without compensation for the labor that went into building their datasets. The AI is not the apprentice in this metaphor. We are, by force. We are told to donate our time and labor in exchange for building corporate profits.
Meta’s head of AI research summed it up:
“Only a small number of book authors make significant money from book sales. This seems to suggest that most books should be freely available for download. The lost revenue for authors would be small, and the benefits to society large by comparison.”
That’s kind of a nice sentiment, if you disregard the reality that devaluing labor is literally the business model of Facebook, Meta and the dataset-driven AI project in general. Cheap data means cheaper models. It’s summed up well in a passage Matteo Pasquinelli flags in his book, The Eye of the Master, in which he cites Andrew Ure’s description of Victorian-era factories as “a vast automaton, composed of various mechanical and intellectual organs, acting in uninterrupted concern for the production of a common object, all of them being subordinated to a self-regulated moving force.”
That’s not an inspiring view of human expression, but I can’t help but be reminded of the way generative AI reduces human expression and communication to a factory assembly line.
The Ruins of Digital-Cultural Infrastructures
We can turn now to a brief history of social infrastructures that responded to this accelerated production of images. In Europe, this jumps us up to the 16th century and to the academic institutionalization of art schools. Art schools were where archives and museums were held, and that’s where lots of aspiring artists could encounter a great variety of styles and compositions. The first European museum didn’t open until 1734, and the Louvre didn’t open until the 1790’s. In the US, copyright law for images was enshrined around this time — inspired by the British system of “monopolies” for trade guilds, which limited who could sell art.
Most art before that was in churches, or in Rome. There’s a niche argument I see online that “artists have always had access to vast troves of visual culture to learn from.” The “always” in question (again, in Europe, which is already a biased understanding of how “all art works”) was chiefly religious art, and for them, it was chiefly Catholic, and among Catholics, it was chiefly in Rome and the Vatican. The notion of having free access to these works of masters is misleading. Artists had to bear the cost of travel. Even then, access was restricted to specific classes and faiths. While private collections held their “proprietary data sets,” all of them paid for the work of the artists contained within them, through commissions.
So, for the most part, artists paid — and were paid — for access to art. They paid artists to teach them how to paint. They paid for art school or apprenticeships. Galleries and museums paid artists to make the work they displayed, which would then serve as “training data” for the artists who visited.
Later, students might look at books in libraries for inspiration, but full color, print resolution is still difficult to produce in ways that serve the purpose of careful attention to detail. Artists hellbent on replication don’t use books or the internet in quite the same way as apprentices, though it can be useful. In any event, a book will contain art that had secured licensing fees, while an aspiring painter could look at these books for free — in a library, which bought the book.
The three-color process saw its first mass popular product in 1893, a copy of a painting of a Gourd by William Kurtz. For the first time in history, everyone could reproduce an image of Kurtz’s gourds! Well, actually, you had to pay for that, too.
So really, the idea that artists had free access to the works of the masters is a pretty recent invention. And unsurprisingly, we might say its most popular advocate was Andy Warhol, who saw “the masters” as ketchup and soup companies. By reproducing the labels of contemporary mass visual culture, Warhol set the art world’s sights on the surfaces of the world around us. After Warhol, artists felt free to grab and steal from supermarkets and magazines alike. These images were then radically (or not so radically) transformed into works of art.
This reflected an explosion of creativity and a complete paradigm shift in views of art that was underfoot across the 1950s and 1960s. Warhol wasn’t responsible, but was emblematic of this shift in what and how we looked at visual culture. In the metaphor of master and apprentice, the master fell away. The world of visual culture far beyond the museums and churches was “democratized” and made available for argument and embrace. When we hear AI artists cast down the “elite” image makers and art institutions, they’re reflecting this rhetoric.
But they’re 60 years late to these radical claims. The visual artist sharing work on Reddit is not an elite cultural force calling for rebuttal. They rely on the Internet — digital infrastructure! — to find audiences and share work. If we were taking art from well-compensated artists with institutional power, and if the images generated by AI didn’t directly reward large tech companies, there might still be a whiff of revolutionary or counter-cultural potential in this gesture. But there isn’t.
The regime of flagrant copyright violation by the Warhols of the world didn’t last long. In 2023, the courts ruled that his work, as unique as it is, is derivative of the sources, requiring his estate to pay photographers whose work his paintings were “trained on.”
We might view the Internet as the modern museum, where a vast many more people are included in the production of an infinitely more dense cascade of digital imagery. We might say, “OK, I can surf the web and see that imagery and learn from it.” All of those things are good and valuable. All of that marks significant process from the time of guilds and “masters” forcing students to replicate images as free labor.
To transfer this concept to artificial intelligence companies, however, is to misapply the metaphor. Sure, an AI also produces images, and some of those images could easily be mistaken for human-made images. As a digital and conceptual artist, I find that very exciting, actually. As a human being, though, I am concerned over the displacement of human artists as a trend tied to the devaluation of labor.
The fair use exception in copyright works reflects an outgrowth of this position that, perhaps, corporatizing all access to all images is a bad idea. Maybe, when researchers are using images to learn something, or artists want to engage critically with an image, they should be able to do so. Each of these comes back once again to the ability of a human mind to make choices about that work, to exert their own expression and curiosity toward it.
AI companies may claim that there is a research exception for building these datasets. I would actually say that’s correct. People should go ahead and use these tools for research. But deliberately training an image generation tool to produce images from that training data is the commercialization of that research.
It’s not about what a generative model makes, but how we make the model. At the moment we have conscripted all cultural production to this end, willingly or unwillingly. If this is to be sustainable, then we need to mobilize a support mechanism for creative expression.
Where are the infrastructures for supporting artists who produce this culture? Are we content with these artists being replaced by artificial intelligence models that cannot reason their way to new ideas, and find inspiration only through constraints on random chance? Are we content to establish a new kind of guild system, where artists are invisibly working to train the models of their corporate masters?
Cultural Infrastructures of AI
Any argument that we should build social and political infrastructures around the idea that machine learning models are “just like humans” troubles me.
I don’t see any value of replacing human metaphors for computational metaphors: no need to call memories “training data,” no need to call humans “agents.” We have a lot of ways of making sense of the world, and a choice about what to prioritize.
It could be as simple as this: machines don’t suffer, don’t experience pain or joy. If you believe that an AI company should be able to make a dataset without paying artists, then you’re also arguing that powerful institutions can do what they want with human labor, as cheaply as they want. That is a consequence of this thinking.
Prioritizing the intelligences that need to eat, in a system where eating requires money, seems like a more ethical framework for structuring our cultures and relationships.
We may not come to common definitions, but I hope we can come to better choices. I would love to see the reliance on copyrights for survival replaced by something that helps independent artists. In the meantime, I make due with the creative commons approach. We need a stronger data rights framework. Perhaps we can build systems that encourage enthusiastic participation in the AI project, rather than relying on subterfuge and legal gray areas. Perhaps we can build a digital-cultural infrastructure that rewards human artists, and allows digital and AI artists alike, to share the benefits of these technologies?
I don’t want to go backwards. I don’t want a return to guilds, or arguments that depend on the guild system to support the rights of Microsoft. We should protect artists of all kinds — whether you’re using a pen, paint brush, or keyboard — and support curiosity and engagement with new ideas. I don’t believe that’s going to achieved by letting these companies build systems that rely on data people don’t want them to use.
So maybe we can imagine something different. After all, we’re humans. We aren’t confined to the decisions of the past.
Things I am Doing This Week
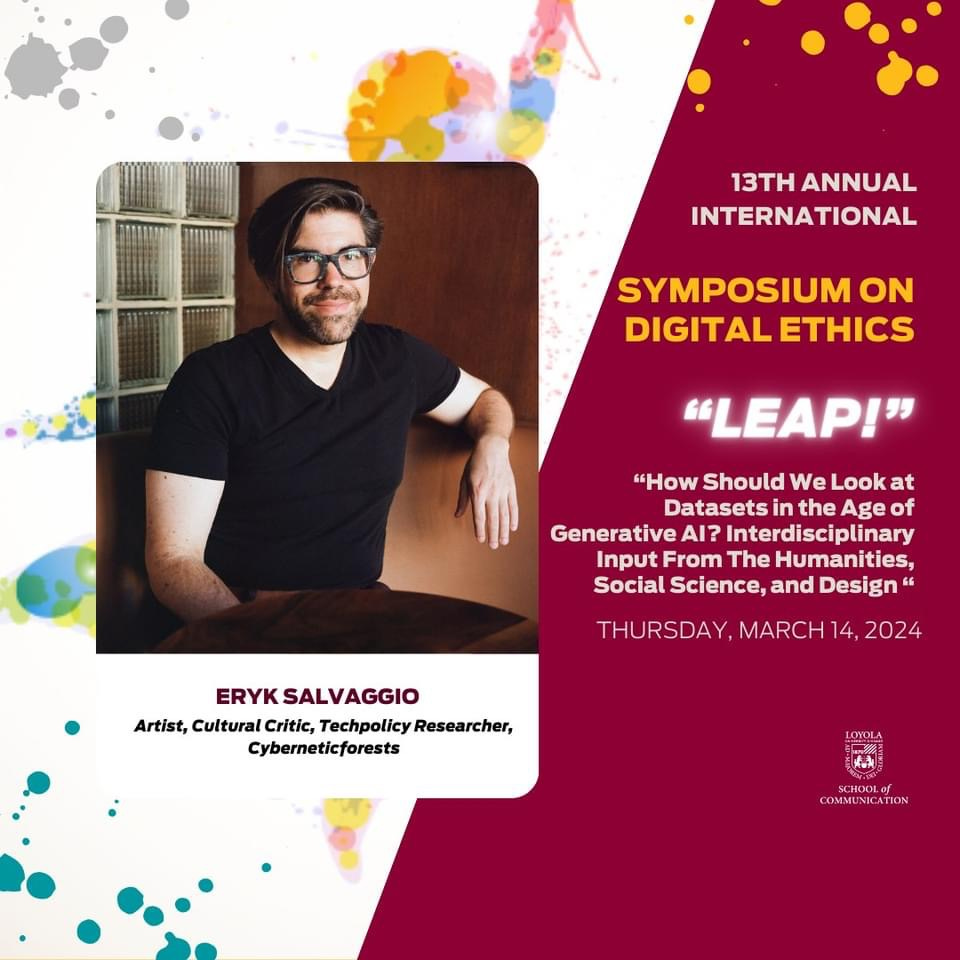
March 14: Leap!
Loyola University Chicago & Zoom
I’ll be speaking in-person at the 13th annual International Symposium on Digital Ethics at Loyola University Chicago’s Center for Digital Ethics and Policy this week. The sessions will be streamed on Zoom and focus on media, education, and tech, with a lot of AI on the table. The event will be accessible in-person or via Zoom, if you can’t make it to Chicago. Info after the button.
March 14: AI or Not AI?
UC Fresno (Zoom only)
That same day I will be the final speaker of a months-long UC Fresno series on AI, art and culture organized by Dr. Ah Ran Koo, which has included artists I knew (Anna Ridler, Lev Manovich) and many cool artists I hadn’t discovered yet! Registration is free. Register with the button below by the 13th to ensure you get the Zoom link to my talk.