What I Learned About AI in 2023
A Very Niche Foresight Report
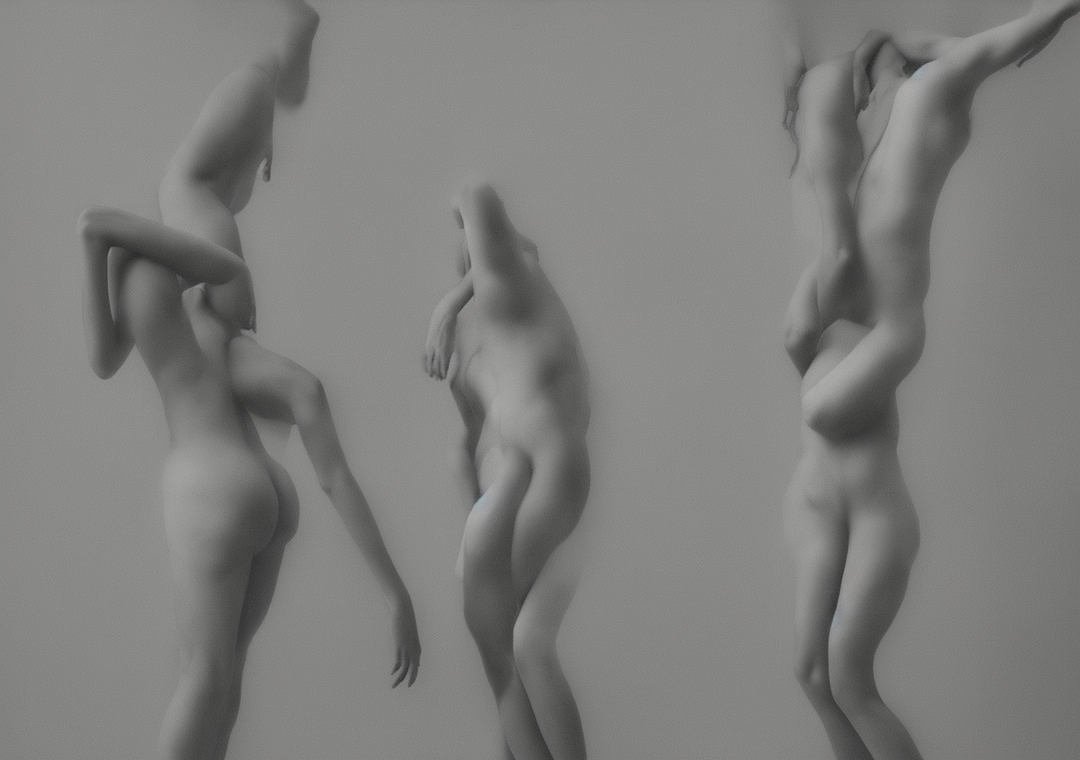
For some reason, I’m haunted by fear that I am a pessimist. In my daily life, I try to remember that cynicism isn’t realism, and that optimism doesn’t necessarily make us into suckers. Despite this, when it comes to AI, I can’t shake the feeling that every advancement is part of a long pattern of repetition, where each new iteration erases any learning from previous failures. In technology, failure doesn’t exist: it just gets replaced by whatever will fail next.
My optimism is what draws me into the practice of writing about AI the way I do. In many ways, I would like to see AI improve. But improvement, to me, means more just, more ecological: more attuned to solving meaningful problems, rather than creating new ones. The mad burst of activity we’ve seen in 2023 around AI has steered us in that direction. The technologies that came to define AI this year seem, in fact, hopelessly disconnected from problem solving, in spite of the siren song of “potential” that emerges from the companies embracing this new use of our data.
There is some evidence that AI is making things worse, even the stuff it is meant to be doing well. In the wake of 240,000 tech industry layoffs, many focused on ethics and safety teams, there’s been a loss of expertise behind the services we’ve come to rely on. At the same time, there has been a mad rush to incorporate wonky, misunderstood generative AI algorithms into backends. All of this is tied to what Cory Doctorow described as “enshittification” in an essay appropriately published in January, and may as well be word of the year in 2023. (Oddly enough, the widely suspected claim that AI itself is getting worse may be an illusion rising from familiarity).
It’s also possible that users have become more savvy, as Sarah Watson notes in an article about the gradual decay of apps. There’s also the marketing pressure to push more experimental features to market sooner, and, as Leif Weatherby wrote this summer, the conflation of owners and managers is increasingly turning tech into personal projects that the rest of us are supposed to enjoy.
AI would seem to fit into this category, as we saw from the reaction to Sam Altman’s much-ballyhooed weekend of unemployment. The cult of the AI leader is very real, and it seems to surround Altman and Musk most strongly. Yet, Altman and Musk aren’t researchers, not “computer guys,” they’re money guys. That’s because you need money to run these models — positioned as the future of our entire economy even as they lose about $700,000 per day.
This lens has created a lot of distortions around how AI works and this concept of “potential,” especially as 2023 was the year where Altman and Musk were the go-to resources for framing AI risk. It’s no surprise that significant verbiage from newspapers and policymakers focused on “existential risks” and “alignment” concerns. It seemed like every news report had to have at least an off-the-cuff remark about how “some even fear the technology could end life as we know it.”
Compare this to coverage of climate-destructive, but “everyday” technology, and you start to see some weird biases: how many newspaper articles about the automotive or aerospace industry reference the damage to “life as we know it” that rises from fossil fuels?
This year we’re finally seeing some of the harms and threats that AI may actually produce, as opposed to the speculative, world-ending scenarios that lead some AI zealots to advocate for bombing AI training centers. On the bright side - and only briefly noted in this post, because I’ve written about it before - we’ve seen a meaningful exercise of worker power over emerging tech in the form of the WGA strike. So here’s a bit of a breakdown of the year 2023 in AI, what we’ve learned so far about real and imagined threats.
Thirsty iPhone Chargers
If you were interested in AI and the environment from, say, 2019 to 2023, you had one reference that you could go to: that training a single AI model emitted about as much carbon as the entire lifecycle of five cars. If you wanted to talk about AI and the environment, that seemed to be the only useful frame of measurement available to you.
Luckily 2023 has given us more transparency. If you’ve ever run Stable Diffusion on your desktop, you know that the speed with which you can generate images using cloud infrastructure like Google Colab is significantly faster than your measly little 2018 Best Buy purchase. That speed can undermine just how much compute goes into a single image, because it seems “fast” and “lightweight.” But the opposite is the case.
From Alexandra Sasha Luccioni at Hugging Face, we’ve learned that a single AI generated image uses as much electricity as charging a cell phone, which is going to join the five-cars fact on slide decks everywhere. (Read a pop press article on the findings here). Most of these tools generate four at a time, so it’s about four cell phones for every spin of the Midjourney roulette wheel. This can vary, based on the location of the cloud server running the operation: companies could build solar-powered cloud networks and drastically shift that performance (or put data centers inside wind turbines) but that’s still energy that could go somewhere else.
Shaolei Ren and others at UC Riverside and UT Arlington have shown just how thirsty these systems can be. Because they need water to cool data centers and to power them, GPT3 used about 700,000 liters (185,000 gallons) of water. The much-expanded GPT4 likely used more. Ren’s paper predicts that by 2027 AI could evaporate as much water as about half of the UK uses every year. It’s about a bottle of water for every 10-50 responses for a GPT3 response, and again, GPT4 has only become more energy exhaustive.
Part of that transparency has shown us how important the money is, but also how important access to natural resources is: for huge companies, this vast energy expenditure is affordable because it can sustain the money burn. Smaller startups, especially those looking at green algorithms, may find it more challenging to compete. Green algorithms would use less computational power — which uses massive amounts of energy and water. But greener AI also makes it easier to compete with the behemoths of cloud power. In other words, more efficient algorithms are better for the environment, but reduce the barriers to competition for the current leaders, OpenAI/Microsoft, Google, and Meta.
Hopefully we’ll see a policy investment in computational infrastructure, one that makes this infrastructure more sustainable. That means funding significantly more research into environmental effects of AI. On that note, I’m following Mel Hogan & the Data Fix podcast, and found a lot to think about in Tamara Kneese’s AI Now report on Climate, Labor & AI.
AI in Education
I was among the instructors bracing for the AI apocalypse in my classroom. Turns out, claims of cheating using AI have been largely overblown. A slew of startups that aimed to capitalize on that fear proved to do more harm than good, often times singling out non-native English speakers. Reports of professors trusting AI detection tools at the expense of punishing entire classrooms started to circulate.
Anecdotally, I did not see a rise in cheating in my classrooms. Rather, most students found Generative AI laughably unreliable and frustrating to use, even when I did my best to position these tools in a realistic, but neutral way. Those who did use it used it — with my permission — to improve their writing, not replace it. That’s in line with findings that AI can improve the bottom-performers at a specific work task, with little improvement to medium and high performers. In a way, this is kind of a blessing: many students may have good ideas, but struggle to write. If they can use these tools to complement the ideas they want to express, all the better. It’s when they replace the writing-as-thinking, by simply asking for an essay and handing it in, that they become a source of frustration as an instructor. As far as I can tell, nobody has done that, and I think I would be able to tell.
That replacement of the work of making is at the heart of generative AI in art, as well: the threat that the labor of creativity, the quest to express ourselves by transcending our own limits and finding our own forms of expression, becomes lost when we accept what the machine gives us — especially if we define all expression in a limited visual vocabulary of “beautiful images.” But like writing, I think this is a simplistic view of the relationship with generative outcomes. In the AIxD Story & Code residency and report this year, I’ve seen lots of folks labor over how to build ethical workflows and preserve their vision, despite AI, rather than seek to replace their ideas with the models. There are still ethical issues with Gen AI, obviously. But the “nobody can ever be creative” trope about AI speaks more to the limits of imagination on the way we use and abuse technology to our own ends.
In high schools, the idea that AI would lead to increased cheating may turn out to be false, but curiously under-examined is the claim that about 70% of US high school kids cheat anyway, with or without AI. Working with AI with a group of 40 first-year students, I came to laugh at headlines and blue-check X users who confidently declared that my students would “already know ChatGPT.” Most had heard of it, few had used it, and even fewer had used AI image generation tools. These are students interested in computers and design, by the way! An audience you’d think would be ripe for that kind of thing.
I taught a class on image generation with AI this year, which is fully available online. Even there, many students approached the tools with steep skepticism and left with a heavy reluctance to engage, given the ethical concerns around the technology. By contrast, I have to say, in chats with European educators — especially in design schools — I’m told that more European students seem engaged and wanting to grapple with these systems. (My modules for Elisava’s Responsible AI masters program are beside the point, since that would be obvious). This is a very small data pool, but it’s interesting nonetheless. Part of me wonders if I’m the pattern: if I am teaching the tools too critically.
In design education I think we need to advocate for these tools as “problematic but worth understanding,” especially if we want to somehow build better systems. Much of that may be on the horizon, and I keep seeing models announcing themselves as ethically trained. There’s demand for strong model ethics, and I can’t imagine that goes unfilled for very much longer.
Either way, I was excited to be part of a great collection of resources and lesson plans for folks interested in teaching AI, which is the Harvard MetaLAB’s AI Pedagogy Project, which blends critical and applied approaches and links to a great variety of useful resources. Perhaps I will find some inspiration for the “preparing students to use them” side of things rather than the “warn them about the risks” side. But I do believe both have merit, as does sitting with the ambiguities and tensions of emerging technology as a way of finding one’s own relationship with them. Perhaps America is too polarized on tech to expect that from our teenagers, but then again, I don’t blame them.
Also: I would love a job teaching responsible AI in an art or design school, if anyone wants to point me to some leads. I say this a lot and I worry people think I am kidding. I am not kidding. Hire me.
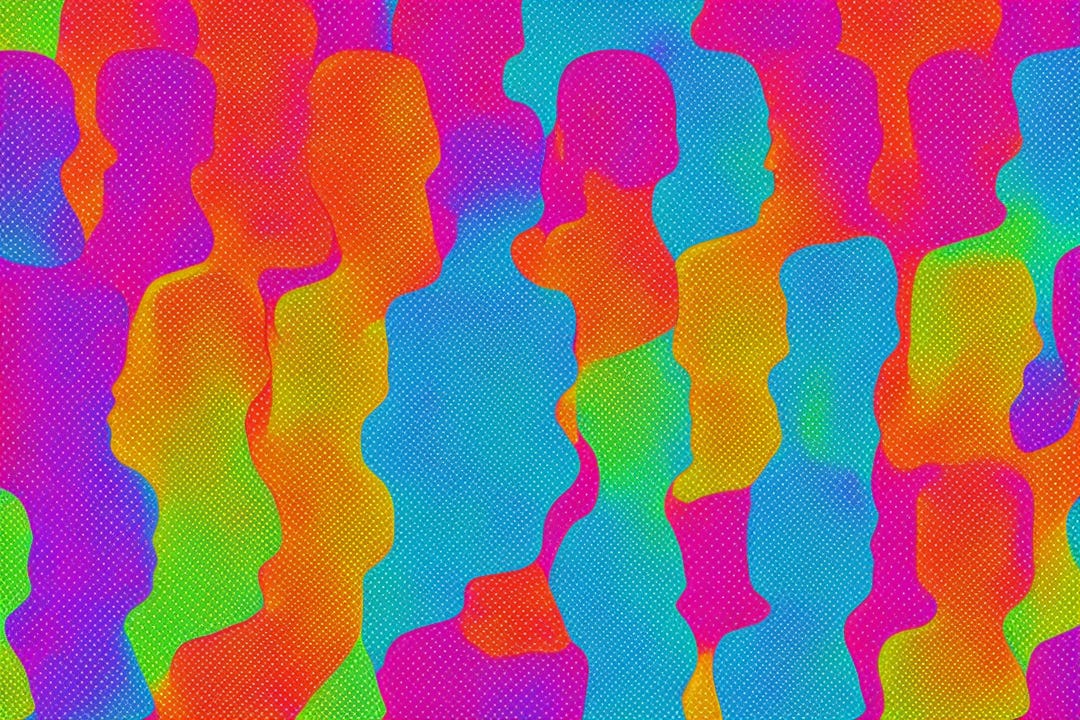
The End of SuperAligned SuperIntelligences(TM)?
Meanwhile, there’s a huge investment into top schools to model a particular view of AI: that of “existential risk.” Stanford is getting a lot of money to study “alignment” of AI against such threats. All we have to do is create a system where all humans agree on a particular set of values, and then instill those universal values into the technologies we build by translating them all into if/then statements. That should be easy: after all, look at how good humankind has been at collective decision-making, finding common ground, and making sure everyone can participate in democratic systems. Pair that with the clear-cut ethics of our everyday decision making, and this is cake!
Extending that sarcasm, much of this effort has been bankrolled by ethical experts like Sam Bankman-Fried, who we know in 2023 has stolen billions of dollars from customers of his crypto exchange. Other luminaries in the space include the “philosopher” Nick Bostrom, who gave a full sorry-not-sorry apology in January after writing “Blacks are more stupid than whites” and asserting it was “logically correct” before topping it off with an “ironic” use of the N-word. In his apology, he extended the damage by claiming that the issue of genetic inferiority between races was unresolved. Oh, and Elon Musk. Musk loves this stuff.
But alignment of a non-existent technology against a theoretical risk of extinction is all a bit of bunk. Model alignment is ideal for specific purposes, but impossible to generalize. Yet, Silicon Valley folks have spent significant mental power trying to sort out alignment problems for tools that don’t exist, and are yet envisioned to be able to do a whole range of human tasks. The problem is that alignment is in the eye of the beholder, and some of those beholders are assholes. If we can align an AI to behave according to a universally applicable standard, then bad actors can align an AI to behave against it. And “bad actors” do not have to be terrorists or crime rings: they can be deluded, highly privileged, wealthy, elitist people with power to custom build tools and deploy them to the rest of us.
OpenAI’s current solution is to build a small series of AI systems that will modify the behavior of larger AI systems: “super alignment.” It’s also not focused on existing systems, but on theoretical systems: “Artificial General Intelligence,” (AGI) that is, a machine that can do any number of “general” tasks.
AGI Alignment is predicated on solving problems in a way that is isolated from designing any specific technology. How you regulate or constrain behaviors of a technology when you don’t know how that technology operates requires a bit of psychic foresight. In other words, it’s science fiction at worst, and at best, nonsense relying on myths of objective data.
If 2023 started to really scratch the weirdness of TESCREAL ideologies, and market pressure might start sidelining it. If there is any bright side to Sam Altman’s weekend off before re-taking control of OpenAI, it’s that it supersedes the work of Ilya Sutskever, who was working on an AI to superalign superintelligent AIs and yes, that’s really the language they were using.
Evidence to the contrary, though? OpenAI recently announced $10 million in grants for “Super Alignment” projects. In May of this year, they launched a “Democratic Inputs Into AI” fund that generated no meaningful conversation, though funded projects had to publicly report on outcomes by October 20, 2023. It’s worth noting that “Super Alignment” — using AI to mediate AI — was literally worth 10x more to OpenAI than the Democratic Inputs fund — giving humans tools to decide how to mediate AI.
This kind of bunk was at a complete remove from the automated social and environmental harms that his technology actually poses. Altman, by comparison, is a salesman with traditional California Ideology vibes. Neither seemed particularly interested in building systems that solve problems. But Altman is a much more comprehensible form of opposition. Because you can’t argue with machines that might never exist.
Generative Racism
I was a little aggravated to see Rest of World, a publication I’ve admired from day one, run an experiment I’d shared in my class (and had presented as early as 2019) without giving me so much of a head-nod. But it’s OK, because I am using their results as supporting evidence that generative images are made entirely out of “means,” that is, they’re infographics for the underlying dataset. Rest of World pushed my examples to the extreme, generating 3,000 images to show the common stereotypes that emerge from Midjourney. Sombreros for “Mexicans,” beards and turbans on old men for “Indians,” etc. (Their work, and the evolution of my own, owes an even greater debt to Abeba Birhane’s 2022 paper).
I don’t think we saw much that was new on the AI equality front in 2023, and that’s disappointing. Tech companies keep churning out the same sludge even though it’s been predictable for years. In 2022, Meta put out a racist chatbot. In 2023, it went ahead and put out a racist image model. Every time it’s the same line: “We’ll continue to improve these features as they evolve and more people share their feedback.”
I’ll expect to see this phrase again and again as a boom of new models floods the market. All deployed without learning lessons of any previous models, all deployed with the idea that they will let users quality test these things until they’re fixed: much like the video game industry, with its constant flood of launch-day bugs.
What’s so often overlooked by these conversations, and ought to be common sense by now, is that diffusion models built on scraped internet data are inherently stereotyping, not only because the Web is built on an enormous library of racist text and images. But because even without the blatant racism of memes and 4Chan, subtle linguistic tendencies define and shape the categories that images are reproduced from. Without system interventions at the outset, or even the most concentrated effort at compiling a diverse and racial-justice-oriented dataset, these models will always produce racist and stereotyping results. That is what they do. Unless we move away from Diffusion based systems, that isn’t going to change.
As of this writing I am still waiting for full details of the EU AI Act. But the White House Executive Order on AI at least included some of this language in its fourth guiding principle:
“Artificial Intelligence systems deployed irresponsibly have reproduced and intensified existing inequities, caused new types of harmful discrimination, and exacerbated online and physical harms.”
In 2024, we’ll see if they do anything about it.
Red Teams
“Red Teaming” is a term used in information security circles to describe the practice of playing out attacks against your own tools in order to identify weaknesses and fix them. For example, a bank might hire a “red team” to attempt to hack its online chatbots or password security. The red team plays as if it is adversarial, pushing the limits of the system and tasked with identifying new ways to break or hack them. Once these techniques are identified, they are quickly patched up, with the aim of finding weaknesses before unfriendly actors exploit them.
I’ve always been interested in artists as “red teams,” and this year I was happy to see that work recognized at events like DEFCON 31, where we were able to talk about how artists respond to socially irresponsible, glitchy AI tools. The paradox of red teaming is tied to exactly what I just described above: it gives companies a way to deploy and rely on users to do their quality assurance work. Digital artists, glitch artists and others have always been looking at these exploits and using them to make art. Socially conscious artists are exploiting these weaknesses to make socially conscious art, in essence red teaming both for the company that makes the tools — but also revealing other underlying forms of harm.
Artists don’t work exclusively in information security realm. They work in the realm of the social, and the vulnerabilities artists can identify transcend the technical systems and look at techno-social systems, and the vulnerabilities that emerge in that contact point.
Red Teaming has gathered a lot of attention, including the involvement of the White House: it is a bit astounding to say that I worked at an event that the White House was actively involved in, primarily because nobody involved said a word to us the entire time we were there.
I can quote myself:
Security and social accountability aren’t contradictory per se, but there’s a marked difference: security experts see LLMs as targets of intended harm, while many of us see LLMs as perpetrators of harm. Red Teaming, in which a player takes on a playfully adversarial role against a system, operates within the confines of a tacit agreement that the player will improve that model.
There’s a kind of complicity in that, but I think artists can see themselves engaged in Red Teaming under a wholly unique framework: one that exposes “vulnerabilities” not just in the software we test and break, but vulnerabilities that touch the communities that we care about. Complicity is certainly a concern, but it’s also a choice. I’ve been intrigued by the concept of parasitical resistance, which is the delicate work of maintaining positions alongside the institutions (or bodies) you wish to change without being overcome by them. (see: Anna Watkins Fisher).
I hope “artists as red teams” can challenge what red teaming is and does, how it works and who it serves, and I hope it doesn’t change the distance that artists have in that relationship, which is a source of power. Red Teaming was discussed a lot in 2023 — not much with artists, of course — but even had its own section in the White House Executive Order. I hope the conversation of artists in this space continues to gain traction in 2024.
Some Expectations
Surprises do happen, thank God. I wrote my last “predictions for next year” article in December of 2019, and I missed that whole Covid thing, so I don’t pretend to do it anymore. But I have been paying attention and right now, this is where I sort of expect things to go. But who knows?
To that end, we got an Executive Order on AI, which may manifest into something more interesting in 2024. But the Executive Order, and the (previously mentioned) WGA victory over AI rules, are both promising signs of policy and the public shaping tech. Less promising is the issue of copyright protection for artists who share their work online, but I find it impossible to imagine that we will not see more artist-friendly models and datasets moving forward.
I’m mostly convinced that the TESCREAL / AGI Alignment stuff is going to get swept aside, because the investment and spending, and the expectations for ROI, are so high that these companies will have no choice but to accelerate into products at the expense of abstract hand wringing about science fiction scenarios. That’s not a good thing, per se, but given that “longtermist” fears have never given much thought to automated capitalism, it never offered much of use, either.
Predictive text is going to have to find a business case, or invent one. The hype for these products is setting it up for disaster, because generative AI simply can’t do many of the things people predict it will. Generative text is supposed to transform search engines (it’s made them dirtier and worse) and transform education (sure, but perhaps not the ways we want it to). It can’t write screenplays (technically, and now, under union contracts). Even the video game industry, speaking to the investment realists at Bain Capital, suggested that generative AI would not be used to write storylines or dialogue anytime soon; nor did most game studios expect to see any reduction in costs as a result of generative AI.
Could we see a wild expansion of generative AI pushed into products by the end of 2024? Will there be an OpenAI branded Furby? Video games that use generative AI (most likely in very simple, pictionary-type ways?) I’m eager to see what weird new kitsch comes from AI in 2024. But most pressingly, I worry that AI’s “productivity” drive will bring a desperate correction to AI’s ongoing, unsustainable financial nosedive. I assume AI will become more expensive to individual users, reflecting real-world costs. I assume AI will become more concentrated into the hands of the wealthy as a result of that cost increase, and I expect that we’ll see an acceleration of unsavory investments, such as a16z’s investment into a non-consensual pornographic project which paid users to create deepfake images based on uploaded images, and offered models that exploited children.
The more this stuff gets embedded into dating apps, refrigerators, car stereos, stuffed animals and pet collars, the more the energy goes up. There is very little chance that it will do anything better than we already have now, but I expect that quite a few people will try. Along the way, quite a few high-level productivity tools are bound to cost some company a slew of talented workers after they’re mistaken for expendable: I’ll guess it will be a media company, but maybe it will be a financial app (think Albert, or Mint).
Since I refuse to ask GPT how to end this article, I’m going to leave it there. It may be unsatisfying, but that’s what we get when we try to predict an unpredictable world! It’s anybody’s guess.
Thank You!
I may get another newsletter out before the new year, but I appreciate your patience with my break as I was traveling to Prague to deliver a workshop to the Uroboros Festival! I wanted to add a quick note of thanks to all of you who read, share and support this newsletter. I am hoping to escalate some of the stuff I’m doing here in 2024 and I hope you all dig what I have planned.
Fun Fact: In 2023, Cybernetic Forests tripled its readership. I’m stunned, and deeply grateful! Sincerely, because so much of that growth and readership has been a direct result of you all sharing it. I don’t advertise and Twitter censors substack links, so I’m building this audience through word of mouth alone. So, thank you!
New Releases on Latent Space Records!
If you’re looking for some AI-derived dissonant drone this holiday, I’ve got you covered.
Latent Space, the label I started to document experimental uses of AI sound tools, has two new releases available for streaming & free/pay-what-you-want downloads: Andrea Bolzoni’s ThreeNNbRe-act and Party Music’s Commodity. Bolzoni’s work is a collection of experimental processes between MAX, a Korg, and Neural Nets. Party Music trained SampleRNN on fragments of ‘60s Sun Ra recordings (Cosmic Tones for Mental Therapy + The Magic City) mixed in with early Sonic Youth (Confusion is Sex/Kill Yr Idols) to generate new textures that were then stitched back together. Highly experimental and exploratory, for fans of the noisier, dronier side of AI sound synthesis!