What was the GAN?
RunwayML is shuttering its original AI art toolset. Get your history while you can.
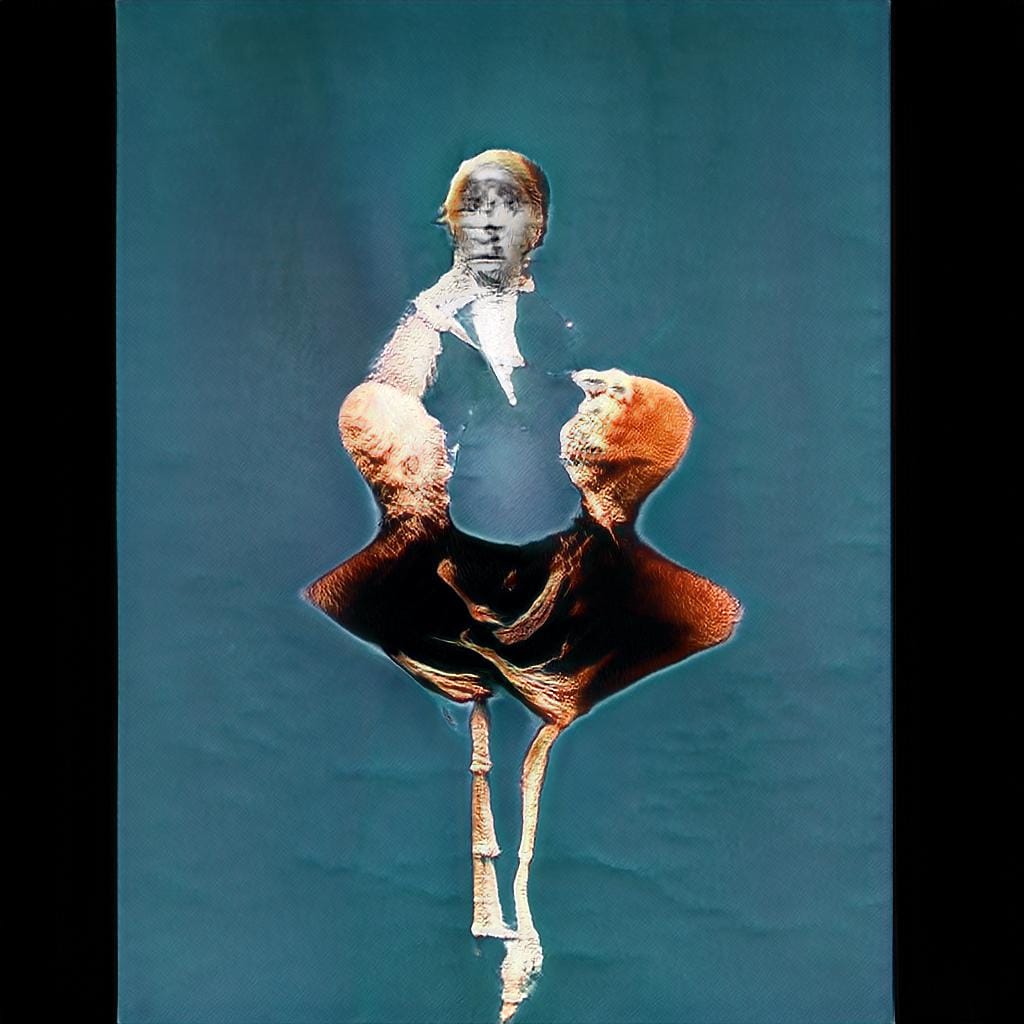
Generative Adversarial Networks were my introduction to AI art. For a while, they were state of the art — capable of generating photorealistic images based on patterns found in large datasets of images. Today, RunwayML, a web-based app and AI playground for creatives, announced that it would be killing off its machine learning tools, GANs included, in favor of diffusion based images and video.
This is a shame, because GANs offered a unique level of control to artists — and room for craft — that Diffusion-based systems do not. While the difference can seem quite technical, I want to talk a bit about the GAN era and what it meant for artists.
First off, there’s the practice of making work with GANs. Unlike the AI art of today, which uses billions of data points paired with text and captions, GANs were trained on a folder of images. That folder’s images had to be prepared by the human artist for the GAN to be able to make any sense of it.
I don’t want to glorify that aspect of GANs or have it lost to nostalgia, and many of these massive commercial datasets were also used for surveillance systems. In commercial applications, this meant often times exploitative uses of ghost workers paid a few pennies per image for cropping images and evaluating them for consistency.
Artists, however, would do this themselves, and in a way it created a relationship and transparency into the systems. Artists such as Anna Ridler have described taking thousands of images of tulips, checking in on the distribution of photos in the dataset, thinking about what images she’d need to collect next in order to build the set in a more complete way.
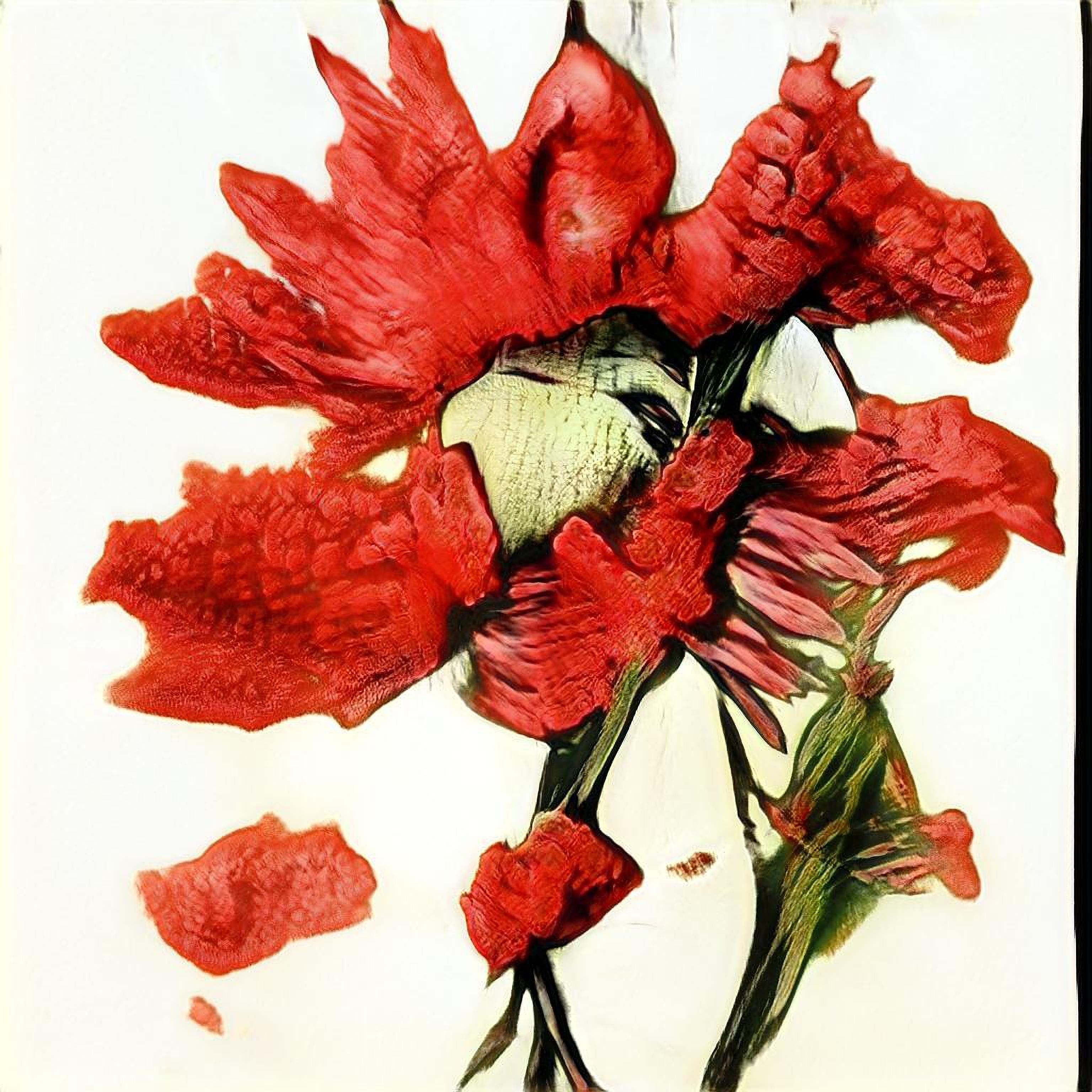
Once these images were collected into an archive, the photos were used to train a model. While Diffusion systems today train on billions of images, assigning them categories based on surrounding text, GANs could only produce extensions of what was in that training data. Give it a thousand tulips, and you’d get a thousand more tulips, but they’d be new tulips. Tulips that replicated patterns learned by the model.
Even on a technical level, the generative adversarial network was a useful metaphor for friction between reality and falsehood. GANs had three steps: first, the training data would be studied for patterns. Then, a generator would make an image based on pixel arrangements discovered to be common across that training data. The generator and the training set would send a sample image to a third processor, the discriminator.
The discriminator was tasked with comparing the two images and deciding which one was closest to the training data. Imagine you’re a contestant on a game show, analyzing which image is a forgery. If you guess right, you explain why and then send it back to the forgers to make a better forgery. That’s what GANs would do. The generator would learn what not to do, and what to do, to get a better score. Eventually, the generator is able to consistently “pass” the test of the discriminator, at which point it can produce images that look — at least to a simple machine — like something in the original dataset.
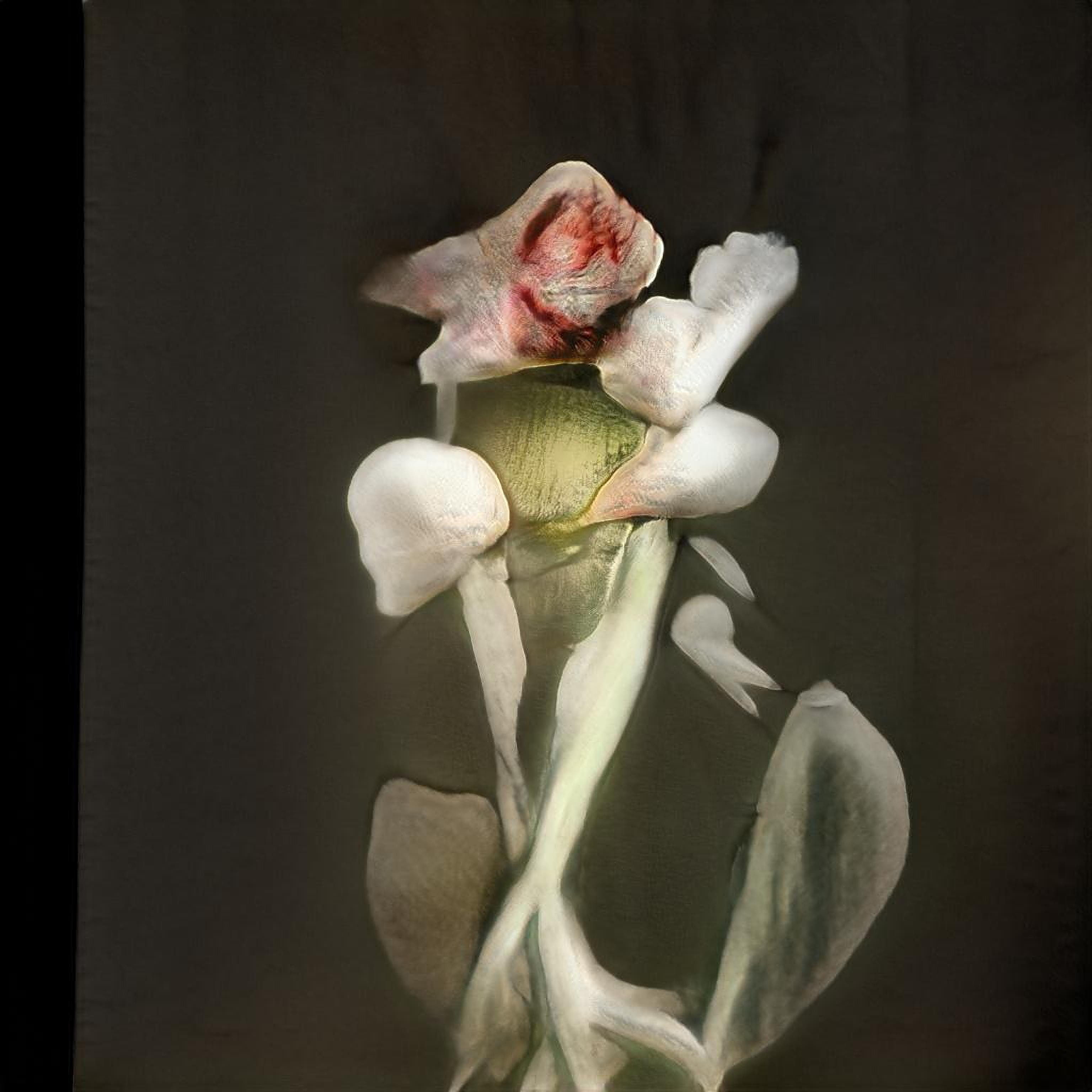
By building our own datasets, we also pitted our own works as photographers — our own ways of seeing — against the machine, in a literal, technical process. We could shape the outputs of these systems with our cameras, in the studio, or in a field taking pictures of leaves or on a beach surrounded by barnacles and driftwood. We had to think like the GAN, as if it was a real collaborator. We had to learn the way it saw, and this was an incredibly useful skill for those of us who engaged with it. It changed the way we saw ourselves in relationship to algorithms, on a scale that went well beyond images themselves. The name itself — generative, adversarial — was a useful description of these contradictions.
Because artists compiled their own datasets, GANs have been useful tools for thinking about the processes of AI systems, from the data assembly line to the output. There was less direct control, but more complete control in the places that mattered most. Today, with Diffusion models, most of their users do not have control over the datasets they use (they can, which is different from do).
This is a shame, because it was this thinking about the dataset that was most illuminating to me as an artist: what would I photograph, how would I photograph it, what kind of things did I need to see in these images? I would download public domain images for textures and shapes and figures using the same logic. I knew what was in my archive and that it was ethically collected.
I wrote several pieces about this — Seeing Like a Dataset was published by Interactions of the ACM this year, and Infinite Barnacle: Notes on AI Snapshots will be published in Leonardo in 2024. So I won’t dwell too much here.
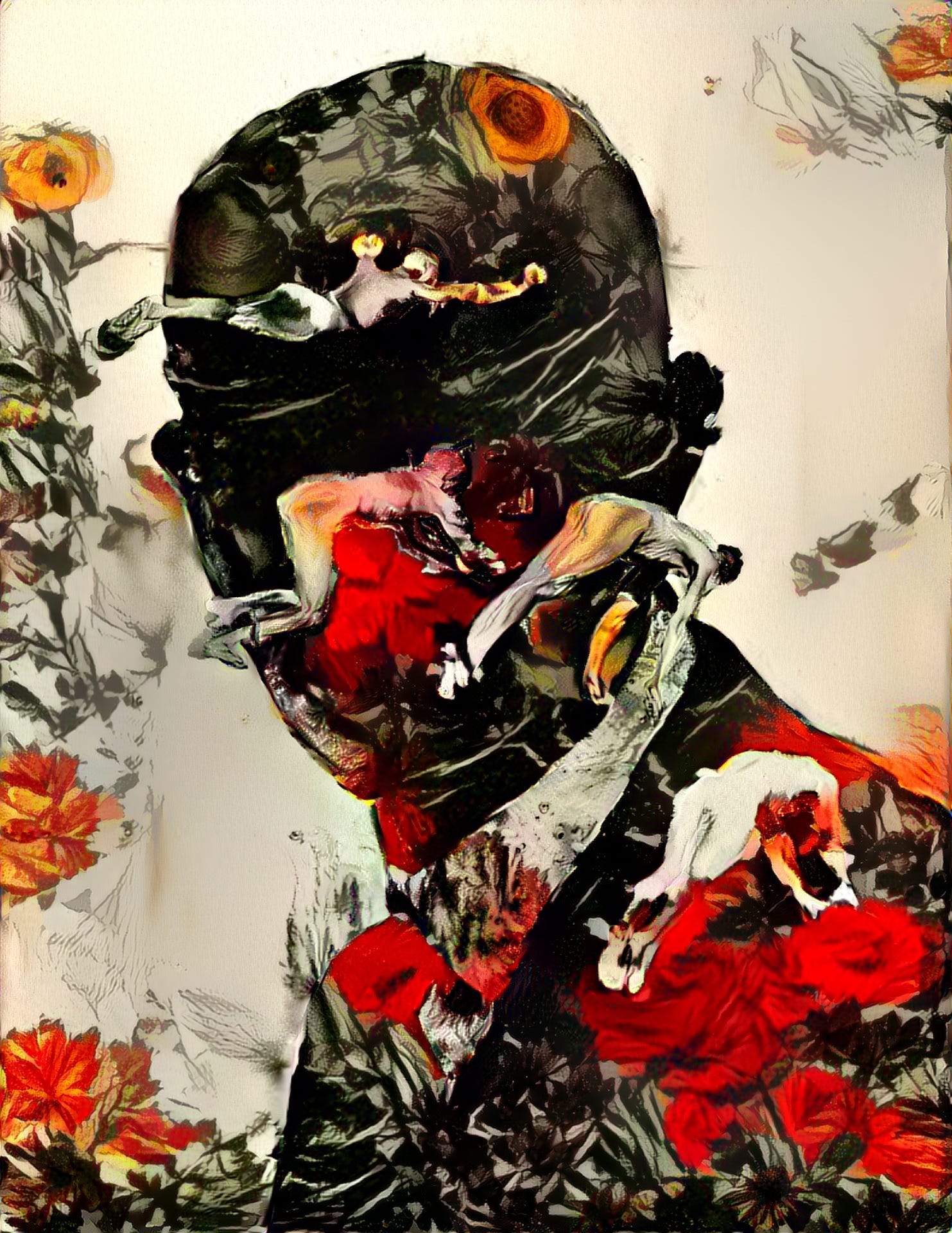
The work I did with GANs was always an experiment, and none of them have ever been shown or collected outside of those articles. There wasn’t much of an interest in this kind of thing when I was doing it. A few models I trained and hoped to tweak I never got to, either, and now never will.
I taught GANs in my AI images class, and I’ll lament their demise as a pedagogical tool. It was so helpful to illustrate the data pipeline and all the ways we could intervene.
Of course, GANs will still exist, and there are thousands of models and notebooks out there to run them, but the loss of Runway — which has buried its ML Labs for years already — means less students working in this way.
It also means the end of many models that were trained on Runway. Artists who made work on Runway during its early years will have to download their models and run them elsewhere, which is a bit like a book publisher telling folks they can still buy any book they want, so long as they bind those books themselves. A lot will be lost in this transition. I just found out that Runway was closing the labs down a week before the official date, and already I can’t find it to run my models one last time.
Aside from Anna Ridler, many GAN works came from artists with links to industry. Refik Anadol’s pieces at the MOMA are GAN based. Mario Klingemann, Gene Kogan, and others relied on GANs both to generate and define AI images through their own interventions and systems built on top of them. The Lava Lamp criticism is chiefly a GAN critique: it was prone to smeary, velevety textures and blobs, and a tendency toward red. That was just an artifact of what they did.
Runway was the space where folks like me — dweeby policy people — could make our own experiments. For archivists of digital art, this is the loss of an already overlooked period of AI art history. Not quite a disaster, as the results of these models are out there and the tech still exists. But so much of the early history of generative AI art is going to get locked in some digital closet on November 30, 2023. GANs will live, but GAN art is, I think, officially history.
So, here’s some work I realize I never shared with anyone from those early experiments. On to the next.
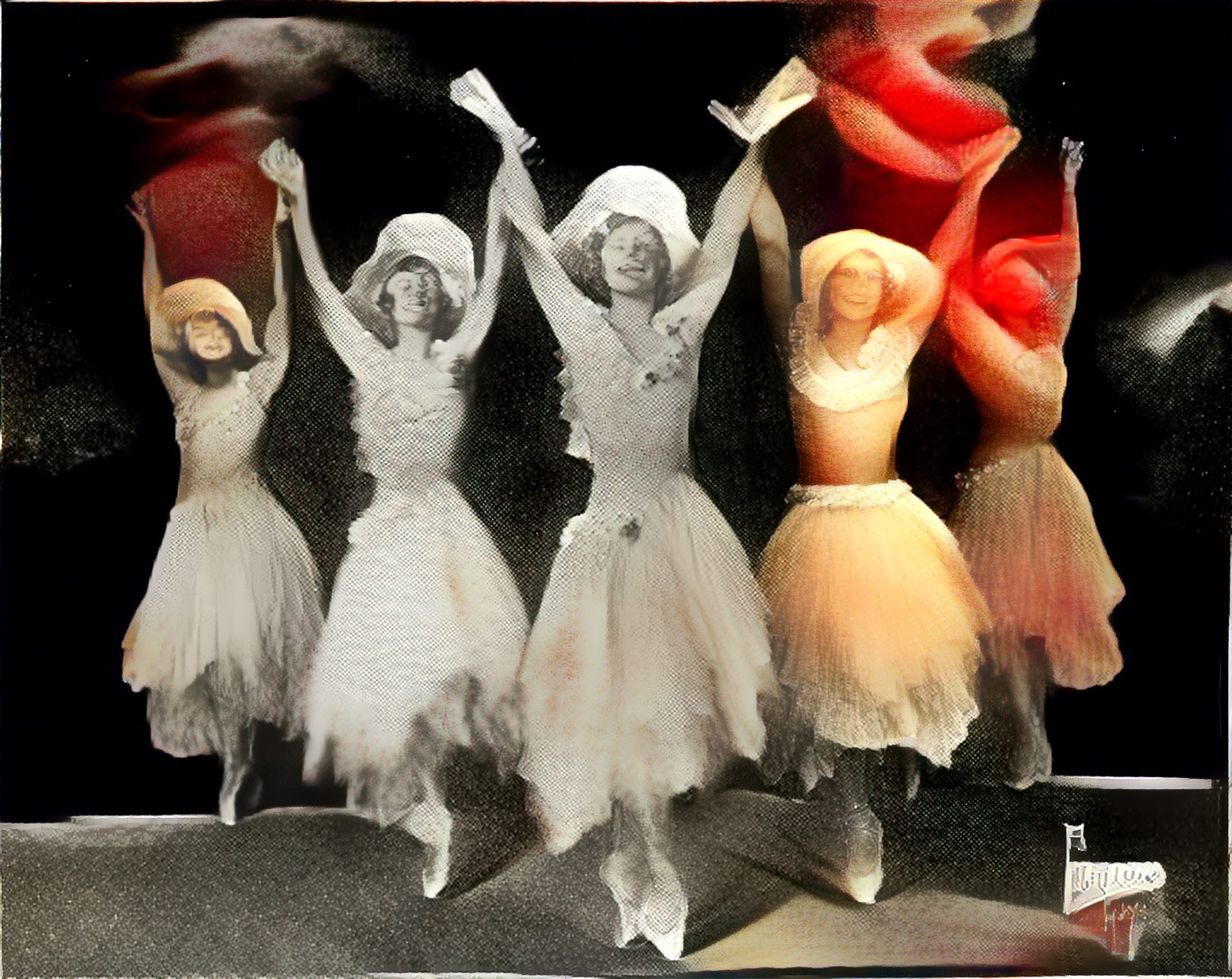
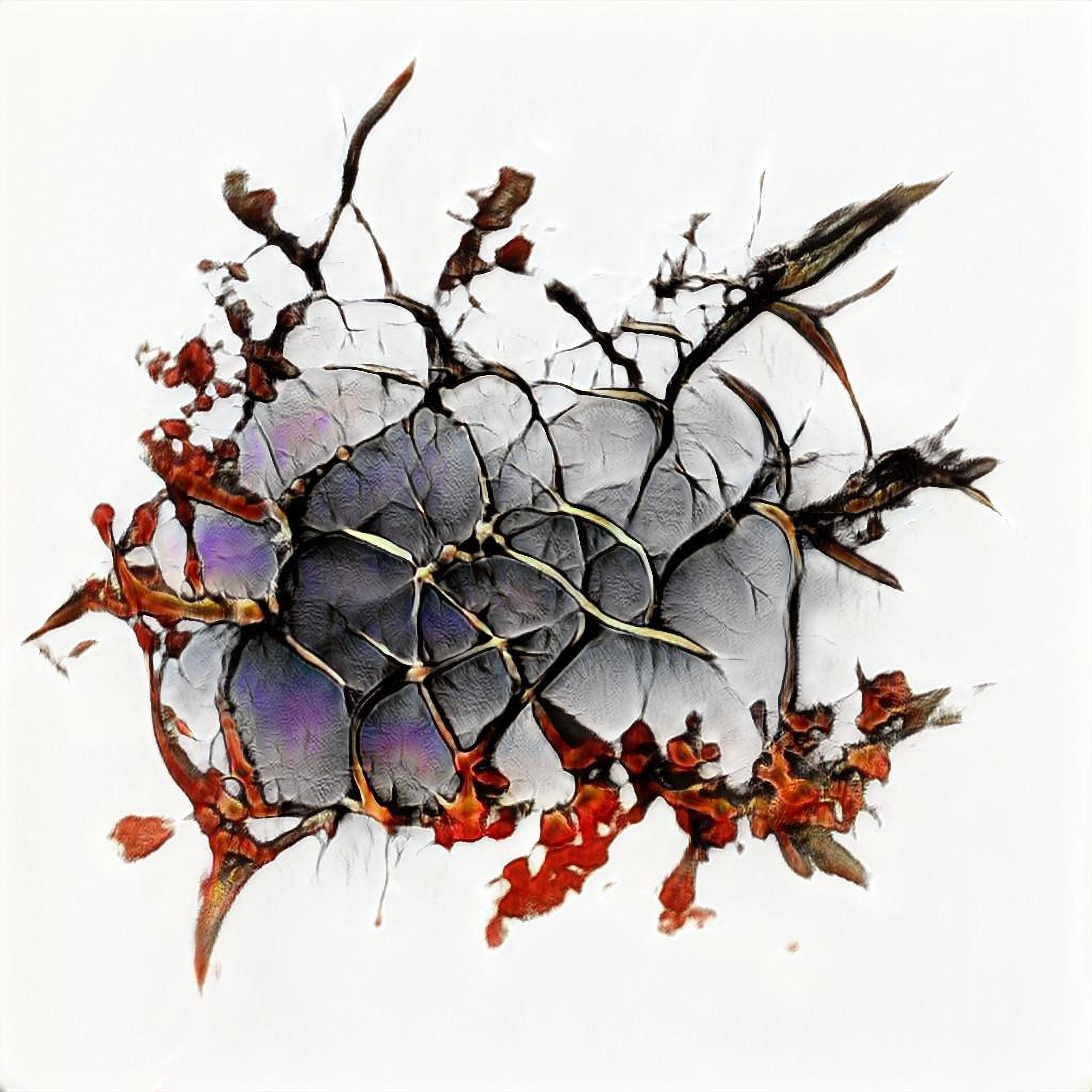
Uroboros Festival!
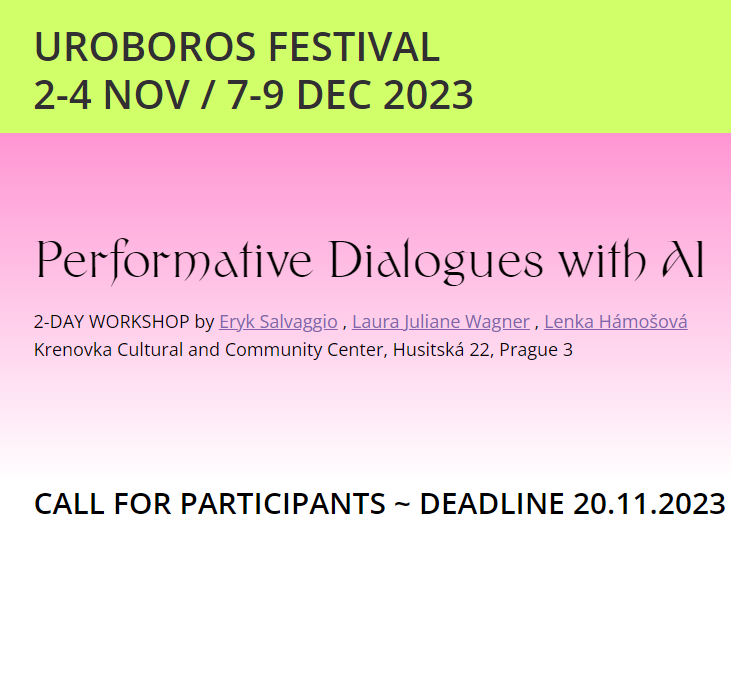
I will be at the Uroboros festival in Prague running a workshop for “Models for Making Distance” and talking about algorithmic resistance. It’s a small space and registrations are, I think, closed. But if you’re in Prague and want to connect at the festival, I will be in the city for the duration and then some, eating as much smažený sýr as possible. (Maybe you can still register, I don’t know).